如何识别媒体偏见_面部识别,种族偏见和非洲执法
如何识别媒体偏见
Many black people presume that the comment, “they all look alike to me”, is the way the rest of the world sees us.
许多黑人认为,“其他人对我看起来都一样”的评论是全世界其他人看到我们的方式。
But research has shown that, although this facial ambiguity is a problem, it’s not unique to black people.
但是研究表明,尽管这种面部歧义是一个问题,但这并不是黑人所独有的。
The human brain de-individualizes faces belonging to groups that we don’t belong to. This behavior is known as the cross-race effect.
人脑将属于我们不属于的组的面Kong去个体化。 这种行为称为交叉竞赛效应 。
Now, if I didn’t know better, I’d have sworn that scientists were doing their best to all-lives-matter the conversation, but there is evidence that, to people of a different race, people of another race do look alike.
现在,如果我不了解,我会发誓科学家们正在全力以赴地进行谈话,但是有证据表明,对于不同种族的人来说,另一个种族的确看起来很像。
That said, the well-documented shortcomings of modern facial recognition technologies are forcing scientists to consider a different, but ultimately, related set of questions.
也就是说,现代人脸识别技术的有据可查的缺点正迫使科学家考虑一系列不同但最终相关的问题。
At a basic level, the goal of artificial intelligence is to simulate the human brain — General AI, or even exceed its intelligence — Super AI.
从根本上讲, 人工智能的目标是模拟人脑-通用AI,甚至超越其智能-超级AI。
But so far, what we’ve been able to do is simulate a subset of human functions — Narrow AI.
但是到目前为止,我们已经能够做的是模拟人类功能的一个子集-Narrow AI。
This field of AI operates within a pre-determined, pre-defined range, and replicates a specific human behaviour based on specific parameters and contexts.
AI的这一领域在预定的,预定的范围内运行,并根据特定的参数和上下文复制特定的人类行为。
While this is no doubt an impressive feat, the cross-race effect isn’t extensible to lower forms of intelligence. What we’ve found, instead, is that the deep learning algorithms that we use in narrow AI might have inherited some deep racial biases.
尽管这无疑是一项令人印象深刻的壮举,但交叉种族效应无法扩展到较低形式的智力。 相反,我们发现,在狭窄的AI中使用的深度学习算法可能继承了一些深深的种族偏见 。
Facial recognition technology has been around since the mid-’60s. But in recent years, the emergence of deep learning has accelerated its adoption in numerous use cases including law enforcement.
面部识别技术自60年代中期开始出现。 但是近年来,深度学习的出现加速了其在包括执法在内的许多用例中的采用。
However, it’s still an imperfect technology.
但是,它仍然是不完善的技术。
US government tests found that, as recently as 2019, even top-performing facial recognition systems misidentified blacks at rates five to ten times higher than they did whites.
美国政府的测试发现,直到2019年,即使是表现最好的面部识别系统,对黑人的识别率也比白人高出五到十倍。
Depending on the use case, the effects of misidentification can be anything from mildly irritating — like in 2009, when an HP webcam designed to track people’s faces, tracked a white worker but not her black colleague — to deeply insulting, like in 2015, when Google Photos classified some black people as gorillas.
视使用情况而定, 误识别的影响可能从轻度的刺激(例如在2009年,当时是设计用于追踪人脸的HP网络摄像头,追踪了白人而不是黑人同事)到深深的侮辱(例如2015年) Google相册将一些黑人归为大猩猩。
But in the context of law enforcement, while it has been successful in fighting crime in some locales, a single case of mistaken identity could be the difference between freedom and incarceration, or worse still, between life and death.
但是在执法的背景下,尽管在某些地区已经成功地打击了犯罪,但唯一一个身份错误的案例可能是自由与监禁之间的区别,或者甚至是生与死之间的区别。
In what might be the first known case of a wrongful arrest caused by inaccurate facial recognition technology, Robert Julian-Borchak Williams was recently arrested in Detroit, after a facial recognition system falsely matched his photo with security footage of a shoplifter.
