web与ai相结合成为趋势_将AI和行为科学相结合可以改变体验
web与ai相结合成为趋势
I’m part of a purpose-driven organization with a core philosophy that empathy is at the center of effective, meaningful design. In an environment where we put a high value on understanding a user’s challenges, it may be surprising that we believe artificial intelligence (AI) could play a significant role in designing a user experience that makes people feel seen, cared for, and yes, the recipients of empathy. While machines can’t truly empathize, they are designed by human beings who can. Designers who bring empathy to the creation of technology can ensure that inserting AI into the user experience does not take away the vital human connection essential to great outcomes.
我是一个以目标为导向的组织的一员,其核心理念是移情是有效,有意义的设计的核心。 在我们高度重视理解用户挑战的环境中,令人惊讶的是,我们相信人工智能(AI)可以在设计使人们感到被关注,关心的用户体验中发挥重要作用,是的。移情的接收者。 虽然机器不能真正移情,但它们是由有能力的人设计的。 重视技术创造的设计人员可以确保将AI插入用户体验中不会夺走对于实现出色成果至关重要的至关重要的人际关系。
“Empathy” may seem to be a fuzzy concept. It is about putting yourself into the user’s shoes and trying to deeply understand their perspective. We find that using a behavior science lens to direct empathy can increase its design power. My team’s approach to experience design focuses on defining desired behaviors, and then understanding what might motivate our users — or not! — to do those behaviors. We look at the context in which people live and take action, and how the environment facilitates or prevents behaviors. Then we design to take advantage of motivating factors and overcome demotivating ones. This approach gives us the ability to make a meaningful connection with the intended audience whether that’s a chronically ill patient, a banking customer, or a retail store employee.
“ Empathy”似乎是一个模糊的概念。 这是关于使自己陷入使用者的鞋子并试图深入了解他们的观点。 我们发现,使用行为科学的视角来引导同理心可以提高其设计能力。 我的团队的体验设计方法着重于定义所需的行为,然后了解可能会激发我们的用户的动机–或没有! -做那些行为。 我们着眼于人们生活和采取行动的环境,以及环境如何促进或防止行为。 然后我们设计以利用激励因素并克服不利因素。 这种方法使我们能够与目标受众建立有意义的联系,无论是长期病患者,银行客户还是零售商店的员工。
AI technology has become both more sophisticated and more common in recent years. As it becomes a bigger part of the design toolkit, it’s critical to consider how AI contributes to the overall user experience. It can be tempting to incorporate AI for its advantages — its scalability, its flexibility, and its promise of enhanced outcomes. But if we do so without considering the larger user context and motivation, we run the risk of missing the mark. Our behavior science lens, rooted in empathy, can help us identify where and how AI can be incorporated into the experiences we design so that they are ultimately successful for both business and people.
近年来,人工智能技术变得越来越复杂 , 越来越普遍 。 随着它成为设计工具包的重要组成部分,考虑AI如何为整体用户体验做出贡献至关重要。 可能会吸引AI来利用AI的优势-它的可伸缩性,灵活性和增强结果的希望。 但是,如果我们这样做时没有考虑更大的用户背景和动机,那么就有冒失商标的风险。 植根于同理心的行为科学视角可以帮助我们确定在何处以及如何将AI整合到我们设计的体验中,从而最终为企业和个人带来成功。
了解动机 (Understanding Motivation)
Our behavior science lens centers on understanding motivation. To do that, we primarily draw from self-determination theory. Over 40 years of research support self-determination theory’s core tenets: The quality, or source, of people’s motivation matters deeply for its effect on behavior, and supporting people’s basic psychological needs is the key to fostering high quality motivation. The good news is that much of the research on self-determination theory looks specifically at how to design products and experiences that people find engaging.
