mad离群值_全部关于离群值
mad离群值
An outlier is a data point in a data set that is distant from all other observations. A data point that lies outside the overall distribution of the dataset. Or in a layman term, we can say, an outlier is something that behaves differently from the combination/collection of the data.
离群值是数据集中的一个数据点,该数据点与所有其他观察值相距较远。 位于数据集总体分布之外的数据点。 或用外行术语来说,离群值是与数据的组合/收集不同的行为。
Outliers can be very informative about the subject-area and data collection process. It’s essential to understand how outliers occur and whether they might happen again as a normal part of the process or study area. To understand outliers, we need to go through these points:
异常值可能对主题区域和数据收集过程很有帮助。 了解离群值如何发生以及在过程或研究区域的正常部分是否会再次发生离群值至关重要。 要了解异常值,我们需要经历以下几点:
- what causes the outliers?是什么导致异常值?
- Impact of the outlier离群值的影响
- Methods to Identify outliers识别异常值的方法
是什么导致异常值? (What causes the outliers?)
Before dealing with the outliers, one should know what causes them. There are three causes for outliers — data entry/An experiment measurement errors, sampling problems, and natural variation.
在处理异常值之前,应该知道是什么导致了异常值。 造成异常的原因有三点:数据输入/实验测量错误,采样问题和自然变化。
- Data entry /An experimental measurement error数据输入/实验测量错误
An error can occur while experimenting/entering data. During data entry, a typo can type the wrong value by mistake. Let us consider a dataset of age, where we found a person age is 356, which is impossible. So this is a Data entry error.
实验/输入数据时可能会发生错误。 在数据输入期间,错字可能会错误输入错误的值。 让我们考虑年龄的数据集,我们发现一个人的年龄是356,这是不可能的。 因此,这是一个数据输入错误。

