lasso回归和岭回归_如何计划新产品和服务机会的回归
lasso回归和岭回归
Marketers sometimes have to be creative to offer customers something new without the luxury of that new item being a brand-new product or built-from-scratch service. In fact, incrementally introducing features is familiar to marketers of consumer goods. But what features are consistently being selected by customers? What should marketers look for to tell development teams, production teams, and partners to plan upon? Applying a regression may help.
营销人员有时必须具有创造力,才能为客户提供一些新东西,而不能将新商品的奢侈性视为全新产品或从头开始的服务。 实际上,消费品的营销人员已经逐渐引入功能。 但是客户一直在选择哪些功能? 营销人员应该寻找什么来告诉开发团队,生产团队和合作伙伴进行计划? 应用回归可能会有所帮助。
It’s not an easy question to answer, even among startups that usually have a unique take on a market. Entrepreneurs other confuse features with products and services, leading to poorly crafted business models. Products and services solve problems for people. Features are characteristics that add value to the products.
即使在通常在市场上占有独特地位的初创企业中,这也不是一个容易回答的问题。 企业家将产品和服务的其他功能混为一谈,从而导致制作不当的商业模式。 产品和服务为人们解决问题。 功能是增加产品价值的特征。
The major challenge for managers is determining what features would add value in a sustainable way. It’s easy to brainstorm ideas about what features a product or service should contain. The real challenge however is selecting that features that will draw customers consistently. This is the essence of building a business model.
管理人员面临的主要挑战是确定哪些功能将以可持续的方式增加价值。 就产品或服务应包含的功能集思广益的想法很容易。 然而,真正的挑战是选择能够持续吸引客户的功能。 这是建立业务模型的本质。
The Chevrolet Corvette Grand Sport is an example of selecting features to produce a different product offering. The Grand Sport trim level was introduced in 2017 for the C7 Corvette. C7 is a nickname for the seventh generation of Corvette. The latest version, a mid-engine is a C8, was introduced in 2019.
雪佛兰Corvette Grand Sport是选择功能来生产其他产品的示例。 Grand Sport内饰级别于2017年为C7 Corvette引入。 C7是第七代Corvette的昵称。 最新版本是中置引擎C8,于2019年推出。
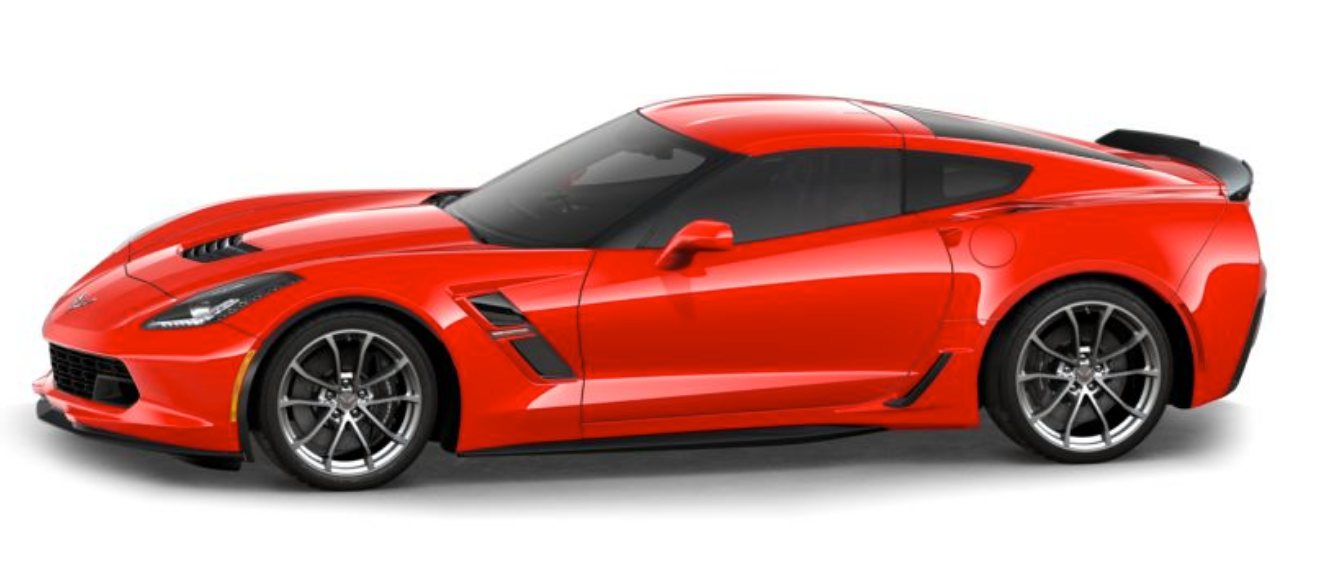
The Grand Sport is a hybrid of exterior and chassis features from a more expensive model, the Corvette Z06, with the engine and core interior features from the entry-level Corvette. The Grand Sport reached a 30% share of overall Corvette vehicle sales in its first year, so from an automotive industry perspective, it successfully contributed significant sales for the model.