我们的行为科学镜头以理解动机为中心。 为此,我们主要借鉴自决理论 。 超过40年的研究支持自决理论的核心宗旨:人的动机的质量或来源对行为的影响深远,而支持人的基本心理需求则是培养高质量动机的关键。 好消息是,许多关于自决理论的研究都专门针对如何设计人们感兴趣的产品和体验。
First, let’s consider motivational quality. The source of people’s motivation matter. Some forms of motivation are externally imposed. These forms of controlled motivation look like the doctor telling you to eat healthier or your parents lecturing you about saving for retirement. You might feel pressured to try a certain behavior (eating better, saving money), but it’s difficult to maintain that behavior when other competing demands arise (pizza night with friends, buying expensive new shoes). In general, controlled motivation can kick-start a behavior, but isn’t enough to sustain it over time.
首先,让我们考虑激励质量 。 人们的动力源于物质。 某些形式的动机是外部施加的。 这些形式的控制动机看起来像是医生告诉您要饮食健康或者您的父母为您讲退休储蓄。 您可能会感到尝试某种行为的压力(饮食更好,省钱),但是当其他竞争需求出现时(与朋友共进比萨之夜,购买昂贵的新鞋),很难维持这种行为。 一般而言,控制动机可以启动行为,但不足以随着时间的流逝维持它。
Contrast with autonomous motivation. These are more internally-generated sources of motivation. An autonomously motivated person can look to their own personal goals, values, or sense of self as a way to drive behavior change. Behaviors become a means to accomplish those goals, live into the values, or be the person they imagine themselves to be. Eating better or saving money become much easier when they’re in service of something like a desire to run around with the grandkids or a dream of retiring to a beach community. When it comes to experience design, we look for ways to tap into autonomous forms of motivation, which often requires getting to know our user. (Good news here — AI can help!)
与自主动机相反。 这些是更多内部产生的动力来源。 一个有上进心的人可以将自己的个人目标,价值观或自我意识作为推动行为改变的一种方式。 行为成为实现这些目标,实现价值观或成为自己想象中的人的一种手段。 当他们服务于与孙子孙女四处奔跑的愿望或退休于海滩社区的梦想时,进食更好或省钱变得容易得多。 当涉及到体验设计时,我们寻求利用动机的自主形式的方法,这通常需要了解我们的用户。 (这里有个好消息-人工智能可以提供帮助!)

Once experience designers have identified their users’ personally meaningful motivational sources, they can begin to craft experiences that support basic psychological needs. These include autonomy (making meaningful choices), competence (learning and growing), and relatedness (being connected to something bigger than oneself). Effective design includes striking the right balance of making things easy for users and making them effortful, so they can feel a sense of choice, growth, and connection without becoming frustrated or overwhelmed. This is another area where AI has the potential to support good design.
体验设计师一旦确定了用户个人有意义的动机来源,就可以开始制作满足基本心理需求的体验。 这些包括自主权(做出有意义的选择),能力(学习和成长)和亲密关系(与比自己更大的事物相关联)。 有效的设计包括在使用户易于操作和使用户轻松工作之间取得适当的平衡,使他们可以感到选择,成长和联系的感觉而不会感到沮丧或不知所措。 这是人工智能有潜力支持良好设计的另一个领域。
了解情境中的经验 (Understanding Experience in Context)
Of course, motivation does not exist in a vacuum. It always takes a behavior as an object — people are motivated to do something — and it plays out in a real-world context that can present obstacles, choice points, social pressures, competing demands, and all manner of factors that influence whether the behavior is actually performed and how. Experience designers dig deep on those contextual factors in order to improve the odds that the desired behaviors happen consistently.
当然,动力并不存在于真空中。 它始终以行为为对象-人们有动力去做某事-并且它在现实世界中发挥作用,可能会出现障碍,选择点,社会压力,竞争需求以及影响行为是否发生的各种因素实际执行以及如何执行。 经验丰富的设计师对这些上下文因素进行了深入研究,以提高期望的行为持续发生的几率。
We do a lot of formative research on our projects to really understand the specific context in which our specific users might be doing a specific behavior. We all know from being human that context matters a lot. The person who leaves messy dishes on the counter at home may keep a pristine desk at work. We can’t make assumptions about that person’s cleanliness writ large; we have to understand the context and what makes their behavior different in different environments. This is where AI sometimes fails, because the people designing it don’t consider the context that influences people’s behavior.
