python 数据框缺失值_Python:处理数据框中的缺失值
python 数据框缺失值
介绍 (Introduction)
In the last article we went through on how to find the missing values. This link has the details on the how to find missing values in the data frame. https://medium.com/@kallepalliravi/python-finding-missing-values-in-a-data-frame-3030aaf0e4fd
在上一篇文章中,我们探讨了如何找到缺失的值。 该链接包含有关如何在数据框中查找缺失值的详细信息。 https://medium.com/@kallepalliravi/python-finding-missing-values-in-a-data-frame-3030aaf0e4fd
Now that you have identified all the missing values, what to do with these missing values? In this article we will go over on how to handle missing data in a data frame.
现在,您已经确定了所有缺失值,如何处理这些缺失值? 在本文中,我们将探讨如何处理数据帧中的丢失数据。
There are multiple ways of handling missing data and this varies case by case. There is no universal best way in dealing with the missing data. Use your best judgement and explore different options to determine which method is best for your data set.
有多种处理丢失数据的方法,具体情况视情况而定。 没有通用的最佳方法来处理丢失的数据。 根据您的最佳判断,探索不同的选项,以确定哪种方法最适合您的数据集。
Deleting all rows/columns with missing data: This can be used when you have rows/columns where majority of the data is missing. When you are deleting rows/columns you might be losing some valuable information and lead to biased models. So analyze your data before deleting and check if there is any particular reason for missing data.
删除所有缺少数据的行/列 :当您缺少大部分数据的行/列时,可以使用此方法。 当您删除行/列时,您可能会丢失一些有价值的信息,并导致模型有偏差。 因此,请在删除数据之前分析您的数据,并检查是否有任何特殊原因导致数据丢失。
Imputing data: This is by far the most common way used to handle missing data. In this method you impute a value where data is missing. Imputing data can introduce bias into the datasets. Imputation can be done multiple ways.
估算数据 :这是迄今为止处理缺失数据的最常用方法。 在此方法中,您将在缺少数据的地方估算一个值。 估算数据可能会使数据集产生偏差。 插补可以通过多种方式完成。
a. You can impute mean, median or mode values of a column into the missing values in a column.
一个。 您可以将一列的均值,中位数或众数值插入一列的缺失值中。
b. You use predictive algorithms to impute missing values.
b。 您可以使用预测算法来估算缺失值。
c. For categorical variables you can label missing data as a category.
C。 对于分类变量,可以将缺少的数据标记为类别。
For this exercise we will use the Seattle Airbnb data set which can be found in the below link. https://www.kaggle.com/airbnb/seattle?select=listings.csv
在本练习中,我们将使用Seattle Airbnb数据集,该数据集可在下面的链接中找到。 https://www.kaggle.com/airbnb/seattle?select=listings.csv
Load the data and find the missing values.