These types of errors are easy to identify. If you determine that an outlier value is an error, we can fix this error by deleting the data point because you know it’s an incorrect value.
这些类型的错误很容易识别。 如果您确定异常值是错误的,我们可以通过删除数据点来解决此错误,因为您知道该值是错误的。
2. Sampling problems
2.抽样问题
Outliers can occur while collecting random samples. Let us consider an example where we have records of bone density of various subjects, but there is an unusual growth of bone in a subject, after analyzing this has been discovered that the subject had diabetes, which affects bone health. The goal was to model bone density growth in girls with no health conditions that affect bone growth. Since the data is not a part of the target population so we will not consider this.
收集随机样本时可能会出现异常值。 让我们考虑一个示例,在该示例中,我们记录了各个受试者的骨密度,但是在分析了该受试者患有糖尿病并影响骨骼健康之后,该受试者的骨骼异常生长。 目的是在没有影响骨骼生长的健康状况的女孩中模拟骨骼密度的增长。 由于数据不是目标人群的一部分,因此我们不会考虑这一点。
3. Natural variation
3.自然变异
Suppose we need to check the reliability of a machine. The normal process includes standard materials, manufacturing settings, and conditions. If something unusual happens during a portion of the study, such as a power failure or a machine setting drifting off the standard value, it can affect the products. These abnormal manufacturing conditions can cause outliers by creating products with atypical strength values. Products manufactured under these unusual conditions do not reflect your target population of products from the normal process. Consequently, you can legitimately remove these data points from your dataset.
假设我们需要检查机器的可靠性。 正常过程包括标准材料,制造设置和条件。 如果在研究的一部分过程中发生异常情况,例如电源故障或机器设置偏离标准值,则可能会影响产品。 这些异常的制造条件可能会通过创建具有非典型强度值的产品而导致异常值。 在这些异常条件下制造的产品不能反映正常过程中目标产品的数量。 因此,您可以合法地从数据集中删除这些数据点。
离群值的影响 (Impact of the outlier)
Outliers can change the results of the data analysis and statistical modeling. Following are some impacts of outliers in the data set:
离群值可以更改数据分析和统计建模的结果。 以下是数据集中异常值的一些影响:
- It may cause a significant impact on the mean and the standard deviation可能会对平均值和标准偏差产生重大影响
- If the outliers are non-randomly distributed, they can decrease normality如果离群值是非随机分布的,则它们可能会降低正态性
- They can bias or influence estimates that may be of substantive interest它们可能会偏向或影响可能具有实质意义的估计
- They can also impact the basic assumption of Regression, ANOVA, and other statistical model assumptions.它们还会影响回归,ANOVA和其他统计模型假设的基本假设。
To understand the impact deeply, let’s take an example to check what happens to a data set with and without outliers in the data set.
为了深入了解其影响,让我们举一个例子来检查在数据集中有无异常时数据集会发生什么情况。
Let’s examine what can happen to a data set with outliers. For the sample data set:
让我们研究带有异常值的数据集会发生什么。 对于样本数据集:
1, 1, 2, 2, 2, 2, 3, 3, 3, 4, 4
1,1,2,2,2,2,3,3,3,4,4
We find the following mean, median, mode, and standard deviation:
我们发现以下平均值,中位数,众数和标准差:
Mean = 2.58
均值= 2.58
Median = 2.5
中位数= 2.5
Mode = 2
模式= 2
Standard Deviation = 1.08
标准偏差= 1.08
If we add an outlier to the data set:
如果我们向数据集添加离群值:
1, 1, 2, 2, 2, 2, 3, 3, 3, 4, 4, 400
1,1,2,2,2,2,3,3,3,4,4,400
The new values of our statistics are:
我们的统计信息的新值是:
Mean = 35.38
均值= 35.38
Median = 2.5
中位数= 2.5
Mode = 2
模式= 2
Standard Deviation = 114.74
标准偏差= 114.74
As you can see, having outliers often has a significant effect on your mean and standard deviation.
如您所见,离群值通常会对平均值和标准差产生重大影响。
识别异常值的方法 (Methods to Identify outliers)
There are various ways to identify outliers in a dataset, following are some of them:
在数据集中识别异常值的方法有多种,以下是其中的一些方法:
- Sorting the data排序数据
- Using graphical Method使用图形方法
- Using z score使用z分数
- Using the IQR interquartile range使用IQR四分位距
排序数据 (Sorting the data)
Sorting the dataset is the simplest and effective method to check unusual value. Let us consider an example of age dataset:
对数据集进行排序是检查异常值的最简单有效的方法。 让我们考虑年龄数据集的示例:
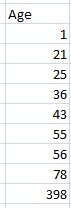
In the above dataset, we have sort the age dataset and get to know that 398 is an outlier. Sorting data method is most effective on the small dataset.
在上面的数据集中,我们对年龄数据集进行了排序,并且知道398是一个离群值。 排序数据方法对小型数据集最有效。
使用图形方法 (Using graphical Method)
We can detect outliers with the help of graphical representation like Scatter plot and Boxplot.
我们可以借助散点图和Boxplot等图形表示来检测异常值。

1. Scatter Plot
1.散点图
Scatter plots often have a pattern. We call a data point an outlier if it doesn’t fit the pattern. Here we have a scatter plot of Weight vs height. Notice how two of the points don’t fit the pattern very well. There is no special rule that tells us whether or not a point is an outlier in a scatter plot. When doing more advanced statistics, it may become helpful to invent a precise definition of “outlier”.
散点图通常具有某种模式。 如果数据点不适合模式,我们称其为离群值 。 这是重量与高度的散点图。 请注意,有两个点不太适合该模式。 没有特殊的规则可以告诉我们点在散点图中是否是异常值。 在进行更高级的统计时,发明精确定义“异常值”可能会有所帮助。

2. Box-Plot
2.箱线图
Box-plot is one of the most effective ways of identifying Outliers in a dataset. When reviewing a box plot, an outlier is defined as a data point that is located outside the box of the box plot. As seen in the box plot of bill vs days. Box-Plot uses the Interquartile range(IQR) to detect outliers.
箱线图是识别数据集中异常值的最有效方法之一。 查看箱形图时,离群值定义为位于箱形图框外部的数据点。 如票据与天数的方框图所示。 箱线图使用四分位间距(IQR)来检测离群值。
使用z分数 (Using z-score)