在什么可能是造成错误的面部识别技术错误逮捕的第一个已知的情况下, 罗伯特·朱利安-威廉姆斯Borchak是最近逮捕在底特律,面部识别系统后,错误匹配他的商店扒手的安全录像照片。
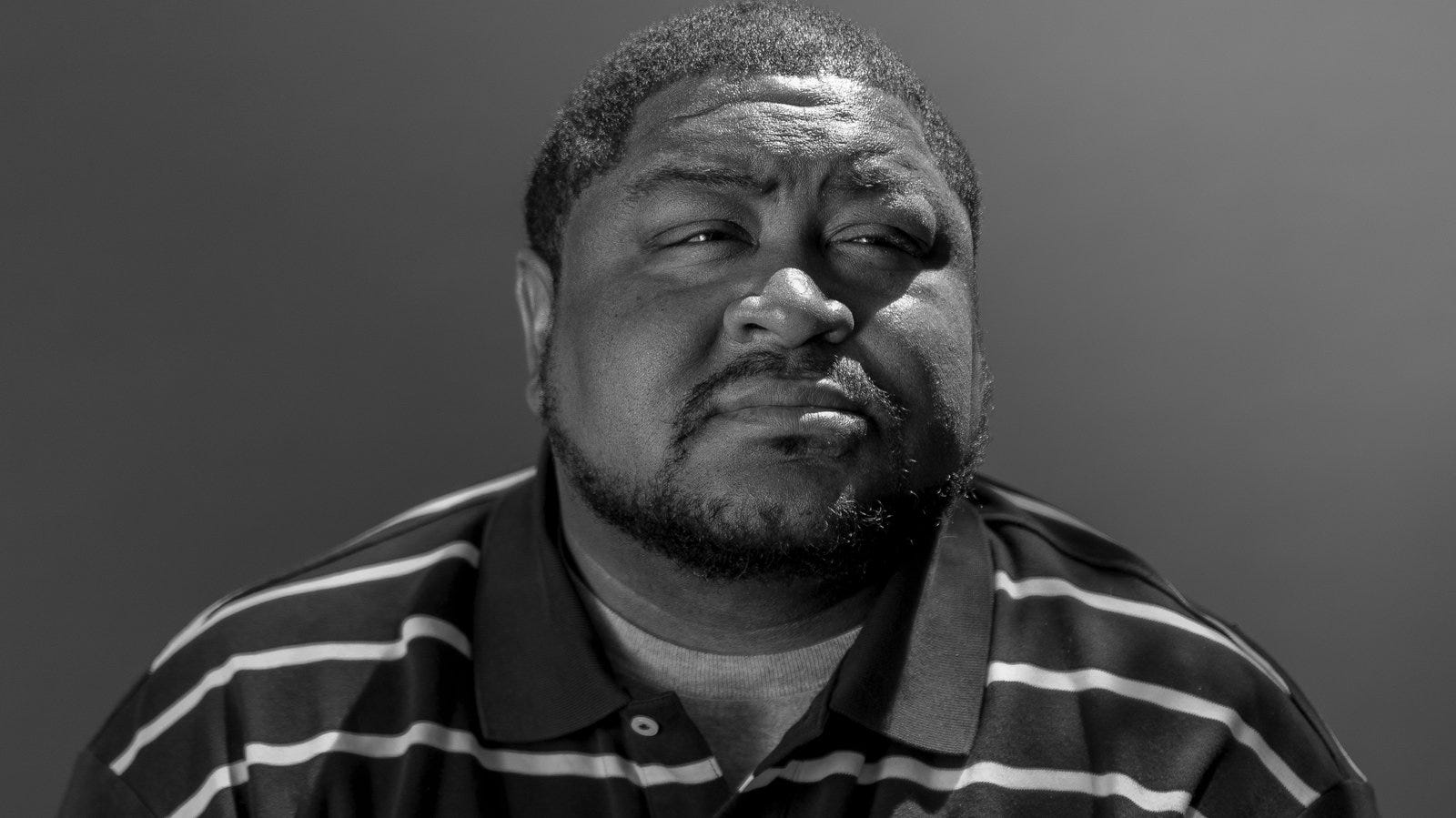
Williams is African-American.