加载数据并找到缺少的值。
The details of this steps can be found in the previous post under the below link. https://medium.com/@kallepalliravi/python-finding-missing-values-in-a-data-frame-3030aaf0e4fd
有关此步骤的详细信息,请参见上一篇文章的以下链接。 https://medium.com/@kallepalliravi/python-finding-missing-values-in-a-data-frame-3030aaf0e4fd
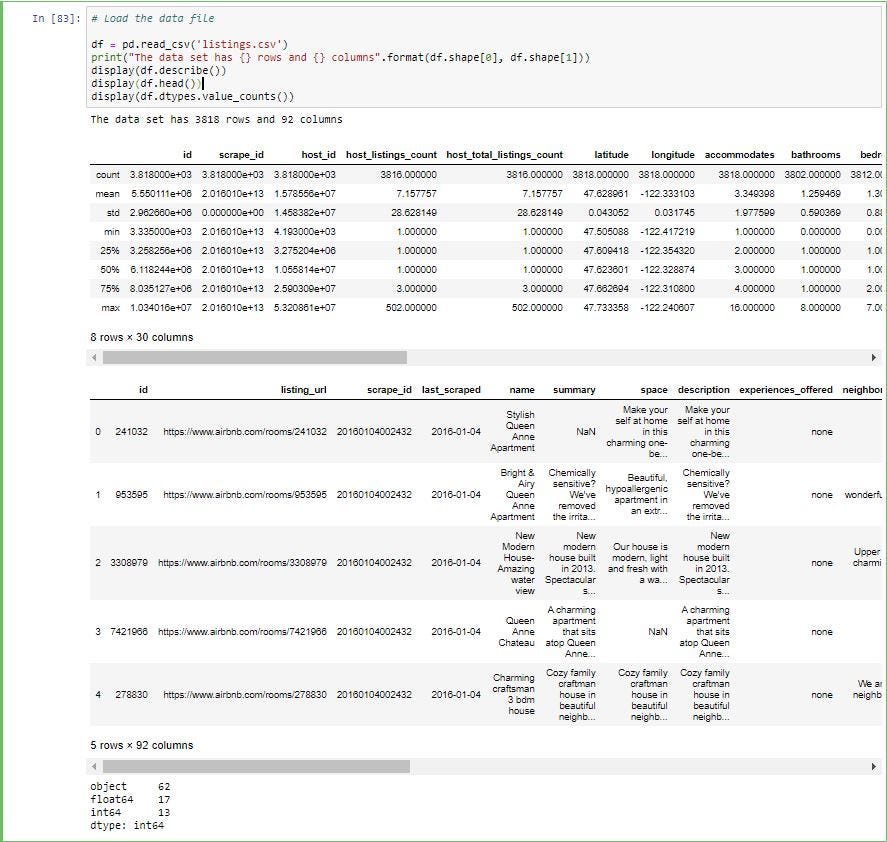
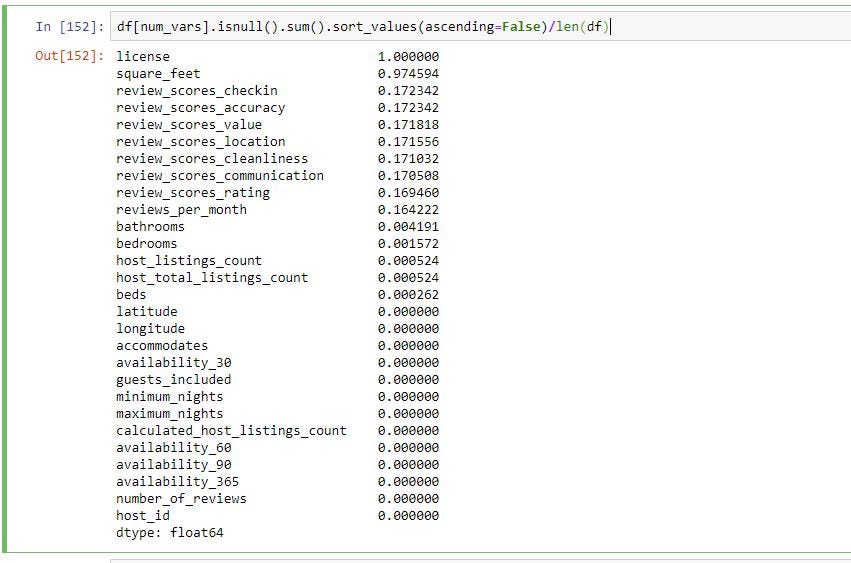
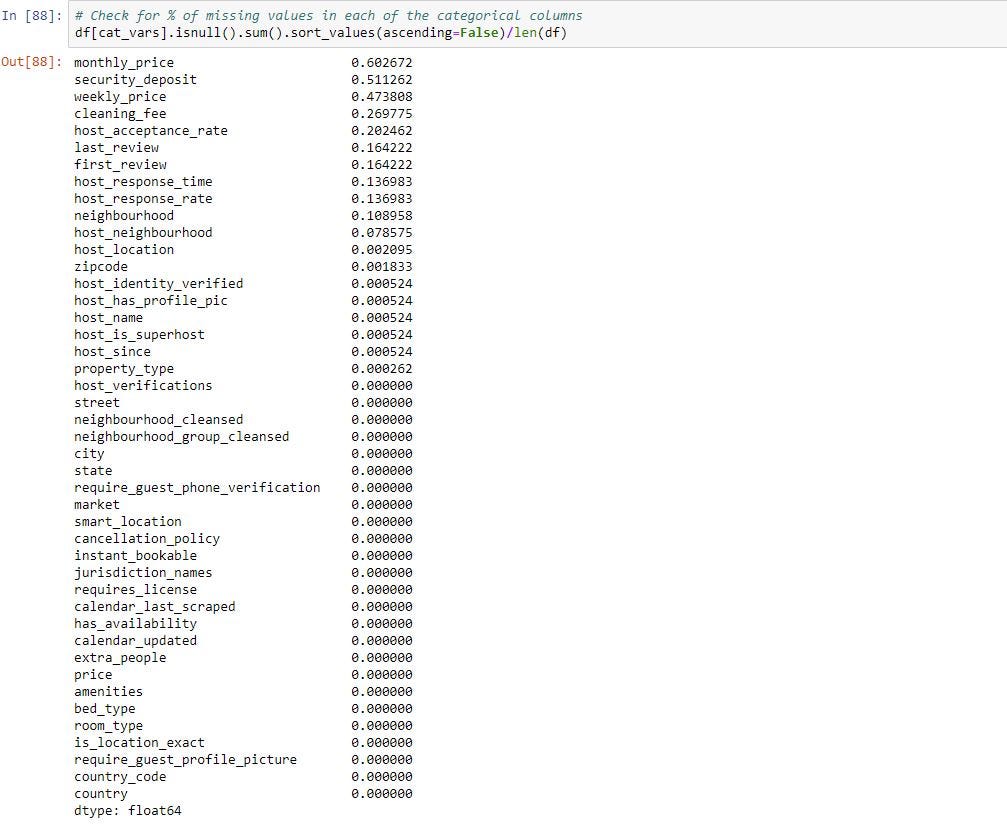
1.删除缺少数据的行/列: (1. Deleting rows/columns with missing data:)
Deleting Specific rows/columns
删除特定的行/列
From the above you can see that 100% of the values in license column and 97% of the square_feet column are missing data in numerical columns.
从上面可以看到,许可证列中的100%的值和square_feet列中的97%的值在数字列中丢失。
60% of the values in monthly_price, 51% of values in security_deposit and 47% of values in weekly_price are missing data
缺少数据的month_price中的值的60%,security_deposit中的51%的值和weekly_price中的47%的值
Lets try deleting these 5 columns.
让我们尝试删除这5列。
Pandas drop function can be used to delete rows and columns. Full details of this function can be found in the below https://pandas.pydata.org/pandasdocs/stable/reference/api/pandas.DataFrame.drop.html
熊猫拖放功能可用于删除行和列。 可以在下面的https://pandas.pydata.org/pandasdocs/stable/reference/api/pandas.DataFrame.drop.html中找到此功能的完整详细信息。
All columns which should be deleted should be included in columns parameter. axis =1 represents column, axis=0 represent rows. In the case we are telling to delete all columns specified in the columns parameter.
应该删除的所有列都应包含在columns参数中。 轴= 1代表列,轴= 0代表行。 在这种情况下,我们告诉您删除columns参数中指定的所有列。

As you can see below now you do not have columns which have been deleted.
