h5py group_人工智能驱动的零售:H&M Group如何做到
h5py group
This article was originally published on Hyperight Read.
本文最初发表于 超读 。
Fashion retailers are increasingly turning to artificial intelligence (AI) for help in the quest to give customers what they want. AI-driven retail enables brands to compete in the economy of the 21st century and meet modern customer demands by personalizing the shopping experience.
时尚零售商越来越多地向人工智能(AI)寻求帮助,以期向顾客提供他们想要的东西。 人工智能驱动的零售使品牌能够在21世纪的经济中竞争,并通过个性化购物体验来满足现代客户的需求。
As more retail companies are taking their business from the traditional brick and mortar retail stores into e-commerce, they are able to get more insight into their customers’ preferences to serve the demand.
随着越来越多的零售公司将其业务从传统的实体零售商店转变为电子商务,他们能够对客户的喜好有更深入的了解,从而满足需求。
Talking about AI in retail brings us to one of the most beloved brands in the fashion industry — H&M.
谈论零售业中的AI,将我们带入了时尚界最受欢迎的品牌之一-H&M。
H&M Group及其AI驱动的故事 (H&M Group and their AI-driven story)
H&M Group has been heavily investing in artificial intelligence to stay on top of fashion cycles, and also to support its massive growth.
H&M Group一直在人工智能方面进行大量投资,以保持时尚周期的领先地位,并支持其大规模增长。
From their beginning in 1947, the fashion retailer followed rapid expansion across Europe, U.S. and globally. And as early as 1998 they began to sell online.
从1947年成立起,这家时装零售商就在欧洲,美国和全球范围内Swift扩张。 早在1998年,他们就开始在线销售商品。

Just in 2004, H&M held more than 1,000 stores, a number which grew exponentially to nearly 5,000 stores in 72 countries worldwide. And as of today, the retail giant employs 177,000 people and contributes to 1.6 million jobs for people employed by their suppliers.
仅在2004年,H&M就在全球72个国家/地区开设了1,000多家商店,而这一数字呈指数增长,达到近5,000家。 截至今天,这家零售业巨头拥有177,000名员工,并为供应商所雇用的人员创造了160万个就业机会。
But how does a brand manager sustain this kind of growth, reaching across different countries, markets and even continents? How do they make sure they meet the needs of such a huge base of customers with different shopping needs and expectations? And most importantly, how do they manage to run thousands of stores worldwide?
但是,品牌经理如何维持这种增长,并覆盖不同的国家,市场甚至大洲呢? 他们如何确保满足具有不同购物需求和期望的庞大客户群的需求? 最重要的是,他们如何在全球经营数千家商店?
The man who has answers for all these questions is Errol Koolmeister, H&M Group’s Product Area Lead Engineer AI Foundation at H&M Group. During his talk at the Data Innovation Summit 2019, he explained that they first started adopting AI into their business in 2016. The impact of digitalization was clearly visible and H&M Group knew they had to do something in order to stay relevant.“We, as a company, and as people, started going more and more online”, describes Errol.
对所有这些问题都有答案的人是H&M集团产品领域首席工程师AI Foundation AI基金会的Errol Koolmeister 。 在他的演讲中 数据创新峰会 他解释说,2019年,他们首先在2016年开始将AI应用于他们的业务。数字化的影响显而易见,H&M Group知道他们必须做点什么才能保持相关性。越来越多地上网”,Errol说道。

“We started to see that scaling a business model of physical stores became quite hard”, Errol says. The obvious solution was that they needed AI in order to sustain their growth.
“我们开始看到扩展实体店的业务模型变得相当困难”,Errol说。 显而易见的解决方案是他们需要AI才能维持自己的成长。
人工智能驱动的零售业的到来 (The advent of AI-driven retail)
Realizing they had to adjust to the new industrial shift, H&M Group asked themselves the question “Where do we want to be as a company?” That’s when the retail brand realised they needed to up their game in AI and advanced analytics. Although they excelled at some departments, such as CRM, they knew they didn’t have it at scale.