威廉姆斯是非洲裔美国人。
His story is, coincidentally, coming at the height of tensions between Black America and the police force.
巧合的是,他的故事是在《黑人美国》与警察之间的紧张局势达到顶峰之时发表的。
The charged atmosphere has forced multiple western tech companies, including IBM, Microsoft, and Amazon, to announce that they’ll be pausing or stopping their facial recognition work for the police.
充斥的气氛迫使包括IBM,微软和亚马逊在内的多家西方科技公司宣布将暂停或停止警方的面部识别工作。
Even though there have been advancements in the space, the margin for error when identifying black faces is still far too high. And it was only a matter of time before an incident like this occurred.
即使空间有所进步,识别黑脸时的误差幅度仍然过高。 发生这样的事件只是时间问题。
The decision of the tech giants to put their programs on hold is the biggest indictment on current facial recognition systems. The reason why the technology performs so differently for darker skin tones is still unclear, but there are at least two plausible explanations:
科技巨头决定暂停其程序是当前面部识别系统的最大指控。 尚不清楚该技术对深色肤色有如此显着差异的原因,但至少有两个合理的解释:
1.黑人人数不足 (1. Black people are underrepresented)
MIT researcher and digital activist Joy Buolamwin has made racial biases in facial recognition technology her life’s work. She’s put forward the theory that, in the datasets used to test or train facial recognition systems, black people are not properly represented.
麻省理工学院的研究员和数字活动家Joy Buolamwin使面部识别技术中的种族偏见成为了她一生的工作。 她提出了一种理论,即在用于测试或训练面部识别系统的数据集中,黑人没有得到适当的代表。
An AI system is only as good as its data. Respected AI researcher Robert Mercer famously said:
一个AI系统仅与其数据一样好。 备受尊敬的AI研究员Robert Mercer曾有句著名的话:
“There’s no data like more data.”
“没有数据像更多的数据。”
The easiest place to “harmlessly” harvest large amounts of photos of faces is the web. Being the largest contributors to the global internet economy, online content tends to skew very male, very white, and very western. It also doesn’t help that it’s that same demo that’s largely responsible for building western AI algorithms.
网络是“无害”采集大量面部照片的最简单的地方。 作为全球互联网经济的最大贡献者,在线内容倾向于偏向非常男性,非常白人和非常西方的人群。 同样的演示主要负责构建西方AI算法,这也无济于事。
2.黑色的光致缺陷 (2. The black photogenicity deficit)
There’s another argument that the lower accuracy on darker skin can be traced back to the beginnings of color film. Photographic technology has always been optimized for lighter skin, and the digital photography we use today is built on the same principles that shaped early film photography. According to this school of thought, narrow AI is having difficulty recognizing black faces simply because modern photography wasn’t designed with the facial features of black people in mind.
还有一个论点是,深色皮肤上较低的准确度可以追溯到彩色胶卷的开始。 摄影技术一直以来都针对较轻的皮肤进行了优化,而我们今天使用的数字摄影基于塑造早期胶片摄影的相同原理。 按照这个思想流派,狭窄的AI很难识别黑脸,这仅仅是因为现代摄影并非在设计时就考虑到了黑人的面部特征。
In western countries where blacks are in the minority, these built-in biases significantly impact the quality of facial recognition-assisted law enforcement. However, in the continent with the largest population of black people, the potential for harm is exponentially greater.
在黑人占少数的西方国家,这些内在偏见极大地影响了面部识别辅助执法的质量。 但是,在黑人人口最多的大陆上,潜在的危害成倍增加。
The US and China are locked in a war over AI dominance.