如下所示,您现在没有已删除的列。
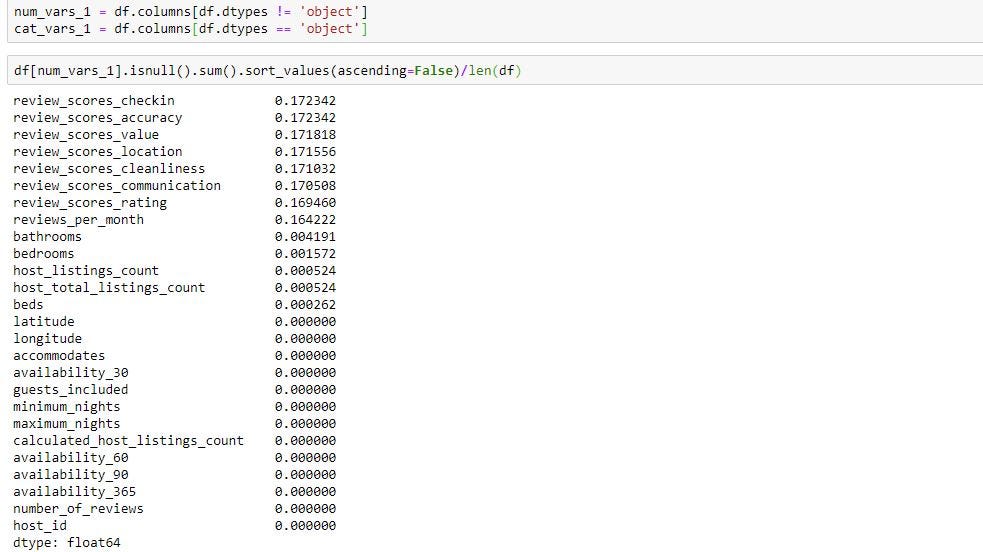
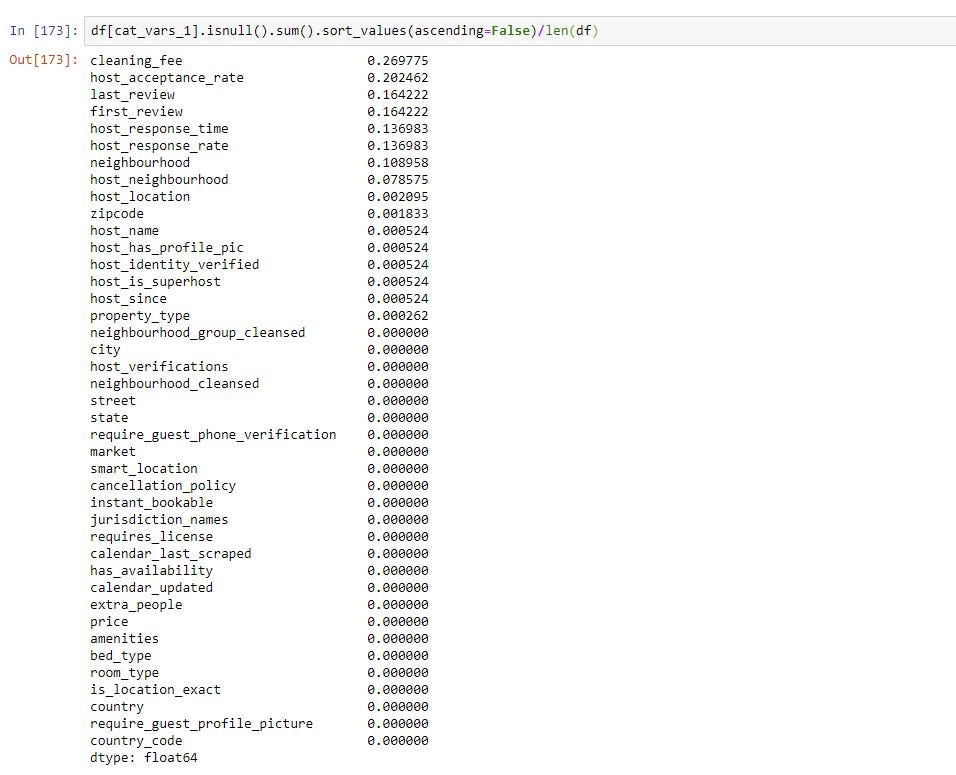
Deleting rows/columns with NA
用NA删除行/列
If you want to delete rows/columns with NA we can use dropna function in pandas. Details of this function can be found in the below link. https://pandas.pydata.org/pandas-docs/stable/reference/api/pandas.DataFrame.dropna.html
如果您想使用NA删除行/列,我们可以在熊猫中使用dropna函数。 可以在下面的链接中找到此功能的详细信息。 https://pandas.pydata.org/pandas-docs/stable/reference/api/pandas.DataFrame.dropna.html
dropna function has multiple parameters, the 3 main ones are
dropna函数有多个参数,其中三个主要参数是
- how : this has 2 options “any” or “all”. If you set to “any” even if one value has NA in row or column it will delete those columns. If you set to “all” only if all the values in rows/columns have NA deletion will happen.方式:这有2个选项“任何”或“全部”。 如果您设置为“ any”,即使一个值在行或列中具有NA,它将删除这些列。 如果仅将行/列中的所有值都具有NA删除,则设置为“所有”。
- axis : this can be set to 0 or 1. If 0 then drops rows with NA values, if 1 then drops columns with NA values.axis:可以将其设置为0或1。如果为0,则删除具有NA值的行,如果为1,则删除具有NA值的列。
- subset: if you want the operation to be performed only on certain columns then mention the column name int he subset. If subset is not define then the operation is performed on all the columns.子集:如果您希望仅对某些列执行操作,请在子集中提及列名。 如果未定义子集,则对所有列执行该操作。

2.估算数据 (2. Imputing Data)
With imputing you are trying to assign a value through inference from the values to which it contributes. In this case you are assigning a value in the place of a missing value by using different methods on the feature which has missing value. Methods can as simple as assigning mean, median, mode of the column to the missing values or you can use machine learning techniques to predict the missing values. Imputation methods can be different for numerical and categorical variables.
