机器学习 深度学习 ai_人工智能,机器学习和深度学习。 真正的区别是什么?...
机器学习 深度学习 ai
The twenty-first century brought tremendous technological advancement that we could not dream about a couple of decades earlier. Today, it can be found that people benefit from Google’s AI-controlled predictions, Ridesharing apps such as Uber and Lyft, as well as commercial flights with an AI autopilot that uses everyday music recommender systems to involve artificial intelligence, Google maps, and many more apps are powered with AI. However, there is still a misunderstanding between artificial intelligence, machine learning, and deep learning. One of Google’s popular queries reads: “is machine learning and artificial intelligence the same thing?” or “is artificial intelligence a subset of machine learning?”
二十一世纪带来了巨大的技术进步,这是几十年前我们无法想象的。 如今,人们可以从Google的AI控制的预测,Uber和Lyft之类的Ridesharing应用程序以及带有AI自动驾驶仪的商业飞行中受益,这些AI使用日常音乐推荐系统来参与人工智能,Google地图等应用程序都支持AI。 但是,人工智能,机器学习和深度学习之间仍然存在误解。 Google最受欢迎的查询之一是:“机器学习和人工智能是一回事吗?” 或“人工智能是机器学习的子集吗?”
AI, machine learning, and deep learning are interchangeable and easily confusing, so begin with a brief intro about them.
人工智能,机器学习和深度学习是可互换的,并且容易造成混淆,因此首先简要介绍一下它们。
Artificial Intelligence: Artificial intelligence, also called machine intelligence, can be understood by an intelligence, unlike the natural intelligence shown by humans and animals, which is demonstrated by machines. It looks at ways of designing intelligent devices and systems that can address problems creatively that are often treated as a human prerogative. Thus, AI means that a machine somehow imitates human behavior.
人工智能:人工智能(也称为机器智能)可以通过一种智力来理解,而人类和动物所表现出的自然智能则是通过机器来证明的。 它着眼于设计智能设备和系统的方法,这些设备和系统可以创造性地解决通常被视为人的特权的问题。 因此,AI意味着机器以某种方式模仿了人类的行为。
Machine Learning: Machine learning is an AI subset and consists of techniques that enable computers to recognize data and supply AI applications. Different algorithms (e.g., neural networks) contribute to problem resolution in ML.
机器学习:机器学习是AI的子集,包含使计算机能够识别数据并提供AI应用程序的技术。 不同的算法(例如神经网络)有助于ML解决问题。
Deep Learning: Deep learning, often called deep neural learning or deep neural network, is a subset of machine learning that uses neural networks to evaluate various factors with a similar framework to a human neural system. It has networks that can learn from unstructured or unlabeled data without supervision.
深度学习:深度学习(通常称为深度神经学习或深度神经网络)是机器学习的一个子集,它使用神经网络来评估各种因素,并具有与人类神经系统相似的框架。 它的网络可以在无监督的情况下从非结构化或未标记的数据中学习。
人工智能 (ARTIFICIAL INTELLIGENCE)
Let’s dive into the branches of Artificial Intelligence:
让我们深入研究人工智能的分支:
AI systems are categorized by their ability to replicate human characteristics, their technology applications, their applications in the real world, and mind theory, which will be further discussed below.