美国和中国陷入了关于人工智能统治的战争。
According to renowned AI researcher and investor Kai-Fu Lee, there are 7 giants of the AI age, namely:
根据著名的AI研究人员和投资者Lee Kai-Fu的说法,有7个AI时代的巨人:
- Google谷歌
- Facebook脸书
- Microsoft微软
- Amazon亚马孙
- Tencent腾讯网
- Baidu百度
- Alibaba阿里巴巴
Currently, it’s almost an even split between the US companies and the Chinese companies. Some analysts believe that Africa could be the final battleground.
目前,这几乎是美国公司和中国公司之间的差距。 一些分析人士认为,非洲可能是最后的战场。
If so, then it’s a battle that US businesses are currently losing.
如果是这样,那么这是美国企业目前正在输掉的一场战斗。
There have been interesting, one-off developments, like Google opening its first AI lab in Ghana last year, but the US has largely been cool on exploring the continent’s AI and data potential.
有一些有趣的一次性开发项目,例如Google去年在加纳开设了第一个AI实验室,但美国在探索非洲大陆的AI和数据潜力方面一直很冷静。
This has handed China a significant advantage, particularly in face recognition.
这给中国带来了巨大的优势,特别是在人脸识别方面 。
In recent years, the Chinese tech giant, Huawei, has been pushing its flagship public safety solution: Safe City. Built on CCTV and facial recognition technologies, the solution provides local authorities with modern tools for law enforcement.
近年来,中国科技巨头华为一直在推动其旗舰产品公共安全解决方案:安全城市。 该解决方案以闭路电视和面部识别技术为基础,为地方当局提供了现代化的执法工具。
According to the Center for Strategic and International Studies (CSIS), a US-based think tank, there are currently twelve Safe City programs operational in sub-Saharan Africa, including in Kenya, Uganda and South Africa.
根据美国智库战略与国际研究中心(CSIS)的数据,目前在撒哈拉以南非洲(包括肯尼亚,乌干达和南非)有十二个“安全城市”计划正在实施。
Questions have been raised about privacy, data protection, and aiding and abetting authoritarian regimes. But, on the other hand, there have also been success stories. Like in Nairobi, where Huawei claims that the initiative led to a 46% reduction in the crime rate.
有关隐私,数据保护以及协助和教,威权政权的问题已经提出。 但是,另一方面,也有成功的故事。 就像内罗毕一样,华为声称该倡议使犯罪率降低了46% 。
It’s, however, instructive that information about false positives and wrongful arrests has, so far, been opaque.
但是,到目前为止,关于误报和错误逮捕的信息是不透明的,这是有启发性的。
In 2018, Chinese AI startup, CloudWalk, signed a deal with Zimbabwean President Emmerson Mnangagwa. Mnangagwa has shown a tendency to use digital tools and the power of the law to restrict civil liberties but that’s not the only thing troubling about the CloudWalk deal.
2018年,中国AI初创企业CloudWalk与津巴布韦总统埃默森·曼纳格格瓦(Emmerson Mnangagwa)签署了一项协议 。 Mnangagwa已经显示出倾向于使用数字工具和法律的力量来限制公民自由的趋势,但这并不是CloudWalk协议唯一令人困扰的事情。
As part of the agreement, Harare has been sending data on millions of black faces to the Chinese company, this is helping to train their technology toward darker skin tones.
作为协议的一部分,哈拉雷(Harare)已向该中国公司发送了数百万张黑脸的数据,这有助于将其技术训练成深色肤色。
It’s a brazen data-for-dollars swap on a national level.
在全国范围内,这是一笔大胆的以美元换数据的交易。
The CloudWalk-Zimbabwe agreement offers a glimpse into the deficit in global facial recognition technology that Chinese companies are trying to make up. These companies are benefiting from the general absence of laws that cover biometric data and cross-border flows of sensitive information.