使用插补时,您试图通过推断贡献值来分配一个值。 在这种情况下,您可以通过对具有缺失值的要素使用不同的方法来为缺失值分配一个值。 方法可以简单到为缺失值分配列的均值,中位数,众数模式,也可以使用机器学习技术来预测缺失值。 数值和分类变量的插补方法可能不同。
Imputation for Numerical values:
数值的估算:
With numerical columns the most common approach to impute data is by imputing mean, median or mode of the column in place of the missing values.
对于数字列,最常用的估算数据方法是通过估算列的均值,中位数或众数来代替缺失值。
To do that we will write a function to fill na with mean/median/mode and then apply that function to all the columns.
为此,我们将编写一个用均值/中位数/众数填充na的函数,然后将该函数应用于所有列。
In the below i am showing a example to fill the missing data with the mean of the column.
在下面的示例中,我展示了使用列的平均值填充缺失数据的示例。
fill_mean function iterates through each column in the data frame and fill’s na with the column mean.
fill_mean函数遍历数据帧中的每一列,并用列均值填充na。
You can then use apply() function to apply fill_mean function on one column or multiple columns in a data frame.
然后,您可以使用apply()函数将fill_mean函数应用于数据框中的一列或多列。
This example shows using mean, you can use median() and mode() function in place of mean() if you want to impute median or mode of the column .
此示例显示了使用均值,如果要对列的中值或众数进行插值,则可以使用mean()和mode()函数代替mean()。

Imputation for Categorical values:
分类值的插补:
For categorical variables clearly you cannot use mean or median for imputation. But we can use mode which is use the most frequently used value or the one other way is to missing data as category by itself.