我们对项目进行了大量的形成性研究,以真正了解特定用户可能在其中进行特定行为的特定上下文。 我们都是人类,因此背景非常重要。 在家里的柜台上留下乱七八糟的菜的人可以在工作时保持原始的办公桌。 我们不能对那个人的清洁度做出大的假设。 我们必须了解上下文,以及它们在不同环境中的行为如何不同。 这就是AI有时会失败的地方,因为设计它的人没有考虑会影响人们行为的环境。
There are many well-documented failures of AI. There sometimes seems to be a perception that AI is objectively correct because it’s based on algorithms and data, but remember that human beings develop those algorithms and choose that data. When AI fails, it‘s very rarely a technological issue. It tends to be errors on the design side: Failed algorithms, poorly chosen data (that may codify historical patterns of discrimination or other biases), or a lack of understanding of the user’s behaviors in context.
有许多 有 据可查的AI 失败案例。 有时候似乎有人认为AI是客观正确的,因为它是基于算法和数据的,但是请记住,人类会开发这些算法并选择数据。 当AI失败时,很少出现技术问题。 在设计方面,这往往是错误:算法失败,数据选择不当(可能会编入辨别力或其他偏见的历史模式)或对用户在上下文中的行为缺乏了解。
Let’s take the data piece: AI is usually trained using existing or synthetic data sets. Designers need to be scrupulous about selecting training data that accurately describes their users’ situations, or the resulting algorithms won’t be right for that audience. In the benign version of that error, AI is just off and ineffective. In more pernicious versions, it doubles down on harmful biases. For example, women have historically been excluded from scientific research on heart disease. Using a data set that includes only men to train an AI heart health program would likely be much less effective for women users. I’ve included some suggested readings at the end of the article that dig into many more examples of these types of errors and how designers can be alert to them.
让我们来看看数据:AI通常是使用现有或合成数据集进行训练的。 设计师必须谨慎选择能够准确描述其用户情况的培训数据,否则生成的算法将不适用于该受众。 在该错误的良性版本中,AI处于关闭状态且无效。 在更有害的版本中,它会加倍有害偏见。 例如,历史上一直将妇女排除在心脏病研究之外。 使用仅包含男性的数据集来培训AI心脏健康计划,对女性用户而言可能没有那么有效。 我在文章的末尾提供了一些建议的阅读材料,以深入探讨此类错误的更多示例以及设计人员如何对其发出警报。
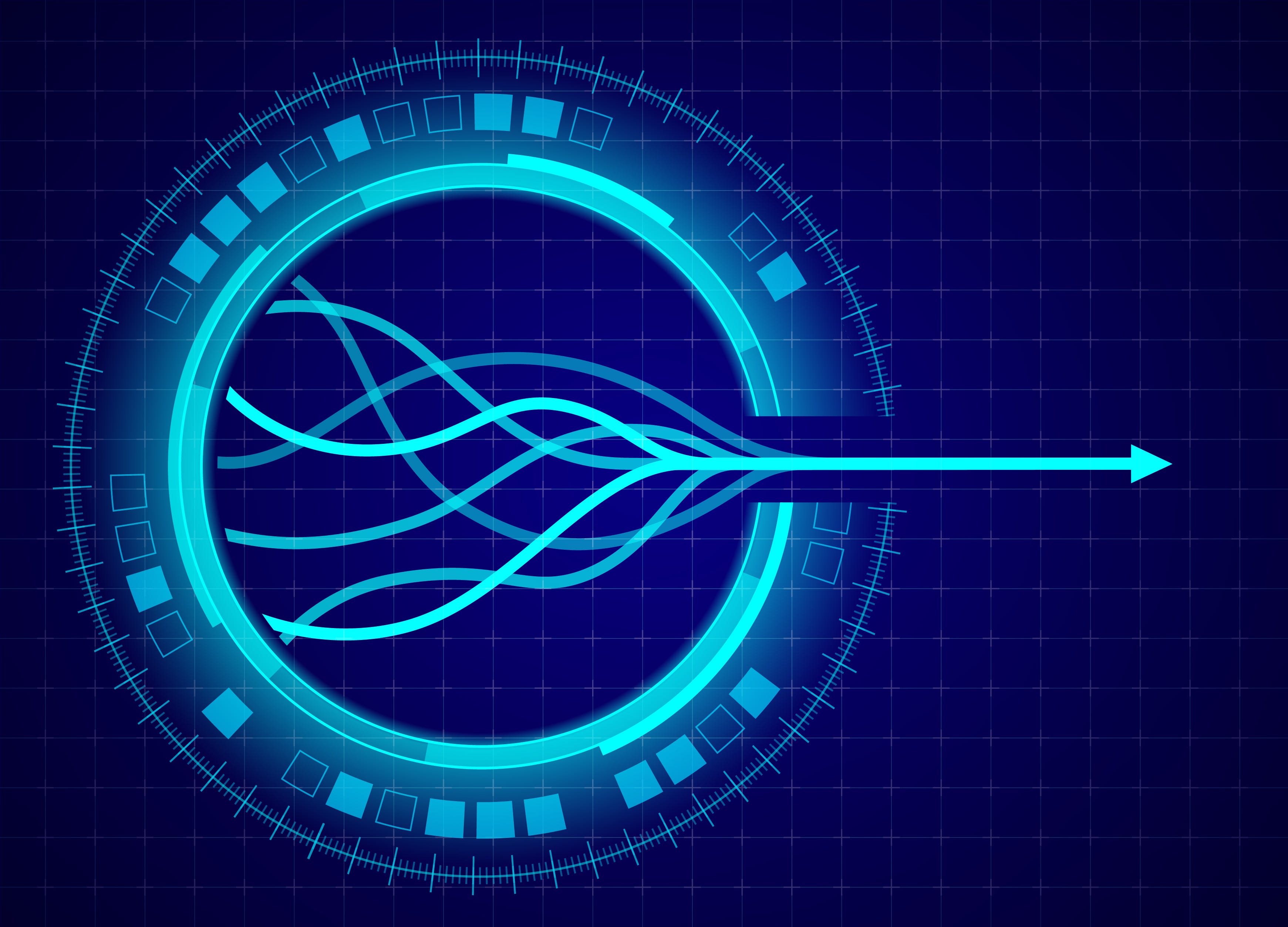