意识到他们必须适应新的产业转变,H&M Group问自己一个问题:“我们想成为一家公司吗?” 那时,零售品牌意识到他们需要在AI和高级分析中提升自己的游戏水平。 尽管他们在CRM等某些部门表现出色,但他们知道他们没有大规模的应用。
That was the moment when they started doing their first POC’s. “We tried to see if we could extract any value from them,” explains Errol. Luckily, the use cases that H&M Group picked were successful and could be put into production, making them profitable.
那是他们开始进行第一个POC的时刻。 “我们试图看看是否可以从中获取任何价值,” Errol解释道。 幸运的是,H&M Group挑选的用例很成功,可以投入生产,从而使它们盈利。
“It’s all about acting really rapidly,” he continues. “If we in 2016 only said let’s do a proof of concept and then stopped, no value would have been extracted”, Errol says. This is unfortunately what happens with 90% of other cases, they just do their POC and don’t continue with production.
他继续说道:“这一切都是关于Swift行动。” “如果我们在2016年只说让我们先做概念验证然后停下来,那将不会提取任何价值”,Errol说。 不幸的是,这是在其他90%的情况下发生的事情,他们只是进行POC而不会继续生产。
What proved to be successful for H&M Group, was that they were focused on production already in 2016. They were determined that if they were to start with AI, they would do it big. “It’s really about starting small, thinking big, and scaling fast”, highlights Errol. According to him, in reality, there are no AI use cases, they are all business cases. H&M Group actually uses AI to amplify their business solutions.
对于H&M Group而言,成功的原因是他们已于2016年开始专注于生产。他们确定,如果他们从AI开始,他们会做得更好。 “真正的出发点是从小处着手,大胆思考,然后Swift扩展”,Errol强调说。 据他介绍,实际上,没有AI用例,它们都是业务用例。 H&M Group实际上使用AI来扩大其业务解决方案。
H&M Group’s use cases have different maturity levels. But regardless of the case, they always focus on production. For this reason, they have a defined process for realizing use cases:
H&M Group的用例具有不同的成熟度级别。 但是无论如何,他们始终专注于生产。 因此,他们有一个定义的过程来实现用例:
- Proof of Concept (POC) — which doesn’t take long to realise because, as Errol explains, they already have their data lake in place, ready to go. This allows them to spin up virtual machines and get insights in a matter of a few weeks. If the POC is successful, it’s time for the next stage.概念验证(POC)-很快就可以实现,因为正如Errol解释的那样,他们已经准备好了自己的数据湖。 这使他们可以在几周内启动虚拟机并获得见解。 如果POC成功,则该进行下一个阶段了。
- Pilot — H&M Group pilots the solution in a few markets to test if the theory matches reality. They put the case into production to try it out and evaluate the results. If the pilot is successful, they roll out the solution.试点— H&M Group在一些市场试点该解决方案,以测试该理论是否符合实际。 他们将案件投入生产以进行试用并评估结果。 如果试点成功,他们将推出解决方案。
- Industrialization and roll-out — the last step of the process is to roll out the solution to all 72 countries, 177,000 employees and nearly 5,000 stores. Considering the number of users, the solutions have to be extremely bulletproof and reliable.工业化和推广-该过程的最后一步是将解决方案推广到所有72个国家/地区,177,000名员工和近5,000家商店。 考虑到用户数量,解决方案必须极其防弹且可靠。