显然,对于分类变量,您不能使用均值或中位数进行插补。 但是我们可以使用使用最常用值的模式,或者另一种方法是单独丢失数据作为类别。
Since i have already went through on how to impute most frequently value, in this step i will show how make a missing data as a category. This is very straight forward, you just replace NA with “missing data” category. Missing data will be one of the levels in each categorical variable.
由于我已经介绍了如何估算最频繁的值,因此在这一步中,我将说明如何将缺失的数据作为类别。 这很简单,您只需将NA替换为“缺少数据”类别。 丢失的数据将是每个分类变量中的级别之一。

Imputation using a model to predict missing values:
使用模型进行插补以预测缺失值:
One more option is to use model to predict missing values. To perform this task you can IterativeImputer from sklearn library. You can find details on this in the below link
另一种选择是使用模型来预测缺失值。 要执行此任务,您可以从sklearn库中获取IterativeImputer。 您可以在以下链接中找到详细信息
https://scikit-learn.org/stable/modules/generated/sklearn.impute.IterativeImputer.html
https://scikit-learn.org/stable/modules/generation/sklearn.impute.IterativeImputer.html
Iterative imputer considers features with missing values and develops a model as function of other features. It then estimates the missing value and imputes those values.
迭代冲刺者会考虑具有缺失值的要素,并根据其他要素开发模型。 然后,它估计缺失值并估算这些值。
It does it in a iterative manner, meaning it will take a 1st feature with missing values which it considers as response variable and considers all the other features as input variables. Using these input variables it will estimate the values for the missing values in the response variable. In the next step it will consider the 2nd feature with missing values as response variable and use all the other features as input variables and estimate missing values. This process will continue until all the features with missing values are addressed.
它以迭代方式进行,这意味着它将采用第一个具有缺失值的特征,将其视为响应变量,并将所有其他特征视为输入变量。 使用这些输入变量,它将估计响应变量中缺少的值的值。 在下一步中,它将把具有缺失值的第二个特征视为响应变量,并将所有其他特征用作输入变量并估计缺失值。 此过程将继续进行,直到解决所有缺少值的功能。
In the below example i am using Random forest in the imputer to estimate the missing values and fitting the imputer to a data frame.
在下面的示例中,我将在嵌入式计算机中使用随机森林来估计缺失值,并将嵌入式计算机拟合到数据帧。
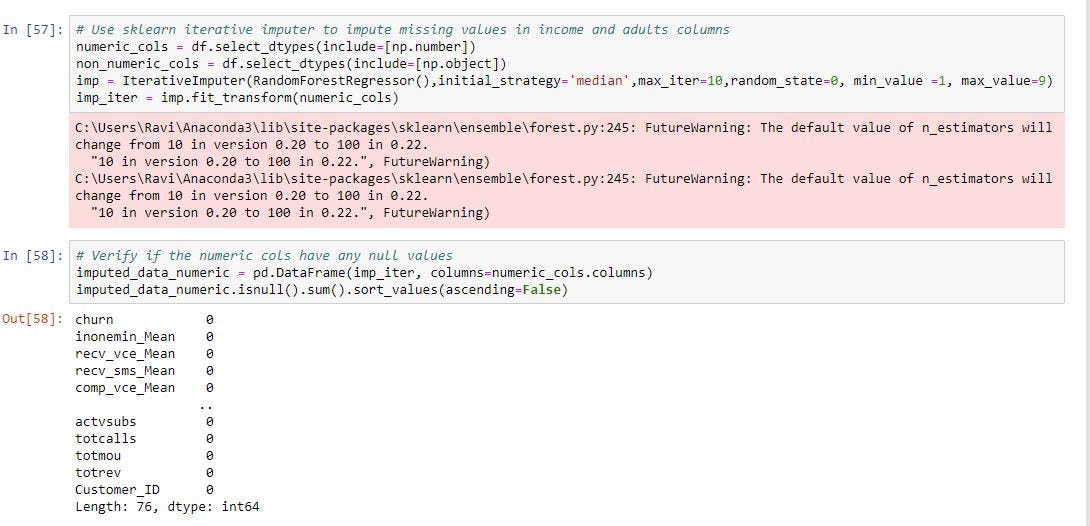
结论: (Conclusion:)
In this article we went through on how to handle the missing values in a data frame.