CloudWalk-Zinbabwe协议可以窥见中国公司试图弥补的全球面部识别技术的不足。 这些公司受益于普遍缺乏涵盖生物识别数据和敏感信息的跨境流动的法律。
As Chinese AI companies continue to support local law enforcement, and conduct business with oppressive regimes, while simultaneously using black faces to train their datasets, there’s no telling how many Robert Julian-Borchak Williams there had been before there was Robert Julian-Borchak Williams.
随着中国AI公司继续支持当地执法部门,并在压迫性政权下开展业务, 同时使用黑脸训练他们的数据集,在罗伯特·朱利安·伯查克·威廉姆斯(Robert Julian-Borchak Williams)之前,并没有告诉我们有多少罗伯特·朱利安·伯查克·威廉姆斯(Robert Julian-Borchak Williams)。
Subscribe to the get.Africa newsletter, a weekly roundup of African tech in a language you’ll understand. A fresh email drops every Monday morning.
订阅 get.Africa 电子报,这是您每周都会用一种会听懂的语言浏览非洲技术的新闻。 每周一早上都会发送一封新电子邮件。
翻译自: https://medium.com/getdotafrica/facial-recognition-racial-bias-and-african-law-enforcement-9e85b4e39a3f
如何识别媒体偏见
http://www.taodudu.cc/news/show-1874003.html
相关文章:
- openai-gpt_GPT-3 101:简介
- YOLOv5与Faster RCNN相比。 谁赢?
- 句子匹配 无监督_在无监督的情况下创建可解释的句子表示形式
- 科技创新 可持续发展 论坛_可持续发展时间
- Pareidolia — AI的艺术教学
- 个性化推荐系统_推荐系统,个性化预测和优点
- 自己对行业未来发展的认知_我们正在建立的认知未来
- 汤国安mooc实验数据_用漂亮的汤建立自己的数据集
- python开发助理s_如何使用Python构建自己的AI个人助理
- 学习遗忘曲线_级联相关,被遗忘的学习架构
- 她玩游戏好都不准我玩游戏了_我们可以玩游戏吗?
- ai人工智能有哪些_进入AI有多么简单
- 深度学习分类pytorch_立即学习AI:02 —使用PyTorch进行分类问题简介
- 机器学习和ai哪个好_AI可以使您成为更好的运动员吗? 使用机器学习分析网球发球和罚球...
- ocr tesseract_OCR引擎之战— Tesseract与Google Vision
- 游戏行业数据类丛书_理论丛书:高维数据101
- tesseract box_使用Qt Box Editor在自定义数据集上训练Tesseract
- 人脸检测用什么模型_人脸检测模型:使用哪个以及为什么使用?
- 不洗袜子的高文博_那个孩子在夏天中旬用高袜子大笑?
- word2vec字向量_Anything2Vec:将Reddit映射到向量空间
- ai人工智能伪原创_AI伪科学与科学种族主义
- ai人工智能操控什么意思_为什么要建立AI分散式自治组织(AI DAO)
- 机器学习cnn如何改变权值_五个机器学习悖论将改变您对数据的思考方式
- DeepStyle(第2部分):时尚GAN
- 肉体之爱的解释圣经_可解释的AI的解释
- 机器学习 神经网络 神经元_神经网络如何学习?
- 人工智能ai应用高管指南_理解人工智能指南
- 机器学习 决策树 监督_监督机器学习-决策树分类器简介
- ai人工智能数据处理分析_建立数据平台以实现分析和AI驱动的创新
- 极限学习机和支持向量机_极限学习机的发展
如何识别媒体偏见_面部识别,种族偏见和非洲执法相关推荐
- 标记偏见_分析师的偏见
标记偏见 "Beware of the HiPPO in the room" - The risks and dangers of top-down, intuition-base ...
- 人工智能也存在偏见?探究人工智能偏见的识别和管理
摘译 | 李朦朦/赛博研究院实习研究员 来源 | NIST 2022年3月16日,美国国家标准与技术研究院(NIST)发布了<迈向识别和管理人工智能偏见的标准>(以下简称<标准> ...