H&M Group现实生活中AI驱动零售的例子 (Real-life H&M Group examples of AI-driven retail)
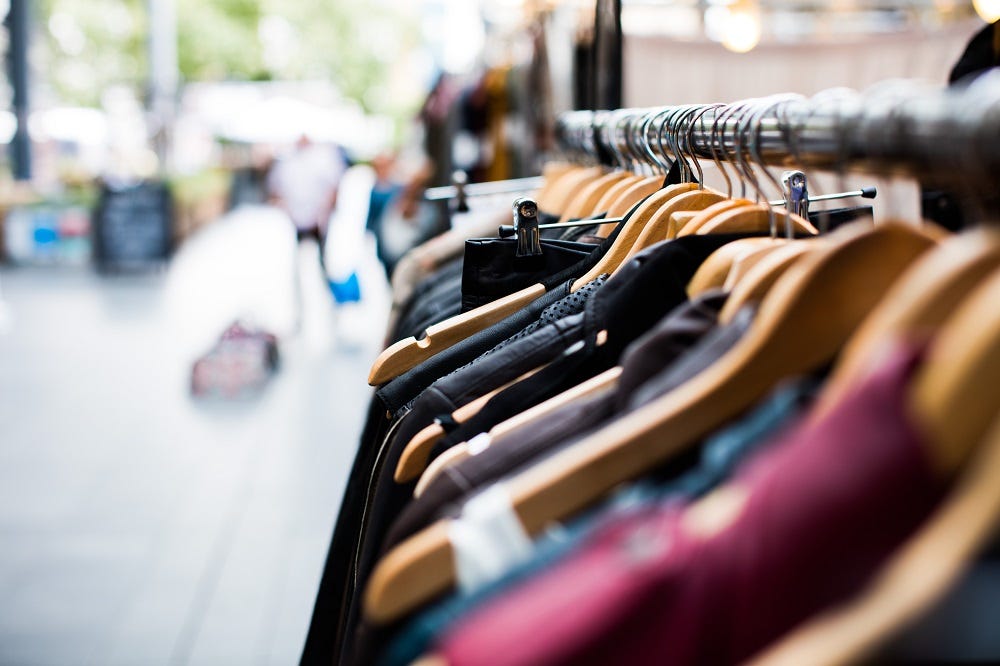
Here are some tangible examples thanks to implementing AI solutions.
由于实施了AI解决方案,这里有一些实际的例子。
Keeping popular items stocked — H&M relies on staying on top of trends in order to be successful. With the help of algorithms, they analyse store receipts and returns to evaluate purchases in each store. This way, the fashion brand knows which items to promote and stock more of in certain locations.
保持热门商品库存 -H&M依靠趋势保持领先才能成功。 借助算法,他们可以分析商店收据和退货,以评估每个商店的购买情况。 这样,时尚品牌便知道在某些位置要促销和库存更多商品。
Predicting market demand — Fashion retailers like H&M rely on fresh products at competitive prices. Data insights help H&M to predict what the market wants so they don’t have to discount their inventory to sell it out.
预测市场需求 -H&M等时尚零售商依赖具有竞争力的价格购买新鲜产品。 数据洞察力可以帮助H&M预测市场需求,因此他们不必打折存货就可以将其售出。
Automated warehouses — Today customers expect fast, hassle-free deliveries anytime and everywhere. Therefore, H&M Group has invested in automated warehouses that will ultimately offer next-day deliveries for the majority of the European markets. The warehouses and their free shipping, exclusive for loyal customers, are driven by algorithms and data.
自动化仓库 -如今,客户期望随时随地快速,轻松地交付货物。 因此,H&M集团投资了自动仓库,最终将为大多数欧洲市场提供第二天的交货。 忠诚客户专用的仓库及其免费送货受算法和数据驱动。
Personalised offline customer experience — H&M has introduced its personalised online recommendations also in their physical stores, with the help of RFID technology. Customers get in-store merchandise suggestions selected by algorithms. They can also see if an item they have seen online is available in a physical store, and scan labels to see if an item is available in another store or online.
个性化的离线客户体验 -H&M借助RFID技术也在其实体店中引入了其个性化的在线建议。 客户会获得由算法选择的店内商品建议。 他们还可以查看他们在网上看到的商品在实体商店中是否可用,并扫描标签以查看该商品是否在其他商店或在线中可用。
Tailor-made clothing — Partnering with an AI-technology platform, the Swedish fashion brand has tested on-demand production, which shows great potential to react more specifically to customer’s wishes, and to align product quantity to local demand.
量身定做的服装 —瑞典时尚品牌与AI技术平台合作 ,已按需生产测试,这显示出巨大的潜力,可以更具体地响应客户的需求,并根据当地需求调整产品数量。

H&M Group的故事有何收获? (What’s the takeaway from H&M Group’s story?)
In today’s digitalised world, it is impossible to manage a global retail brand such as H&M solely by humans. And where human capabilities end, AI begins. Taking H&M Group’s example of AI-driven retail, retailers can gain much from enhancing human intelligence by implementing AI into their business: more accurate merchandise decisions streamlined supply chain and top-level customer experience. This is what H&M Group calls Amplified Intelligence — the collaboration between machines and humans, science and art, data and gut feeling.
