熊猫数据集_大熊猫数据框的5个基本操作
熊猫数据集
Tips and Tricks for Data Science
数据科学技巧与窍门
Pandas is a powerful and easy-to-use software library written in the Python programming language, and is used for data manipulation and analysis.
Pandas是使用Python编程语言编写的功能强大且易于使用的软件库,可用于数据处理和分析。
Installing pandas: https://pypi.org/project/pandas/
安装熊猫: https : //pypi.org/project/pandas/
pip install pandas
pip install pandas
什么是Pandas DataFrame? (What is a Pandas DataFrame?)
A pandas DataFrame is a two dimensional data structure which stores data in a tabular form. Every row and column are labeled and can hold data of any type.
pandas DataFrame是二维数据结构,以表格形式存储数据。 每行和每列都有标签,可以保存任何类型的数据。
Here is an example:
这是一个例子:

1.创建一个熊猫DataFrame (1. Creating a pandas DataFrame)
The pandas.DataFrame constructor:
pandas.DataFrame构造函数:
pandas.DataFrame(data=None, index=None, columns=None, dtype=None, copy=False
pandas.DataFrame(data=None, index=None, columns=None, dtype=None, copy=False
data This parameter serves as the input to make a DataFrame, which could be a NumPy ndarray, iterable, dict or another DataFrame. An ndarray is a multidimensional container of items of the same type and size. An iterable is any Python object capable of returning its members one at a time, permitting to be iterated over in a for-loop. Some examples for iterables are lists, tuples and sets. Dict here can refer to pandas Series, arrays, constants or list-like objects.
data此参数用作制作DataFrame的输入,该DataFrame可以是NumPy ndarray,可迭代,dict或另一个DataFrame 。 ndarray是具有相同类型和大小的项目的多维容器。 可迭代对象是能够一次返回其成员并允许在for循环中对其进行迭代的任何Python对象。 可迭代的一些示例是列表,元组和集合。 这里的Dict可以引用pandas系列,数组,常量或类似列表的对象。
indexThis parameter could have an Index or an array-like data type and serves as the index for the row labels in the resulting DataFrame. If no indexing information is provided, this parameter will default to RangeIndex.
index此参数可以具有Index或类似数组的数据类型,并用作结果DataFrame中行标签的索引。 如果没有提供索引信息,则此参数将默认为RangeIndex 。
columnsThis parameter could have an Index or an array-like data type and serves as the index for the column labels in the resulting DataFrame. If no indexing information is provided, this parameter will default to RangeIndex.
columns此参数可以具有Index或类似数组的数据类型,并用作结果DataFrame中列标签的索引。 如果没有提供索引信息,则此参数将默认为RangeIndex 。
dtypeEach column in the DataFrame can only have a single data type. This parameter is used to force a certain data type. By default, datatype is inferred from data.
DTYPE在数据帧的每一列只能有一种数据类型。 此参数用于强制某种数据类型。 默认情况下,从数据推断出数据类型。
copyWhen this parameter is set to True, and the input data is a DataFrame or a 2D ndarray, data is copied into the resulting DataFrame. By default, copy is set to False.
复制如果将此参数设置为True,并且输入数据是DataFrame或2D ndarray,则将数据复制到结果DataFrame中。 默认情况下,复制设置为False。
从Python字典创建Pandas DataFrame (Creating a Pandas DataFrame from a Python Dictionary)
import pandas as pd
import pandas as pd
d = {'Name' : ['John', 'Adam', 'Jane'], 'Age' : [25, 18, 30]}pd.DataFrame(d)
d = {'Name' : ['John', 'Adam', 'Jane'], 'Age' : [25, 18, 30]}pd.DataFrame(d)

The index parameter can be used to change the default row index and the columns parameter can be used to change the order of the keys:
index参数可用于更改默认行索引, columns参数可用于更改键的顺序:
d = {'Name' : ['John', 'Adam', 'Jane'], 'Age' : [25, 18, 30]}pd.DataFrame(d, index=[10, 20, 30], columns=['First Name', 'Current Age'])
d = {'Name' : ['John', 'Adam', 'Jane'], 'Age' : [25, 18, 30]}pd.DataFrame(d, index=[10, 20, 30], columns=['First Name', 'Current Age'])

从列表创建Pandas DataFrame: (Creating a Pandas DataFrame from a list:)
l = [['John', 25], ['Adam', 18], ['Jane', 30]]pd.DataFrame(l, columns=['Name', 'Age'])
l = [['John', 25], ['Adam', 18], ['Jane', 30]]pd.DataFrame(l, columns=['Name', 'Age'])

从文件创建Pandas DataFrame (Creating a Pandas DataFrame from a File)
For any Data Science process, the dataset is commonly stored in files having formats like CSV (Comma Separated Values). Pandas allows storing data along with their labels from a CSV file using the method pandas.read_csv().
对于任何数据科学过程,数据集通常存储在具有CSV(逗号分隔值)之类的格式的文件中。 Pandas允许使用pandas.read_csv()方法将数据及其标签中的数据与CSV文件一起存储。