人工智能系统按其复制人类特征的能力,其技术应用,其在现实世界中的应用和思维理论进行分类,这些将在下面进一步讨论。
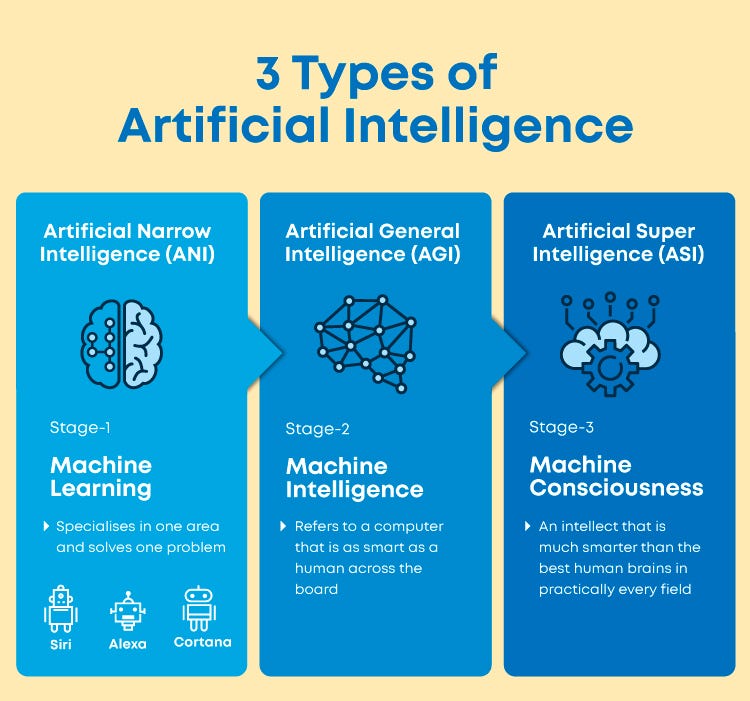
Artificial narrow intelligence (ANI), which has a narrow range of abilities.
人工窄智能 (ANI),具有窄范围的能力。
Artificial general intelligence (AGI), which is on par with human capabilities.
人工智慧 (AGI),与人类能力相当。
Artificial superintelligence (ASI), which is more capable than actual human intelligence.
人工超智能 (ASI),比实际的人类智能更强大。
窄/弱AI (Narrow/Weak AI)
Artificial narrow intelligence (ANI), also referred to as weak AI or narrow AI, is the only type of artificial intelligence we have successfully realized. Narrow AI is aim-oriented to perform different tasks — i.e., face recognition, speech recognition/voice assistants, automobile driving, and internet search — and is smart when carrying out a particular task.
人工狭窄人工智能(ANI),也称为弱AI或狭窄AI,是我们成功实现的唯一人工智能类型。 Narrow AI具有针对性,可以执行不同的任务(例如,面部识别,语音识别/语音助手,汽车驾驶和互联网搜索),并且在执行特定任务时非常聪明。
You may have learned of Deep Blue, the first machine in chess to beat a human. Not just any human — in 1996 Garry Kasparov. Deep Blue can produce about 200 million chess positions per second and analyze them. In the whole scenario, some did not readily call it AI in its entirety, while others thought that it was one of the first examples of weak AI.
您可能已经了解了深蓝,这是国际象棋中第一个击败人类的机器。 不只是人类-1996年,加里·卡斯帕罗夫(Garry Kasparov)。 深蓝每秒可以产生约2亿个国际象棋位置,并对其进行分析。 在整个场景中,有些人并没有整体上将其称为AI,而另一些人则认为这是弱AI的首例。
强大的AI /深度AI (Strong AI / Deep AI)
General Intelligence (AGI) is a concept of a computer that imitates human intelligence and behaviors, with its ability to learn and use its intelligence to solve any problem, which is also referred to as a Strong AI or deep AI. AGI can, in any given situation, think, understand, and behave in a manner that can be no different from that of a human being.
通用情报(AGI)是一种计算机的概念,该计算机模仿人类的情报和行为,具有学习和使用其情报来解决任何问题的能力,这也称为“强AI”或“深度AI”。 在任何给定的情况下,AGI都能以与人类相同的方式思考,理解和表现。
That is where robots can become human-like in the future. They decide themselves and learn without human intervention. They learn. They are not only able to solve intellectual problems but feelings.