We’re traveling down a two-way street: We’re not just training people to use technology, we’re training technology to relate to people. It’s critical to use the right training materials. This may mean investing in deep research at the outset of design, but it will pay off in terms of efficacy and accuracy. Going forward, getting this human-centric design right is the biggest factor in the success or failure of AI-driven transformations.
我们正沿着一条双向的街道旅行:我们不仅在培训人们使用技术,而且还在培训与人们相关的技术。 使用正确的培训材料至关重要。 这可能意味着在设计之初就投资于深度研究,但在功效和准确性方面会有所回报。 展望未来,正确实现以人为本的设计是AI驱动的转换成败的最大因素。
算法规避 (Algorithm Aversion)
As AI has become integrated into more experiences, researchers have identified a persistent issue known as “algorithm aversion.” Basically, people are wary of having an algorithm make decisions for them. Often people feel that it’s not possible for a machine to understand them the same way a human could. As a result, they interpret AI-generated recommendations as impersonal, and not right for them. Even when evidence suggests an algorithm delivers greater accuracy than a human expert, people tend to prefer having human involvement.
随着AI已融入更多经验中,研究人员已经发现了一个持续存在的问题,称为“算法厌恶”。 基本上,人们会警惕让算法为他们做决定。 人们通常会觉得机器不可能像人类一样理解它们。 结果,他们将AI生成的建议解释为非个人推荐,而不适合他们。 即使有证据表明算法比人类专家提供更高的准确性, 人们也倾向于选择让人类参与 。
That’s one reason why we don’t necessarily look to design technology-only solutions. There can be enormous power in blending a human touch with technology when AI is involved. Live experts can support AI-generated recommendations by providing rationales, tailoring recommendations, or making adjustments based on user feedback. They can reinforce caring and warmth, and bring human empathy into the experience to build trust with the user. Providing emotional connection and caring is important to a successful experience. (It goes back, in part, to that basic psychological need of relatedness.)