在当今的数字化世界中,不可能仅靠人类来管理诸如H&M之类的全球零售品牌。 在人类能力终结的地方,人工智能开始了。 以H&M Group的AI驱动零售为例,零售商可以通过在其业务中实施AI来增强人工智能,从而从中受益匪浅:更准确的商品决策可简化供应链和顶级客户体验。 这就是H&M Group所谓的增强智能-机器与人类,科学与艺术,数据和直觉之间的协作。
翻译自: https://towardsdatascience.com/ai-driven-retail-how-h-m-group-does-it-c9606597f7bc
h5py group
http://www.taodudu.cc/news/show-1874046.html
相关文章:
- openai-gpt_GPT-3的不道德故事:OpenAI的百万美元模型
- 通话时自动中断音乐播放_您知道用户在何处以及为何中断通话吗?
- 机器视觉科学计算可视化_模因视觉:对模因进行分类的科学
- 人工智能与自动驾驶汽车_自动驾驶汽车中的道德AI
- 是你渡过人生难关的助力_人工智能将助力安全返回工作场所。 这是如何做
- 机器学习 流式特征_Web服务与实时机器学习端点的流式传输
- 算法 博士_Strangecode博士-我如何学会不再担心并喜欢算法
- 妲己机器人功能_来自机器人影响者的5个功能强大的Instagram教训
- 创建dqn的深度神经网络_深度Q网络(DQN)-I
- dis 密集光流_密集光流估计的自监督注意力机制
- 意图识别 聊天机器人_如何解决聊天机器人中的意图冲突
- «构建并破坏它»:某些算法如何生成验证码,而另一些则如何破解
- 蒙特卡洛方法习题_蒙特卡洛方法
- 人体面部检测python_使用Python检测面部特征
- int是终结符还是非终结符_什么是自动化的终结?
- aba问题实际中有什么影响_实际影响是什么?
- 使用Pytorch的LSTM文本分类
- 创建dqn的深度神经网络_深度Q网络(DQN)-III
- 数字社会的下一波浪潮_下一波创业浪潮是自然而然的
- acl自然语言处理_ACL 2020中自然语言处理中的知识图
- gan生成印刷体字母_字母GAN:AI生成英语字母!
- 点击量作弊真的可以检测吗_作弊道德可以使自动驾驶汽车可行吗?
- 欺骗神经网络_可以欺骗神经网络吗?
- 图像增强python_Python图像增强简介(第1部分)
- jio tomcat_透过Jio Glass看
- 用于无人驾驶技术的车道线_自动驾驶汽车可用于查找车道的4种技术
- q-learning_教计算机如何通过Q-Learning玩Snake
- 深度强化学习从入门到大师_深度学习大师的经验教训
- 自然语言处理 入门_自然语言处理入门指南
- 变形金刚图纸_变形金刚救援
h5py group_人工智能驱动的零售:H&M Group如何做到相关推荐
- 6.26线下深度交流:数据如何驱动新零售升级?
[友盟+]数据训练营系列 数据训练营-如何驱动新零售体验升级 如题,本周二,清数大数据产业联盟将走进友盟+,与友盟+新零售业务总经理刘延明.市场战略总监史敏君,深度探讨新零售数据服务及最新应用实践. ...
- AI奇点将至 如何成为人工智能驱动型公司
数据智能产业创新服务媒体 --聚焦数智 · 改变商业 2023年4月16日,中泰证券首席经济学家李迅雷先生发表了<奇点将至:AI或开启新一轮科技革命>的文章.李迅雷先生认为,以智能化为特 ...
- AI算法不断突破 人工智能驱动创新需找对方向
https://www.toutiao.com/a6658047781480956429/ 近日,IBM 2019年Think大会上一场奇特的辩论赛展现在人们眼前,人类辩手在讲台上正进行着精彩陈述,一 ...
- AI+零售:人工智能撬动零售变革
来源:乐晴智库精选 人工智能的快速发展将有助于赋能新零售商,有效重构零售行业"人.货.场"等要素,提升各环节效率,最终提升消费者购物体验,推动零售行业迎来第五次变革. 近年来,在数 ...