这就是将来机器人可以变得像人一样的地方。 他们决定自己,无需人工干预就能学习。 他们学习了。 他们不仅能够解决智力问题,而且能够解决感情问题。
When one sees the human brain as the model of the creation of general intelligence, the immense challenge of achieving strong AI is not surprising. Scientists fail to reproduce the essential functions of sight and motion without a solid understanding of the human brain’s features.
当人们将人类的大脑视为创造通用智能的典范时,实现强大的AI的巨大挑战就不足为奇了。 如果不对人脑的功能有深入的了解,科学家将无法再现视觉和运动的基本功能。
超级智能 (Superintelligence)
Superintelligence is the conceptual AI that does not merely mimic or recognize human intelligence and behavior; ASI means that computers are self-conscious and outperform human ability and knowledge.
超级智能是概念性的AI,它不仅模仿或识别人类的智力和行为; ASI意味着计算机具有自我意识,并且胜过人类的能力和知识。
It is the material that everyone wants to learn about AI. Machines, long before humans. Articulate, articulate, imaginative, and outstanding professional competence. Its purpose is to either enhance or kill the lives of human beings.
这是每个人都想学习有关AI的材料。 机器早于人类。 表达,表达,想象力和杰出的专业能力。 其目的是改善或杀死人类的生命。
It may seem enticing to have such powerful tools at our fingertips, but there are many unknown implications for the idea itself. If super-intelligent and self-conscious beings were to be created, they would be able to have concepts like autonomy. It is mere speculation that will impact humanity, our future, and our way of life.
唾手可得的如此强大的工具似乎很诱人,但是对于这个想法本身有许多未知的含义。 如果要创造超智能和自我意识的生物,他们将能够拥有诸如自治的概念。 仅仅是推测会影响人类,我们的未来和我们的生活方式。
The word AI says nothing about solving these issues. Include rules-based or expert systems; there are many different techniques. In the 1980s, one group of methods became more common: machine learning.
AI一词并没有说明解决这些问题。 包括基于规则的系统或专家系统; 有很多不同的技术。 在1980年代,一组方法变得更加普遍:机器学习。
机器学习 (MACHINE LEARNING)
What exactly is Machine Learning?With new computer technologies, today’s machine learning is not like machine learning from the past. It was born from the identification of patterns and the idea that computers would learn without programming themselves for specific tasks; scientists interested in artificial intelligence wanted to see if computers could learn from data.
机器学习到底是什么? 借助新的计算机技术,当今的机器学习已不再像过去的机器学习。 它源于模式的识别以及计算机无需为特定任务编写程序即可学习的思想。 对人工智能感兴趣的科学家想看看计算机是否可以从数据中学习。
The early researchers found that problems were many more difficult because the old techniques used for AI were not compatible with those problems. Hard-coded algorithms or fixed, rule-based systems didn’t work very well for things like image recognition or text-based extraction.