这就是为什么我们不一定要设计仅技术解决方案的原因之一。 当涉及AI时,将人的触觉与技术融合在一起可能具有巨大的力量。 实时专家可以通过提供理由,定制建议或基于用户反馈进行调整来支持AI生成的建议。 他们可以加强关怀和温暖,并在体验中引入人性共鸣,与用户建立信任。 提供情感联系和关怀对于成功的体验至关重要。 (它可以部分追溯到相关性的基本心理需求。)
One example where Mad*Pow worked to overcome algorithm aversion is in developing a connected health solution called ImagineCare. Developed in partnership with Dartmouth-Hitchcock Medical Center, ImagineCare enables patients with specific chronic health conditions to have 24/7 monitoring and support. The behavioral goals of the program were to help patients better manage their conditions (with specific behaviors for each condition) and for clinical staff to be able to identify imminent health crises and intervene before emergency care is needed. Ultimately, the hope was that ImagineCare would improve patient outcomes and lower medical costs.
Mad * Pow致力于克服算法厌恶的一个例子是开发一种称为ImagineCare的互联健康解决方案。 与达特茅斯-希区柯克医学中心合作开发的ImagineCare使患有特定慢性疾病的患者能够进行24/7全天候监测和支持。 该计划的行为目标是帮助患者更好地管理自己的病情(针对每种病情采取特定的行为),并让临床人员能够识别迫在眉睫的健康危机并在需要紧急护理之前进行干预。 最终,人们希望ImagineCare能够改善患者预后并降低医疗成本。
The project required a simultaneous focus on the high-tech and analog experiences. Patients received an array of internet-enabled devices to help monitor their health depending on their specific diagnoses. Someone with congestive heart failure might get a Bluetooth-enabled scale, while someone with COPD received a connected inhaler and someone with hypertension, a connected blood pressure cuff. The physical device unboxing included coaching on setting up the smartphone app on which patients could review their data and receive feedback and recommendations. Meanwhile, the data was also sent to a dashboard monitored by Dartmouth-Hitchcock nurse case managers. When the algorithm detected data that indicated a potential health crisis based on a patient’s prior history and diagnosis, the nurse case manager received an immediate alert to prompt outreach to the patient.
该项目需要同时关注高科技和模拟体验。 患者会收到一系列支持互联网的设备,以根据他们的具体诊断帮助监测他们的健康状况。 患有充血性心力衰竭的人可能会使用支持蓝牙的秤,而患有COPD的人会收到一个连接的吸入器,而患有高血压的人会连接一个血压袖带。 物理设备的拆箱包括指导安装智能手机应用程序,患者可以在该应用程序上查看其数据并接收反馈和建议。 同时,数据也被发送到由达特茅斯-希区柯克护士案例经理监视的仪表板。 当算法基于患者的既往病史和诊断检测到表明潜在健康危机的数据时,护士病例管理员会立即收到警报,以提示与患者联系。
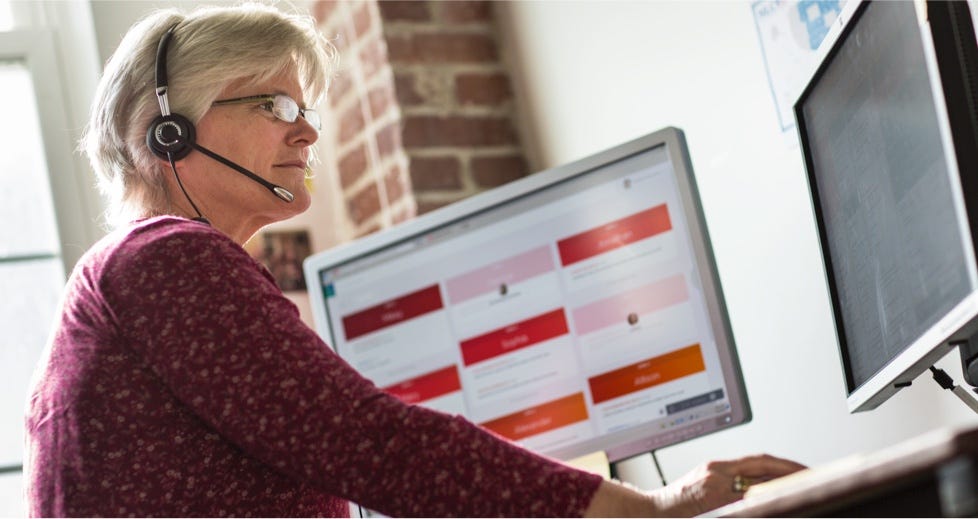
By prompting providers to make contact with patients as soon as data suggested a problem, ImagineCare was able to reduce emergency room utilization and save costs. Specifically, Dartmouth-Hitchcock saw savings of $298 per patient per month, primarily due to reduced unplanned and emergency medical visits. But what was more important in many ways was that the blend of technology and human expertise struck the right tone for patients: ImagineCare received a stunning 95% satisfaction rating from pilot participants.