在本文中,我们探讨了如何处理数据框中的缺失值。
- Delete the rows/columns with missing values删除缺少值的行/列
- Imputing the missing values with statistic like mean, mean or mode.用均值,均值或众数等统计数据来估算缺失值。
- For categorical variables making missing data as a category.对于类别变量,将缺少的数据作为类别。
- Using Iterative Imputer develop a model to predict missing values in each of the features.使用Iterative Imputer开发一个模型来预测每个功能部件中的缺失值。
翻译自: https://medium.com/analytics-vidhya/python-handling-missing-values-in-a-data-frame-4156dac4399
python 数据框缺失值
http://www.taodudu.cc/news/show-997427.html
相关文章:
- 外星人图像和外星人太空船_卫星图像:来自太空的见解
- 棒棒糖 宏_棒棒糖图表
- nlp自然语言处理_不要被NLP Research淹没
- 时间序列预测 预测时间段_应用时间序列预测:美国住宅
- 经验主义 保守主义_为什么我们需要行动主义-始终如此。
- python机器学习预测_使用Python和机器学习预测未来的股市趋势
- knn 机器学习_机器学习:通过预测意大利葡萄酒的品种来观察KNN的工作方式
- python 实现分步累加_Python网页爬取分步指南
- 用于MLOps的MLflow简介第1部分:Anaconda环境
- pymc3 贝叶斯线性回归_使用PyMC3估计的贝叶斯推理能力
- 朴素贝叶斯实现分类_关于朴素贝叶斯分类及其实现的简短教程
- vray阴天室内_阴天有话:第1部分
- 机器人的动力学和动力学联系_通过机器学习了解幸福动力学(第2部分)
- 大样品随机双盲测试_训练和测试样品生成
- 从数据角度探索在新加坡的非法毒品
- python 重启内核_Python从零开始的内核回归
- 回归分析中自变量共线性_具有大特征空间的回归分析中的变量选择
- python 面试问题_值得阅读的30个Python面试问题
- 机器学习模型 非线性模型_机器学习:通过预测菲亚特500的价格来观察线性模型的工作原理...
- pytorch深度学习_深度学习和PyTorch的推荐系统实施
- 数据库课程设计结论_结论:
- 网页缩放与窗口缩放_功能缩放—不同的Scikit-Learn缩放器的效果:深入研究
- 未越狱设备提取数据_从三星设备中提取健康数据
- 分词消除歧义_角色标题消除歧义
- 在加利福尼亚州投资于新餐馆:一种数据驱动的方法
- 近似算法的近似率_选择最佳近似最近算法的数据科学家指南
- 在Python中使用Seaborn和WordCloud可视化YouTube视频
- 数据结构入门最佳书籍_最佳数据科学书籍
- 多重插补 均值插补_Feature Engineering Part-1均值/中位数插补。
- 客户行为模型 r语言建模_客户行为建模:汇总统计的问题
python 数据框缺失值_Python:处理数据框中的缺失值相关推荐
- python处理csv文件缺失值_Python:如何处理CSV中的缺失值?
我有一个给定的CSV示例,如下所示: ID,ID_TYPE,OB_DATE,VERSION_NUM,MET_DOMAIN_NAME,OB_END_CTIME,OB_DAY_CNT,SRC_ID,REC ...
- python处理csv文件缺失值_python处理数据中缺失值
(1)直接删除法(当缺失值个数只占整体很小一部分时) 在采用删除法处理缺失值时,需要首先检测样本总体中确实值得的个数.python中统计缺失值的方法为: import numpy as np impo ...