早期的研究人员发现问题要困难得多,因为用于AI的旧技术与这些问题不兼容。 硬编码算法或固定的基于规则的系统在诸如图像识别或基于文本的提取之类的系统中效果不佳。
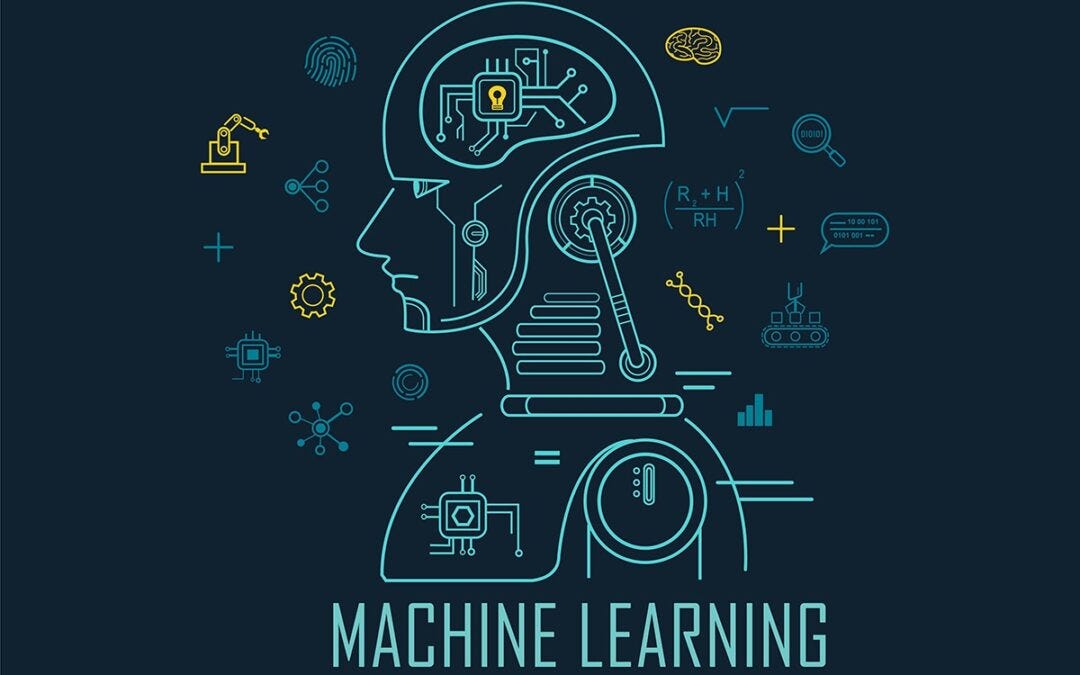
“The solution proved not only to mimic human behavior (AI) and to imitate the way people learn.”
“事实证明,该解决方案不仅可以模仿人类行为(AI)并模仿人们的学习方式。”
Consider how you have learned to read. Before you picked up your first book, you did not sit and learn orthotics and grammar. You read basic books, which over time, became more complicated. You learned the laws of orthography and grammar (and exceptions) from your lecture. In other words, you have processed and learned a lot of information.
考虑一下您如何学习阅读。 在您拿起第一本书之前,您没有坐下来学习矫正和语法。 您阅读基础书籍,随着时间的流逝,基础书籍变得越来越复杂。 您从您的讲座中学到了拼字法和语法法(和例外)。 换句话说,您已经处理并学习了很多信息。
This is the idea of machine learning precisely. Feed a lot of data into an algorithm (unlike the brain) and let things figure out. Feed an algorithm a lot of financial transaction information, tell them which financial transactions are fraudulent, and make them work out what is fraudulent so that they can predict fraud in the future. Or supply your customer base information and let it know how to segment it best. Read more about machine learning here.
这就是机器学习的思想。 将大量数据输入到算法中(不同于大脑),然后让事情解决。 向算法提供大量金融交易信息,告诉他们哪些金融交易是欺诈性的,并使它们确定什么是欺诈性的,以便他们可以预测将来的欺诈行为。 或者提供您的客户群信息,并让其知道如何对其进行最好的细分。 在此处阅读有关机器学习的更多信息。
With the development of these algorithms, several problems could be dealt. But certain things people find simple (e.g., understanding of speech or writing) were still difficult for machines.
随着这些算法的发展,可以解决几个问题。 但是,人们发现某些简单的事情(例如,对语音或写作的理解)对于机器仍然很困难。
The notion that artificial neurons are the key elements of your brain was for some time to be used (neurons, bound by synapses). And software simulated neural networks began to be used for specific issues. They have been successful, and they can solve complex problems that other algorithms can not answer.
人造神经元是大脑关键要素的观念已经使用了一段时间(神经元,由突触结合)。 并且软件模拟的神经网络开始用于特定问题。 他们已经取得了成功,并且可以解决其他算法无法解决的复杂问题。
特定机器如何学习? (How can a particular machine learn?)