2.从Pandas DataFrame中选择行和列 (2. Selecting Rows and Columns from a Pandas DataFrame)
从Pandas DataFrame中选择列 (Selecting Columns from a Pandas DataFrame)
Columns can be selected using their column names.
可以使用列名称选择列。
df[column_1, column_2])
df[ column_1 , column_2 ])

从Pandas DataFrame中选择行 (Selecting Rows from a Pandas DataFrame)
Pandas provides 2 attributes for selecting rows from a DataFrame: loc
and iloc
Pandas提供了2个用于从DataFrame中选择行的属性: loc
和iloc
loc
is label-based, which means that the row label has to be specified and iloc
is integer-based which means that the integer index has to be specified.
loc
是基于标签的,这意味着必须指定行标签,而iloc
是基于整数的,这意味着必须指定整数索引。

3.在Pandas DataFrame中插入行和列 (3. Inserting Rows and Columns to a Pandas DataFrame)
在Pandas DataFrame中插入行 (Inserting Rows to a Pandas DataFrame)
One method of inserting a row into a DataFrame is to create a pandas.Series()
object and insert it at the end of the DataFrame using the pandas.DataFrame.append()
method. The column indices of the DataFrame serve as the index attribute for the Series object.
将行插入DataFrame的一种方法是创建pandas.Series()
对象,然后使用pandas.DataFrame.append()
方法将其插入DataFrame的pandas.DataFrame.append()
。 DataFrame的列索引用作Series对象的索引属性。

将列插入Pandas DataFrame (Inserting Columns to a Pandas DataFrame)
One easy method of adding a column to a DataFrame is by just referring to the new column and assigning values.
将列添加到DataFrame的一种简单方法是仅引用新列并分配值。

4.从Pandas DataFrame删除行和列 (4. Deleting Rows and Columns from a Pandas DataFrame)
从Pandas DataFrame删除行 (Deleting Rows from a Pandas DataFrame)
A row can be deleted using the method pandas.DataFrame.drop() with it’s row label.
可以使用带有行标签的pandas.DataFrame.drop()方法删除一行。

To delete a row based on a column, the index of the row is obtained using the DataFrame.index attribute and then the row with the index is deleted using the pandas.DataFrame.drop() method.
要删除基于列的行,请使用DataFrame.index属性获取该行的索引,然后使用pandas.DataFrame.drop()方法删除具有索引的行。

从Pandas DataFrame删除列 (Deleting Columns from a Pandas DataFrame)
A column can be deleted from a DataFrame based on its label as well as its position in the DataFrame using the method pandas.DataFrame.drop().
可以使用pandas.DataFrame.drop()方法根据列的标签及其在DataFrame中的位置从DataFrame中删除列。

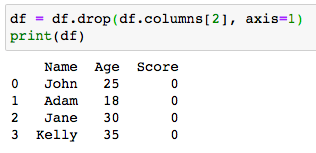
The axis
argument is set to 1 when dropping columns, and 0 when dropping rows.
删除列时, axis
参数设置为1;删除行时, axis
参数设置为0。
5.对Pandas DataFrame排序 (5. Sorting a Pandas DataFrame)
A Pandas DataFrame can be sorted using the pandas.DataFrame.sort_values()
method. The by parameter for the method serves as the label of the column to sort by and ascending is set to True for sorting in ascending order and to False for sorting in descending order.
可以使用pandas.DataFrame.sort_values()
方法对Pandas DataFrame进行排序。 该方法的by参数用作要按其进行排序的列的标签,并且升序设置为True(以升序排序),设置为False(以降序排序)。