- 如何识别媒体偏见_超越偏见:为什么我们不能仅仅“修正”面部识别
如何识别媒体偏见 In recent months, the troubling issue of facial recognition has hit headlines. 近几个月来,令人困扰的面 ...
- 如何识别媒体偏见_描述性语言理解,以识别文本中的潜在偏见
如何识别媒体偏见 TGumGum can do to bring change by utilizing our Natural Language Processing technology to s ...
- 生活中的观察者偏见例子_消除人工智能第2部分中的偏见,解决性别和种族偏见...
生活中的观察者偏见例子 Chatbots that become racist in less than a day, facial technology that fails to recogniz ...
- 人脸脸部识别技术_面部识别技术的道德安全问题
人脸脸部识别技术 Terminator and similar movies depict a world that is controlled by AI and robots. For the m ...
- 人脸脸部识别技术_面部识别技术的危险后果
人脸脸部识别技术 揭露 (Disclosure) The following introduction references existing technology and future advanc ...
- Jmeter识别登录验证码_使用百度AI图片识别技术
Jmeter识别登录验证码_使用百度AI图片识别技术 一.环境准备 1.下载并引用以下Jar包 2.将下载的jar包放至Jmeter中的lib目录中即可使用 二.使用步骤 1.在获得验证码的请求后使用 ...
- 人脸识别种族偏见:黑黄错误率比白人高100倍 | 美官方机构横评189种算法
赖可 发自 亚龙湾 量子位 报道 | 公众号 QbitAI 没错,美国人脸识别系统最爱的是:白人中年男性 其他非裔.亚裔族群,识别率就没那么高了. 这周四,美国NIST发布的研究报告给出了这样的结果. ...
- 标记偏见_协作和透明的机器学习可消除偏见
标记偏见 No one wants bias in their organization. Underrepresentation has plagued the business world for ...
最新文章
- koa-router让人迷惑的文档和源码实现
- oracle mysql sql serve where in 语句的不同
- bash-shell高级编程--退出和退出状态码
- 关于python pdb的描述_The python debugger(PDB)的简介
- Java技术分享:SpringBoot多模块开发
- 怎么使用socket在云服务上通信步骤(可支持TCP或UDP)
- Visio各图形如何一键自动对齐?
- 中countif函数_Count系列函数-Count、Counta、Countblank、Countif、Countifs
- vue 转换信息为二进制 并实现下载
- android 逆地址,Android高德获取逆地址编码(经纬度坐标-地址描述如省市区街道)
- Python—常用正则表达式方法
- ARC项目中部分类不用ARC
- 自律selfdiscipline
- 浙江工业大学python试卷_20浙江工业大学计算机专硕考研经验贴
- 提高计算机CPU运行速度,提高cpu运行速度的方法
- 结构图(SC)中的模块类型
- Orinda无线ap
- 解决Git push提交时Permission denied(publickey).Could not read from remote...的问题
- revit二开之过滤族(Family)
- 笔记本换固态硬盘-华硕K555L
热门文章
- PVNet(6D姿态估计)
- Building a Better Vocabulary: Lecture 1 Five Principles for Learning Vocabulary
- 190302每日一句
- unity数组或链表需要空间很大赋值与调用
- Atitit 2017年的技术趋势与未来的大技术趋势 1. 2017年的技术趋势	2 1.1. Web not native	2 1.2. 更加移动优先 ,,more spa	3 1.3. Ar
- Atitit 查看目录与分区空间占用原理 查看目录空间就是查看所在分区空间的占用 [root@lenovo ~]# df -h /elk 文件系统 容量 已用 可用
- Atitit 遍历 与循环模式大总结 目录 1.1. 遍历的对象 数组 或对象 或对象数组	1 2. 遍历的概念	2 2.1. 祖先后代同胞 过滤	2 3. 常见的遍历四种方式	2 3.1.
- atitit 业务 触发器原理. 与事件原理 docx
- paip.手机ROOT过程总结
- 酒后谈IT,那些术语大妈都能秒懂!