We will need these three components to “educate” the machine or make the computer learn:
我们将需要这三个组件来“教育”机器或使计算机学习:
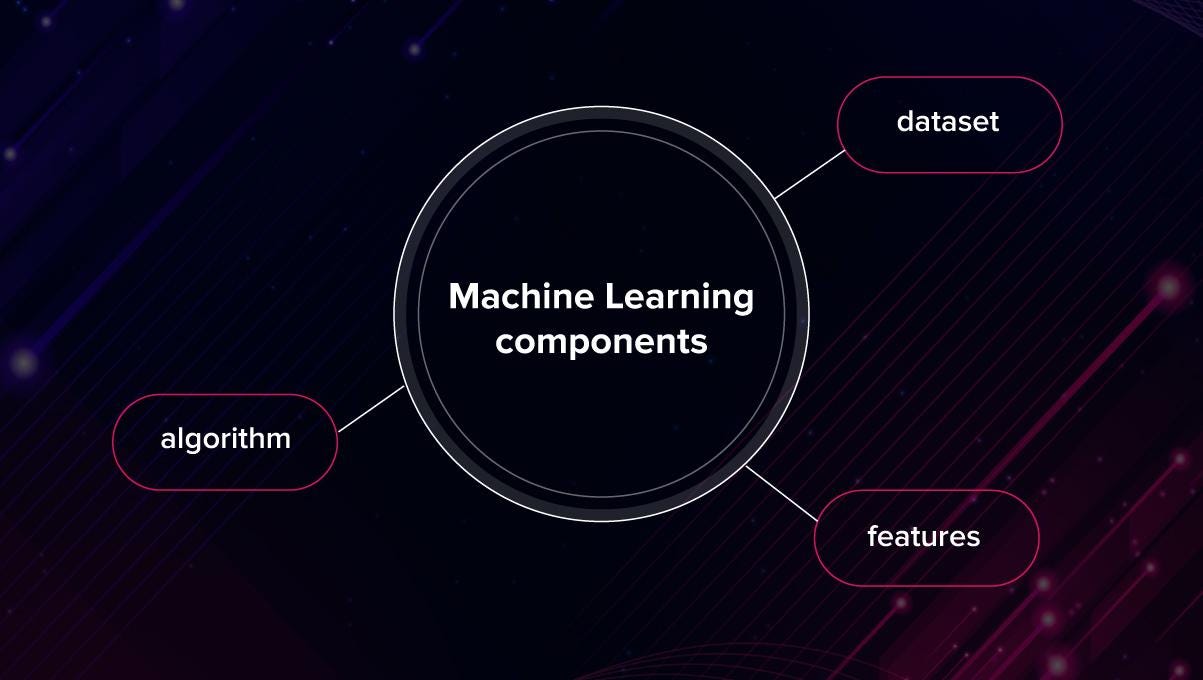
Datasets:A data set consists of collecting similar entities and values within a single body that can be viewed individually or as an entire item and structured with a data structure. Typically, building a successful dataset requires a lot of time and effort.
数据集:数据集包括在单个主体内收集相似的实体和值,这些实体和值可以单独查看,也可以作为一个整体查看,并使用数据结构进行结构化。 通常,构建成功的数据集需要大量时间和精力。
Two ways to collect data for your Model:1. Rely on open-source data2. Collect your data in the right way
收集模型数据的两种方法:1。 依靠开源数据2。 以正确的方式收集数据
Features:Features are essential data objects, which are the key to the task’s solution. They are individual measures or characteristics of a phenomenon being observed. The selection of informative, discriminatory, and independent features is crucial for effective pattern recognition, classification, and regression algorithms. They display the machine what to pay attention to.
功能:功能是必不可少的数据对象,这是任务解决方案的关键。 它们是观察到的现象的单独度量或特征。 信息性,区分性和独立性的选择对于有效的模式识别,分类和回归算法至关重要。 他们向机器显示要注意什么。
Algorithm:An algorithm is a component that allows systems to learn and develop experience automatically without being programmed explicitly. There are extensive use and description of algorithms like linear regression, deep learning, coevolutionary networks, and recommendation systems. The accuracy or speed of the results will vary depending on the algorithm.