Z-score (also called a standard score) gives you an idea of how many standard deviations away a data point is from the mean.. But more technically it’s a measure of how many standard deviations below or above the population mean a raw score is.
Z分数(也称为标准分数 )使您了解一个数据点与平均值之间有多少标准偏差。但是从技术上讲,它衡量的是低于或高于总体的多少标准偏差表示原始分数是多少。 。
Z score = (x -mean) / std. deviation
Z分数=(x-均值)/ std。 偏差
In a normal distribution, it is estimated that
在正态分布中,估计
68% of the data points lie between +/- 1 standard deviation.
68%的数据点位于+/- 1标准偏差之间。
95% of the data points lie between +/- 2 standard deviation.
95%的数据点在+/- 2标准偏差之间。
99.7% of the data points lie between +/- 3 standard deviation.
99.7%的数据点位于+/- 3标准偏差之间。
Formula for Z score = (Observation — Mean)/Standard Deviation
Z分数的公式=(观测值–平均值)/标准差
z = (X — μ) / σ
z =(X —μ)/σ
Let us consider a dataset:
让我们考虑一个数据集:


使用IQR四分位距 (Using the IQR interquartile range)

Interquartile range(IQR), is just the width of the box in the box-plot which can be used as a measure of how spread out the values are. An outlier is any value that lies more than one and a half times the length of the box from either end of the box.
四分位数间距(IQR)只是箱形图中箱形的宽度,可用作度量值分布的程度。 离群值是从框的两端到框长度的一半以上的任何值。
Steps
脚步
- Arrange the data in increasing order按升序排列数据
- Calculate first(q1) and third quartile(q3)计算第一个(q1)和第三个四分位数(q3)
- Find interquartile range (q3-q1)查找四分位数范围(q3-q1)
- Find lower bound q1*1.5求下界q1 * 1.5
- Find upper bound q3*1.5求上限q3 * 1.5
Anything that lies outside of lower and upper bound is an outlier
上下限以外的任何东西都是异常值
Let us take the same example as of Z-score:
让我们以与Z分数相同的示例为例:


As you can see we have found Lower and upper values that is: 7.5 and 19.5, so anything that lies outside these values is an outlier.
如您所见,我们发现下限值和上限值分别是:7.5和19.5,因此超出这些值的任何值都是异常值。
This is all we have about outliers, I hope you enjoyed reading. Thank you
这就是关于异常值的全部内容,希望您喜欢阅读。 谢谢
翻译自: https://medium.com/analytics-vidhya/its-all-about-outliers-cbe172aa1309
mad离群值
http://www.taodudu.cc/news/show-2898556.html
相关文章:
- 离群值
- 思维导图ubuntu_XMind for Linux - 思维导图 | linux软件
- win32分割窗口的一个简易做法,非常好的编程思维(一)
- 什么是GO+和GO哪里不一样
- 关于Win10与Ubuntu18.04的装机教程与疑问解答
- 强推win10损害用户利益?这锅微软不背
- win2003 由于可用空间计算失败_幼儿编程启蒙怎么做?智能家居+ai 玩空间是最佳教具...
- xman的思维导图快捷键_macz技巧分享:思维导图XMind快捷键汇总
- 剖析ArrayList / 计算机程序的思维逻辑
- 分布式系统之道:Lamport 逻辑时钟
- nag在逆向中是什么意思_CrackMe003-如何理解透VB逆向中的4C大法(图文+视频)-重在思维方法...
- python是高级语言还是机器语言_02_python是一种什么语言?
- win10系统CMD窗口MySQL5.6中文乱码问题
- 分分钟教你如何在win10中运行第一个C语言程序
- 在win10查看本机linux的文件,Windows 10变身开发者利器:内置Linux内核,轻松查看Linux子系统文件...
- 第一章 c语言概述程序逻辑,第一章 程序逻辑与C语言概述
- 程序员如何提高自己的逻辑思维和技术水平
- 软件项目“免坑”指南
- 最新论文笔记(+16):K-Time Modifiable and Epoch-Based Redactable Blockchain / TIFS 2021
- 你在职场可能犯下的最大错误
- 14-《ARKit by Tutorials》读书笔记1:开始入门
- OSX + iTerm2 + vim + ssh + CentOS/本机配色原理小分析
- 《失控》后半部
- Uber是什么 读书笔记
- 每天读一点职场心理学读书笔记
- 能力陷阱读书笔记
- 读书笔记《能力陷阱》第四章:试着朝更多不同的方向发展自己
- 腾讯产品法之读书笔记
- 《滴滴重MVVM框架Chameleon》架构篇读后感
- (超级详细秒懂)带你秒懂web电子账单板块代码
mad离群值_全部关于离群值相关推荐
- 图像离群值_什么是离群值?
图像离群值 你是! (You are!) Actually not. This is not a text about you. 其实并不是. 这不是关于您的文字. But, as Gladwell ...
- matlab离群值算法_什么是离群值如何检测和删除它们对离群值敏感的算法
matlab离群值算法 In statistics, an outlier is an observation point that is distant from other observation ...
- python 离群值_数据预处理初学者宝典:360° 掌握离群值识别
全文共6023字,预计学习时长20分钟或更长 来源:Pexels 离群值监测和处理是数据预处理中最重要的环节之一.机器学习算法注重数据点的范围和分布,而数据离群值掩盖训练进程,导致训练时间加长.模型准 ...
- 直线回归数据 离群值_处理离群值:OLS与稳健回归
直线回归数据 离群值 When it comes to regression analysis - outliers (or values that are well outside of the m ...
- 离群值如何处理_有理处理离群值的局限性
离群值如何处理 ARIMA models can be quite adept when it comes to modelling the overall trend of a series alo ...
- python 离群值_如何从Numpy数组中删除离群值
我写了一个代码,取多个图像的平均值来检索背景,这基本上删除了图像中的移动对象.我试着在取平均值之前去掉离群值,这样我就可以得到背景而不是褪色的对象.我尝试了一些技巧,最近的一个是:#!/usr/bin ...
- spotify 数据分析_我的Spotify流历史分析
spotify 数据分析 Spotisis /spo-ti-sis/ noun The analysis of one's Spotify streaming history using Python ...
- 米其林餐厅 盐之花_在世界范围内探索《米其林指南》
米其林餐厅 盐之花 Among the culinary world, there are few greater accolades for a restaurant than being awar ...
- 朴素贝叶斯分类器 文本分类_构建灾难响应的文本分类器
朴素贝叶斯分类器 文本分类 背景 (Background) Following a disaster, typically you will get millions and millions of ...
最新文章
- yii权限rbac验证三张表字段说明
- 软件分发、补丁推送排错
- 微型计算机滑动平均值滤波器方程,微型计算机控制技术期末复习2011.ppt
- Appium下载安装及环境配置
- java 十进制转十六进制、十进制转二进制、二进制转十进制、二进制转十六进制...
- oracle 数据库中执行数据库语句能找到数据,但是程序中却抓取不到
- jQuery缓存数据——仿Map
- 浅谈Java回调机制
- springcloud- FeginClient 调用统一拦截添加请求头 RequestInterceptor ,被调用服务获取请求头...
- 基于CentOS7配置ArcGIS enterprise
- C语言实现汉诺塔【图文讲解】
- 百度文库免下载券下载的方法
- 计算机基础17秋在线作业3,西南大学17秋1056《计算机基础》在线作业(参考资料)...
- SpringBoot+Mybatis-plus的员工打卡系统
- android studio git 错误 unable to read askpass
- wx小程序笔记(2)
- 获取网易云音乐封面图片
- jtag、在线仿真器
- 2018校招笔试题——网易编程题跳石板
- 三个等号和两个等号的区别(“===”和“==”)