https://www.datacamp.com/community/tutorials/pandas-tutorial-dataframe-pythonhttps://realpython.com/pandas-dataframe/#creating-a-pandas-dataframehttps://www.tutorialspoint.com/python_pandas/python_pandas_dataframe.htmhttps://pandas.pydata.org/pandas-docs/stable/reference/api/pandas.DataFrame.html
https://www.datacamp.com/community/tutorials/pandas-tutorial-dataframe-python https://realpython.com/pandas-dataframe/#creating-a-pandas-dataframe https://www.tutorialspoint.com/python_pandas/python_pandas_dataframe.htm https://pandas.pydata.org/pandas-docs/stable/reference/api/pandas.DataFrame.html
翻译自: https://medium.com/ml-course-microsoft-udacity/5-fundamental-operations-on-a-pandas-dataframe-93b4384dff9d
熊猫数据集
http://www.taodudu.cc/news/show-995304.html
相关文章:
- 帮助学生改善学习方法_学生应该如何花费时间改善自己的幸福
- 熊猫数据集_对熊猫数据框使用逻辑比较
- 决策树之前要不要处理缺失值_不要使用这样的决策树
- gl3520 gl3510_带有gl gl本机的跨平台地理空间可视化
- 数据库逻辑删除的sql语句_通过数据库的眼睛查询sql的逻辑流程
- 数据挖掘流程_数据流挖掘
- 域嵌套太深_pyspark如何修改嵌套结构域
- spark的流失计算模型_使用spark对sparkify的流失预测
- Jupyter Notebook的15个技巧和窍门,可简化您的编码体验
- bi数据分析师_BI工程师和数据分析师的5个格式塔原则
- 因果推论第六章
- 熊猫数据集_处理熊猫数据框中的列表值
- 数据预处理 泰坦尼克号_了解泰坦尼克号数据集的数据预处理
- vc6.0 绘制散点图_vc有关散点图的一切
- 事件映射 消息映射_映射幻影收费站
- 匿名内部类和匿名类_匿名schanonymous
- ab实验置信度_为什么您的Ab测试需要置信区间
- 支撑阻力指标_使用k表示聚类以创建支撑和阻力
- 均线交易策略的回测 r_使用r创建交易策略并进行回测
- 初创公司怎么做销售数据分析_初创公司与Faang公司的数据科学
- 机器学习股票_使用概率机器学习来改善您的股票交易
- r psm倾向性匹配_南瓜香料指标psm如何规划季节性广告
- 使用机器学习预测天气_如何使用机器学习预测着陆
- 数据多重共线性_多重共线性对您的数据科学项目的影响比您所知道的要多
- 充分利用昂贵的分析
- 如何识别媒体偏见_描述性语言理解,以识别文本中的潜在偏见
- 数据不平衡处理_如何处理多类不平衡数据说不可以
- 糖药病数据集分类_使用optuna和mlflow进行心脏病分类器调整
- mongdb 群集_群集文档的文本摘要
- gdal进行遥感影像读写_如何使用遥感影像进行矿物勘探
熊猫数据集_大熊猫数据框的5个基本操作相关推荐
- 熊猫数据集_为数据科学拆箱熊猫
熊猫数据集 If you are already familiar with NumPy, Pandas is just a package build on top of it. Pandas pr ...
- 熊猫数据集_对熊猫数据框使用逻辑比较
熊猫数据集 P (tPYTHON) Logical comparisons are used everywhere. 逻辑比较随处可见 . The Pandas library gives you a ...
- 熊猫数据集_熊猫迈向数据科学的第一步
熊猫数据集 I started learning Data Science like everyone else by creating my first model using some machi ...
- 熊猫数据集_处理熊猫数据框中的列表值
熊猫数据集 Have you ever dealt with a dataset that required you to work with list values? If so, you will ...
- 熊猫数据集_在日常生活中使用熊猫数据框
熊猫数据集 Before getting started let us do a quick revision, Pandas is a python library that gives elite ...
- 熊猫数据集_用熊猫掌握数据聚合
熊猫数据集 Data aggregation is the process of gathering data and expressing it in a summary form. This ty ...
- 熊猫数据集_使用大数据的熊猫
熊猫数据集 减少多达90%的内存使用量的提示 (Tips for reducing memory usage by up to 90%) When working using pandas with ...
- 熊猫数据集_熊猫迈向数据科学的第三部分
熊猫数据集 Data is almost never perfect. Data Scientist spend more time in preprocessing dataset than in ...
- 熊猫数据集_熊猫迈向数据科学的第二部分
熊猫数据集 If you haven't read the first article then it is advised that you go through that before conti ...
最新文章
- 《经济学人》万字长文:DeepMind和谷歌的AI拉锯战
- java B2B2C 源码 Springcloud多租户电子商城系统- Stream重新入队(RabbitMQ)
- runtime objc_msgSend
- [css] css的linear-gradient有什么作用呢?
- c语言 数组、字符串的形参格式_华中师范大学计算机考研874C语言笔记(一)
- dataframe数据标准化处理_数据处理中的标准化、归一化究竟是什么?
- 【LeetCode】【HOT】19. 删除链表的倒数第 N 个结点(双指针)
- java 测试磁盘io,详解三种Linux测试磁盘IO性能的方法总结,值得收藏
- 可多语句执行下不用单引号outfile写shell
- opta球员大数据预测胜负_大数据预测4个特征,10个典型行业
- 力扣LCP3机器人大冒险
- 【SpringBoot】SpringBoot的banner制作
- 5大输入法突然下架!得知真相的网友懵了:我都用了10年了……
- vue+element中多选框选一个然而就全部选中了
- mysql dede arctiny_dede标签的使用
- 瑞士轮赛制模拟器_【入门必读】VGC综合介绍(下篇)【翻译】
- Arduino实时时钟设计(TM1637数码管显示)
- Hive查询报错,return code 1 from org.apache.hadoop.hive.ql.exec.mr.MapRedTask
- Nature给学术界立规矩:ChatGPT等大模型不可以成为作者
- php内容怎么设置隐藏,在PHP中设置隐藏元素的值 - php