算法:算法是允许系统自动学习和发展经验而无需进行显式编程的组件。 诸如线性回归,深度学习,协同进化网络和推荐系统之类的算法已得到广泛使用和描述。 结果的准确性或速度会因算法而异。
学习类型 (Types of Learning)
There are four types of machine learning:
机器学习有四种类型:
Supervised Learning: Training data contains optimal outcomes (also known as inductive learning). Learning is tracked in this method. Some famous examples of supervised machine learning algorithms are Linear regression for regression problems.
监督学习 :培训数据包含最佳结果(也称为归纳学习)。 通过这种方法跟踪学习。 监督机器学习算法的一些著名示例是用于回归问题的线性回归。
Unsupervised Learning: There are not the desired outputs in the training results. Clustering is an example. It is impossible to know what is and what is not good learning.
无监督学习:培训结果中没有所需的输出。 集群就是一个例子。 不可能知道什么是什么,什么不是很好的学习。
Semi-supervised Learning: A few desired outputs are included in the training data.
半监督学习:训练数据中包含一些期望的输出。
Reinforcement Learning: Rewards are given after a sequence of actions. In a given case, it is a matter of taking appropriate steps to maximize compensation. It is the most ambitious method of learning in AI.
强化学习:奖励是在一系列行动之后给出的。 在给定的情况下,必须采取适当的步骤来最大化补偿。 这是AI中最雄心勃勃的学习方法。
深度学习 (DEEP LEARNING)
Deep learning is a subset of machine learning in artificial intelligence (AI) with networks capable of learning unsupervised from unstructured or unlabeled data. Also known as deep neural learning or deep neural network. Deep learning algorithms use hierarchical multi-level neural networks, in which the abstraction level slowly increases through non-linear input data transformations.
深度学习是人工智能(AI)中机器学习的一个子集,其网络能够从非结构化或未标记的数据中不受监督地学习。 也称为深度神经学习或深度神经网络。 深度学习算法使用分层的多级神经网络,其中抽象级别通过非线性输入数据转换缓慢增加。
它实际上如何运作? (How does it actually work?)
The digital era, which contributed to the proliferation of data in all forms, has been transformed into profound learning from all the corners of the world. Such data, also known as big data, come from outlets such as social media, Internet search engines, electronic commerce, and online videos. This massive amount of data can be easily accessed and exchanged through fintech applications, such as cloud computing.
推动各种形式的数据扩散的数字时代已经转变为世界各个角落的深刻学习。 这些数据也称为大数据,来自社交媒体,互联网搜索引擎,电子商务和在线视频等渠道。 通过金融技术应用程序(例如云计算)可以轻松访问和交换大量数据。
The typically unstructured data, however, is so large that it may take decades for people to grasp it and extract relevant information. Companies understand the enormous potential that this wealth of information can bring about and are increasingly adapting for automated support to AI systems.
但是,通常非结构化的数据是如此之大,以至于人们可能需要数十年才能掌握它并提取相关信息。 公司了解大量信息可以带来的巨大潜力,并且越来越适应于对AI系统的自动支持。
The specific number of neurons is called bias. In addition to the weights, this bias is added to the weighted amount of inputs to the neuron. When the neuron is activated, the outcome of the task decides. Each activated neuron passes the following layers. It goes on to the last second sheet. The final layer generating program outputs is the output layer in an artificial neural network.
神经元的具体数量称为偏见。 除了权重,此偏差还会添加到神经元输入的加权数量上。 当神经元被激活时,任务的结果将决定。 每个激活的神经元都经过以下层。 继续到最后第二张纸。 生成程序输出的最后一层是人工神经网络中的输出层。
Deep learning algorithms are very hype today, but a boundary between deep and not as deep algorithms.