- 如何用python做无限弹窗_python弹出框
广告关闭 腾讯云11.11云上盛惠 ,精选热门产品助力上云,云服务器首年88元起,买的越多返的越多,最高返5000元! super(winform, self).init(parent) self.r ...
- python爬虫写入数据库_Python爬虫数据写入操作
Python Scrapy爬虫数据写入操作 在我们写完一个爬虫项目,得到了一大堆的数据,为了以后的分析和使用,我们需要把我们得到的数据进行保存.保存数据的方式主要有:保存到数据库,保存到CSV文件,保 ...
- python 导入离线地图_Python绘制数据地图可以应用与各种场景,只需要更改数据就行。...
前言 本文的文字及图片来源于网络,仅供学习.交流使用,不具有任何商业用途,版权归原作者所有,如有问题请及时联系我们以作处理. 主要用到的新的Python模块是Geopandas,就是利用它来绘制数据地 ...
- python数据存储方式_python各数据存储方法
声明: 1)仅作为个人学习,如有冒犯,告知速删! 2)不想误导,如有错误,不吝指教! 本节内容: 简介 ------------------文件存储------------------ TXT文本存储 ...
- python降维之时间类型数据的处理_Python学习数据降维方法
使用sklearn库初次尝试PCA和T-SNE,原理还不太理解,写错了请一定指出,或者等我自己发现hhhh 1. PCA 首先读入sklearn里自带的鸢尾花数据库,并调用相关的包,再查看一下这些数据 ...
- 用python做透视表_python制作数据透视表pivot_table方法详解
数据透视表(Pivot Table)是一种交互式的表,可以进行某些计算,如求和与计数等.所进行的计算与数据跟数据透视表中的排列有关. 之所以称为数据透视表,是因为可以动态地改变它们的版面布置,以便按照 ...
- python 可视化分析平台_python 数据分析数据可视化工具matplotlib
说明: 数据可视化中的数据集下载地址:(数据来源:从零开始学python数据分析和挖掘) 链接:https://pan.baidu.com/s/1zrNpzSNVHd8v1rGFRzKipQ 提取码: ...
- python数字类型转换函数_Python的数据类型转换函数
玩蛇网这篇文章给大家介绍关于,Python数据类型的转换函数. Python提供了一些可以把某个值从一种数据类型,转换成为另一种数据类型的内置函数和方法.int函数可以将任何可以转换为整型的值转换为整 ...
最新文章
- C#读取资源文件的两种方法及保存资源文件到本地
- [即将举行的网络研讨会]对Kubernetes进行故障排除:您需要具备的7个关键组件
- 使用ITEXT操作PDF文件
- BBED for PostgreSQL
- js 在html中新建个节点,javascript节点是什么?
- react循环key值_01 React快速入门(一)——使用循环时对于‘key’报错处理
- 网络编程~socket
- Quartz配置文件
- win11电脑快捷键
- c语言编程学习宝典,C语言学习宝典
- WPF——自定义日历
- 【Uly】微软产品开发中的“战争与和平”
- 有关串口、USB接口串联小电阻的对话
- 使用IDA对ipa进行反编译
- Mybatis与JPA的优缺点
- idea中module项目没有蓝色小方块问题
- [BJOI2019]排兵布阵
- ASCII-from baidubaike
- 惠普笔记本连接wifi无internet,手机连接正常
- 2小时学会CSS,完成网页制作!
热门文章
- C++ 网络开发工具
- Educational Codeforces Round 25 C. Multi-judge Solving
- CentOS6 下Samba服务器的安装与配置
- 408. Valid Word Abbreviation
- 跳过 centos部署 webpy的各种坑
- QT连接多种数据库f方法及测试
- iOS开发学无止境 - NSFileManager文件操作的十个小功能
- Oracle 11g 新特性 -- 自适应游标共享(Adaptive Cursor Sharing: ACS) 说明
- 【转载】用Snort巧妙检测SQL注入和跨站脚本攻击
- Activiti 简易教程