通过提示提供者在数据表明存在问题时立即与患者联系,ImagineCare能够减少急诊室使用率并节省成本。 具体而言,达特茅斯-希区柯克每个人每月节省298美元,这主要是由于减少了计划外和紧急医疗就诊。 但是,在许多方面更重要的是,技术和人类专业知识的融合为患者敲响了正确的音调:ImagineCare从试点参与者那里获得了惊人的95%满意度 。
我们可以让AI变得重要 (We Can Make AI Matter)
The devil, as they say, is in the details. By using a behavior science lens to understand user needs and the context in which they’ll approach an experience, designers can leverage AI in ways that both support desired outcomes and help users feel understood. AI has the power to collect and analyze data that provides a sharper picture of a user and can facilitate a more effective experience — in healthcare, retail, or financial services. By understanding people, their motivations, and any reservations they may have about AI-driven design, designers have the opportunity to create transformational experiences that deliver outcomes that are better for business and better for people.
正如他们所说,魔鬼在细节中。 通过使用行为科学的视角来理解用户需求以及他们体验体验的环境,设计人员可以以支持期望结果并帮助用户感觉被理解的方式利用AI。 AI具有收集和分析数据的能力,这些数据可以为用户提供更清晰的图像,并可以促进在医疗保健,零售或金融服务方面的更有效的体验。 通过了解人们,他们的动机以及对AI驱动设计的任何保留,设计师有机会创造变革性的体验,从而带来对企业和人类都更好的结果。
Further Reading
进一步阅读
Criado Perez, C. (2019). Invisible Women: Exposing Data Bias in a World Designed for Men. New York: Abrams.
Criado Perez,C.(2019年)。 看不见的女人:暴露在为男人设计的世界中的数据偏见 。 纽约:艾布拉姆斯。
Eubanks, V. (2017). Automating Inequality: How High-Tech Tools Profile, Police, and Punish the Poor. New York: St. Martin’s Press.
Eubanks,V.(2017年)。 自动化不平等:高科技工具如何识别,管理和惩罚贫困者 。 纽约:圣马丁出版社。
Noble, S. U. (2018). Algorithms of Oppression: How Search Engines Reinforce Racism. New York: New York University Press.
SU,Noble(2018)。 压迫算法:搜索引擎如何增强种族主义。 纽约:纽约大学出版社。
O’Neil, C. (2016). Weapons of Math Destruction: How Big Data Increases Inequality and Threatens Democracy. New York: Broadway Books.
奥尼尔(2016)。 数学破坏武器:大数据如何加剧不平等并威胁民主。 纽约:百老汇书。
Wachter-Boettcher, S. (2017). Technically Wrong: Sexist Apps, Biased Algorithms, and Other Threats of Toxic Tech. New York: WW Norton & Company.