如今,深度学习算法非常受炒作,但深度算法与非深度算法之间存在界限。
翻译自: https://medium.com/swlh/artificial-intelligence-machine-learning-and-deep-learning-whats-the-real-difference-94fe7e528097
机器学习 深度学习 ai
http://www.taodudu.cc/news/show-1874127.html
相关文章:
- 锁 公平 非公平_推荐引擎也需要公平!
- 创建dqn的深度神经网络_深度Q网络(DQN)-II
- kafka topic:1_Topic️主题建模:超越令牌输出
- dask 于数据分析_利用Dask ML框架进行欺诈检测-端到端数据分析
- x射线计算机断层成像_医疗保健中的深度学习-X射线成像(第4部分-类不平衡问题)...
- r-cnn 行人检测_了解用于对象检测的快速R-CNN和快速R-CNN。
- 语义分割空间上下文关系_多尺度空间注意的语义分割
- 自我监督学习和无监督学习_弱和自我监督的学习-第2部分
- 深度之眼 alexnet_AlexNet带给了深度学习的世界
- ai生成图片是什么技术_什么是生成型AI?
- ai人工智能可以干什么_我们可以使人工智能更具道德性吗?
- pong_计算机视觉与终极Pong AI
- linkedin爬虫_这些框架帮助LinkedIn大规模构建了机器学习
- 词嵌入生成词向量_使用词嵌入创建诗生成器
- 端到端车道线检测_如何使用Yolov5创建端到端对象检测器?
- 深度学习 检测异常_深度学习用于异常检测:全面调查
- 自我监督学习和无监督学习_弱和自我监督的学习-第3部分
- 聊天工具机器人开发_聊天机器人-精致的交流工具? 还是您的客户服务团队不可或缺的成员?...
- 自我监督学习和无监督学习_弱和自我监督的学习-第4部分
- ai星际探索 爪子_探索AI地牢
- 循环神经网络 递归神经网络_递归神经网络-第5部分
- 用于小儿肺炎检测的无代码AI
- 建筑业建筑业大数据行业现状_建筑—第2部分
- 脸部识别算法_面部识别技术是种族主义者吗? 先进算法的解释
- ai人工智能对话了_产品制造商如何缓解对话式AI中的偏见
- 深度神经网络 轻量化_正则化对深度神经网络的影响
- dbscan js 实现_DBSCAN在PySpark上的实现
- 深度学习行人检测简介_深度学习简介
- ai初创企业商业化落地_初创企业需要问的三个关于人工智能的问题
- scikit keras_使用Scikit-Learn,Scikit-Opt和Keras进行超参数优化
机器学习 深度学习 ai_人工智能,机器学习和深度学习。 真正的区别是什么?...相关推荐
- 机器学习 深度学习 ai_人工智能,机器学习,深度学习-特征和差异
机器学习 深度学习 ai Artificial Intelligence (AI) will and is currently taking over an important role in our ...
- 深度学习(一): 人工智能-机器学习-深度学习的区别
人工智能-机器学习-深度学习 他们之间是有区别的 先来一张图做一下解释 从发展历史上来看 AI:让机器展现出人类智力 回到1956年夏天,在当时的会议上,AI先驱的梦想是建造一台复杂的机器(让当时刚出 ...
- 损失能收敛到0么 深度学习_人工智能-Tensorflow进行深度学习的一些损失函数的可视化...
TensorFlow目前是数值计算的最佳开源库,它使机器学习更快更容易.在这篇文章中,您将学习机器学习中使用的一些损失函数.损失函数在机器学习领域非常重要.它们用作测量模型预测输出Y_out与grou ...
- TensorFlow深度学习算法原理与编程实战 人工智能机器学习技术丛书
作者:蒋子阳 著 出版社:中国水利水电出版社 品牌:智博尚书 出版时间:2019-01-01 TensorFlow深度学习算法原理与编程实战 人工智能机器学习技术丛书 ISBN:97875170682 ...