- 全球及中国人工智能驱动的医学成像行业全景调研及运营战略分析报告2021-2027年版
全球及中国人工智能驱动的医学成像行业全景调研及运营战略分析报告2021-2027年版 目录 2020年,全球人工智能驱动的医学成像市场规模达到了 百万美元,预计2027年将达到 百万美元,年复合增长率 ...
- 【BDTC 2016】蚂蚁金服人工智能部技术总监李小龙:人工智能驱动金融生活
[CSDN现场报道]2016年12月8-10日,由中国计算机学会(CCF)主办,CCF大数据专家委员会承办,中国科学院计算技术研究所.中科天玑数据科技股份有限公司与CSDN共同协办,以"聚焦 ...
- 软件 3.0:人工智能驱动下的未来
[CSDN 编者按]软件 3.0 有望改变我们构建技术以及与技术交互的方式.这场革命的核心在于人工智能驱动的开发,以实现无缝的.类似人与人的软件交互. 原文链接:https://divgarg.sub ...
- 人工智能在新零售中的应用
什么是新零售? 新零售就是企业以互联网为依托,通过运用大数据.人工智能等先进技术手段,对商品的生产.流通与销售过程进行升级改造,对线上服务.线下体验以及现代物流进行深度融合的零售新模式. " ...
- 人工智能驱动的道路安全网络白皮书(英) (附下载)
点击文后阅读原文,可获得下载资料的方法. 成熟的人工智能(AI)和物联网(IoT)技术能够快速改善道路安全的潜力在很大程度上被忽视了.一方面,道路投资仍然集中在传统的物理基础设施上,而另一方面,技术人 ...
- 人工智能驱动的智能制造与工业4.0(人工智能大数据公司)
全球大型制造商正通过深度学习技术进行质量检测,能够有效检测产品上的裂纹.不均匀边缘和划痕等产品缺陷,并通过深度学习预测重型设备失效时间,提前进行预测性维护.广泛应用于生产流水线的人工智能机器人实现制造 ...
最新文章
- 黄聪:如何使用CodeSmith批量生成代码(转:http://www.cnblogs.com/huangcong/archive/2010/06/14/1758201.html)...
- K8S集群的搭建:环境准备及相关命令
- java数据传递给安卓_Android数据传递的五种方法汇总
- flink配置+停止flink中的job
- CMake常用命令整理
- “国家科学数据中心”联合专刊征稿
- 手把手教你用FineBI,快速搭建可视化驾驶舱
- ReferenceError: XXX is not defined 错误及解决办法
- 变形二叉树中节点的最大距离(树的最长路径)——非递归解法
- LVM详解;Linux逻辑卷管理
- SQLserver添加主键
- jQuery报错:Uncaught ReferenceError: $ is not defined
- 为什么wait和notify必须在同步方法或同步块中调用?
- 二维码生成,打包下载zip,BigDecimal的取值和计算,java发送http请求
- 薄板样条插值(Thin Plate Spline)
- xsmax进入dfu模式_没有home键的iPhone XS怎么进入恢复模式?我教你
- 纯数学教程 Page 325 例LXVIII (4) 比值判别法和达朗贝尔判别法失效的一种情形...
- bootmgr is missing错误:首先检查系统分区是否为活动分区
- Deltix Round, Autumn 2021 (open for everyone, rated, Div. 1 + Div. 2)
- CAD修复块中心(com接口c#语言)
热门文章
- 前端js、jQuery实现日期格式化、字符串格式化
- Android基于mAppWidget实现手绘地图(九)–如何处理地图对象的touch事件
- 玩转 Python 3.5 的 await/async
- Java 引用jar包
- yolov5数据集标注txt2xml和xml2txt
- 190519每日一句
- 传智播客黑马 Python学习笔记之python初识
- unity矩阵运算,数学计算
- Atitit nlp重要节点 v3 目录 1. 语法分析重点 节点余额365个 1 2. nlp词性表 2 2.1. 词语分类13类 2 2.2. 副词 约20个 3 2.3. 代词30个 3 2
- Atitit refact art 重构的艺术 目录 1. Concept	1 1.1. Bp	1 2. Prob	2 3. Tool	2 1.Concept 	1. legacy code遗留代