Wachter-Boettcher,S.(2017年)。 从技术上讲是错误的:性别歧视应用,偏见算法和有毒技术的其他威胁 。 纽约:WW Norton&Company。
翻译自: https://uxdesign.cc/combining-ai-and-behavior-science-can-transform-experience-af20b6252847
web与ai相结合成为趋势
http://www.taodudu.cc/news/show-1873898.html
相关文章:
- 递归神经网络/_递归神经网络
- Kardashev量表和AI:可能的床友
- 变异数分析_人工智能系统中分析变异的祸害
- ai时代大学生的机遇和挑战_评估AI对美术的影响:威胁或机遇
- 人工智能+智能运维解决方案_人工智能驱动的解决方案可以提升您的项目管理水平
- c语言 机器语言 汇编语言_多语言机器人新闻记者
- BrainOS —最像大脑的AI
- 赵本山 政治敏锐_每天5分钟保持敏锐的7种方法
- 面试问到处理过什么棘手问题_为什么调节人工智能如此棘手?
- python svm向量_支持向量机(SVM)及其Python实现
- 游戏世界观构建_我们如何构建技术落后的世界
- 信任的机器_您应该信任机器人吗?
- ai第二次热潮:思维的转变_基于属性的建议:科技创业公司如何使用AI来转变在线评论和建议
- 建立RoBERTa模型以发现Reddit小组的情绪
- 谷歌浏览器老是出现花_Google全新的AI平台值得您花时间吗?
- nlp gpt论文_开放AI革命性的新NLP模型GPT-3
- 语音匹配_什么是语音匹配?
- 传统量化与ai量化对比_量化AI偏差的风险
- ai策略机器人研究a50_跟上AI研究的策略
- ai人工智能 工业运用_人工智能在老年人健康中的应用
- 人工智能民主化无关紧要,数据孤岛以及如何建立一家AI公司
- 心公正白壁无瑕什么意思?_人工智能可以编写无瑕的代码后,编码会变得无用吗?
- 人工智能+社交 csdn_关于AI和社交媒体虚假信息,我们需要尽快进行三大讨论
- 标记偏见_人工智能的影响,偏见和可持续性
- gpt2 代码自动补全_如果您认为GPT-3使编码器过时,则您可能不编写代码
- 机器学习 深度学习 ai_什么是AI? 从机器学习到决策自动化
- 艺术与机器人
- 中国ai人工智能发展太快_中国的AI:开放采购和幕后玩家
- 让我们手动计算:深入研究Logistic回归
- vcenter接管_人工智能接管广告创意
web与ai相结合成为趋势_将AI和行为科学相结合可以改变体验相关推荐
- ai无法启动产品_启动AI启动的三个关键教训
ai无法启动产品 重点 (Top highlight) Let me be upfront: I was the technical co-founder of an AI startup and i ...
- ai自动生成字幕_使用AI对您的会议进行现场字幕
ai自动生成字幕 Many people rely on captions every day. Unfortunately, not everything has captions; this of ...
- ai人工智能算法工程师_与AI时代息息相关:阿里巴巴算法工程师指南
ai人工智能算法工程师 随着AI越来越擅长处理越来越多的计算任务,致力于开发AI的人们可能很快就会失业. 随着人工智能进入当今越来越多的技术中,有抱负的算法工程师面临着独特的困境. 一方面,他们必须竞 ...
- ai皮肤检测分数_智能AI皮肤检测仪三步走话术
智能 AI 皮肤检测仪三步走话术 第一步:仪器介绍 话术: 某某小姐,您好!我们现在用了是 德国进口智能 AI 皮肤检测仪 给您的皮肤做拍照 测试. 我们这台仪器是国外引起的一台专业皮肤定量分析仪器, ...
- ai如何旋转画布_「AI教程」使用AI制作3D立体文字效果
今天macdown小编要通过AI制作一种3D立体字体,主要的知识点是混合工具的使用.Shift键.Alt键的灵活运用.3D旋转命令.投影效果的创建等,学会后可以应用在需要的设计中,比如海报设计,log ...