- 机器学习中的数学 人工智能深度学习技术丛书
作者:孙博 著 出版社:中国水利水电出版社 品牌:智博尚书 出版时间:2019-11-01 机器学习中的数学 人工智能深度学习技术丛书 ISBN:9787517077190
- 图解人工智能机器学习深度学习的关系和区别
图解人工智能机器学习深度学习的关系和区别,先直观看下图的关系: AI(Artificial Intelligence.人工智能).机器学习(machine learning).深度学习(Deep le ...
- 自学机器学习、深度学习、人工智能的学习资源集合
本人美国top50本科统计就读,想当初我的机器学习和深度学习就是靠自学入门的.现在行业中对于人工智能自学这一方法众说纷纭,也有很多人质疑那些通过自学获得的技术及实力.就我个人而言,我体验过各种各样的课 ...
- 自学机器学习、深度学习、人工智能学习资源推大聚合
想要解决如何自学机器学习.深度学习和人工智能这一问题,首先要了解三个概念以及它们之间的关系. 人工智能:人工智能英文缩写为AI,它是研究.开发用于模拟.延伸和扩展人的智能的理论.方法.技术及应用系统的 ...
- 人工智能-机器学习-深度学习的区别与联系
AI,机器学习,深度学习,这些术语使很多人感到困惑.如果您也是其中之一,那么人工智能-机器学习-深度学习的区别与联系– AI vs Machine Learning vs Deep Learning绝 ...
- (不定期更新)《人工智能AI资讯》(Yanlz+VR云游戏+Unity+SteamVR+云技术+5G+AI+人工智能+AlphaGo+神经网络+深度学习+机器学习+自动驾驶+图像识别+立钻哥哥+==)
<人工智能AI资讯> 人工智能(Artificial Intelligence) 人工智能简称AI,是研究.开发用于模拟.延伸和扩展人的智能的理论.方法.技术及应用系统的一门新的技术科学. ...
最新文章
- Spark Shuffle原理解析
- Thinkphp5 还有这种操作?
- HTML表格的简单使用1
- 一次解决DB2接口文件到Oracle无法导入问题的经历
- COGS 2687 讨厌整除的小明
- 有向图的深度优先遍历
- 【PhotoScan精品教程】PhotoScan简介、安装教程(附PhotoScan1.4.5安装包下载)
- 谈下论坛和商城的开发项目
- 【Unity】跨场景音乐资源不间断播放(可控音量)
- 删除主键索引 oracle,删除主键无法删除对应索引问题 drop constraint
- CDOJ1323柱爷的下凡
- 【网络】inux流量控制器TC(Traffic Control)
- ConnectBot的使用
- Pycharm下载地址、汉化方法与常用快捷键
- AI Earth ——开发者模式案例5:鄱阳湖水体区域识别
- 使用describe()查看catering_sale.xls数据的基本情况,通过箱式图检测出catering_sale.xls中的异常值
- 微信小程序基础(二)
- 三维空间直线与平面交点
- python网页爬虫+简单的数据分析
- 成都拓嘉辰丰:拼多多库存不足会导致排名下降吗
热门文章
- 20200705每日一句
- 每日一句20200104
- Atiitt 可视化 报表 图表之道 attilax著 Atitit.可视化与报表原理与概论 1. 信息可视化	1 2. Gui可视化	2 2.1. atitit 知识的可视化.docx	2
- Atitit java jsp 新的tag技术
- paip.输出内容替换在Apache 过滤器filter的设置
- paip.手写OCR识别方法总结--基于验证码识别... 1
- paip.取回密码功能总结
- qq安全使用NTFS权限设置法
- 硬盘分区后的逻辑结构
- 使用C#的后端Web API:循序渐进教程