- ai算子是什么_隐私AI框架中的数据流动与工程实现
在上一篇文章中,我们介绍了,对于安全技术开发者,如何快速的基于 Rosetta 等隐私 AI 框架所提供的一系列接口,将自己的安全协议集成落地到上层的 AI 应用中来.在这一篇文章中,我们将介绍为了保 ...
- ai项目实施步骤_停止AI产品开发中道德责任的6个步骤
ai项目实施步骤 这是官方的:人工智能(AI)并不是我们想要成为的公正的天才. Google的母公司Alphabet(Alphabet)使用其最新的年度报告来警告称,对其产品的道德顾虑可能会损害未来的 ...
- ai怎么画路线_使用AI画一个离心管
介绍 今天有粉丝提问,如何使用AI画一个离心管,对于这种由线条和简单的色彩组成的图,还是比较好画的.还有就是涉及到一些小工具的用法,配合着使用就可以画出来了.怎么样,你来学学吗?安排呗! 软件 Ado ...
- 怎么用ai做出适量插画_用AI打造矢量人像插画
小编:新年好..是否有小伙伴已开工啦? APP的引导页现在多数倒入小插画,更能表现场景氛围,用AI打造矢量人像插画这个技能必须get.一起去看看@飞屋睿带来的这篇教程吧- 前言 曾经看到有人这样说:画 ...
- ai切换rgb模式_当AI频繁切换色彩预览模式时 颜色会越变越深 求解?
当然会如此!因为这中间经历了一个三分色(四分色)的算法过程,所以当你转换的次数越多,他们的颜色畸变就越严重. AI中提供的RGB和CMYK的切换模式,目的是为了提供一个RGB转换CMYK的接口,而不是 ...
最新文章
- 关于不过洋节的通知_迁安各商家从今往后不再过“洋节”!
- 如何允许非Administrator用户远程登录主机
- 如何c51和mdk共存兼容_电磁兼容入门篇之基础知识(一)
- 深度学习(18)神经网络与全连接层一: 数据加载
- 使用Azure Blob Storage实现一个静态文件服务器
- P7408-[JOI 2021 Final]ダンジョン 3【贪心,树状数组】
- 企业如何快速应对市场环境的不断变化
- R.java文件无法自动生成的问题
- 奈飞win10安装包_使用Windows10系统在奈飞/网飞上观看4K的必要条件
- 高等数学(第七版)同济大学 习题2-1 个人解答
- 视频监控安防平台-GB35114和GB28181的注册信令
- 网络安全从业者“行话”
- 计算机win加r,快捷键win+r是什么意思_打开运行快捷键win+r怎么按
- 计算机软件专业如何选科,软件工程高中选什么科?附新高考软件工程专业选科要求...
- 微信小程序:语音变声器支持多种音效与流量主
- 社会分工的理想与现实
- A Survey on Malicious Domains Detection through DNS Data Analysis
- 阴阳师最新的服务器,阴阳师跨区服务器大全 集结之境新增跨区服务器汇总详解...
- 中国联通智慧银行大数据解决方案.ppt
- java判断文件夹下是否存在文件_java 判断文件夹是否存在文件
热门文章
- Swift中的Masonry第三方库——SnapKit
- 各种排序算法的实现直接插入排序
- 20200516每日一句
- opencv打开双目
- Atitit 文档资料整理的规范流程与问题解决目录1. 减肥 11.1. 剥离非原创类文件 11.2. 去重 11.3. 转换格式 21.4. Topic主题剥离 22. 脱敏 2
- Atitit go语言 golang 艾提拉总结特性优缺点 目录 1. Go 语言最主要的特性:	1 2. 体积大概100M	1 3. 问题	1 3.1. 编译速度和异常控制怎么样	1 3.2.
- Atitit.远程文件技术 webdav ftp scp ssh-sftp http nfs smb 的区别与总结
- Atitit webserver tomcat 7 8.0 8.5 9.0新特性 Tomcat 7 的七大新特性 - 编程语言 - ITeye资讯.html tomcat 8.0特性 - CSD
- paip.gui控件form窗体的原理实现以及easyui的新建以及编辑实现
- Paip.断点调试MYSQL存储过程跟函数的解决方案大法