Grand Sport混合了更昂贵的Corvette Z06的外观和底盘功能,以及入门级Corvette的发动机和核心内饰功能。 Grand Sport在第一年就占据了Corvette整车销售的30%,因此,从汽车行业的角度来看,它成功地为该车型贡献了可观的销售。
Combining features are not too complicated, a good brainstorming session with insightful customer comments can yield some ideas as to what features may be worth investigating. But you may want to see if those choices seem really correlated and sustainable. The last part is the most important. The last decision you want to make is one where features indeed seem correlated but are more of a blip rather than a predicted trend.
组合功能并不太复杂,一个很好的头脑风暴会议以及有见地的客户评论可以就可能值得研究的功能产生一些想法。 但是您可能想看看这些选择是否看起来确实相关且可持续。 最后一部分是最重要的。 您要做出的最后一个决定是,其中的功能看上去确实相关,但更多的是暂时的,而不是预测的趋势。
Regressions can back up a professional intuition by asking what sort of features are sustainable enough to support sales. A regression determines if data associated with the features reflect a relationship that represents sustainability. Regressions can add a logical influence on a business decision, especially on products that receive a ton of “fanboy” social media commentary but remain unclear if that interest turns into real sales. Fanboy comments on iconic sports cars like the Corvette are no exception.
回归可以通过询问什么样的功能足以支持销售来支持专业直觉。 回归确定与要素关联的数据是否反映了代表可持续性的关系。 回归可以对业务决策产生逻辑上的影响,尤其是对收到大量“粉丝”社交媒体评论但对这种兴趣是否转化为实际销售尚不清楚的产品。 Fanboy对像Corvette这样的标志性跑车的评论也不例外。
A useful first step in planning data is to consider a feature and how its observations are being measured. For example, if you want to compare engine size for a powered object like a lawnmower or motorcycle, then you would have a column with engine sizes for observations.
计划数据的有用的第一步是考虑一个特征以及如何测量其观测值。 例如,如果要比较割草机或摩托车等电动对象的发动机尺寸,则将有一个列,其中包含用于观察的发动机尺寸。
Determining a correlation is the next step. Gaining an idea of how the data roughly takes shape can be done in different ways. One quick-and-dirty way to create a scatter plot to see how the data takes a shape. A correlation is a more specific way to see if there is a linear correlation. The range of correlations is from -1 to 1. How close each value is calculated demonstrates the strength and direction of the correlation. You can then begin to examine a regression method that will likely provide the best fit that represents the correlation calculated.
确定相关性是下一步。 了解数据如何大致成形的想法可以通过不同的方式来完成。 一种创建散点图以查看数据如何成形的快捷方法。 相关性是查看是否存在线性相关性的更具体方法。 相关范围是-1至1。计算每个值的接近程度证明了相关的强度和方向。 然后,您可以开始研究一种回归方法,该方法可能会提供代表所计算相关性的最佳拟合。
A regression treats each feature as independent variables, then determines which one correlates to a dependent variable: the output we want. That output is usually sales, but the regression can also be created for a variation of sales, such as purchase orders or market share. Linear regressions are the simplest regression models. They can be made with just a few columns of data. They also assume a linear relationship between the outcome and the predictor variables.
回归将每个特征视为独立变量,然后确定哪个特征与因变量相关:我们想要的输出。 该输出通常是销售额,但是也可以为销售额的变化(例如采购订单或市场份额)创建回归。 线性回归是最简单的回归模型。 它们可以仅由几列数据组成。 他们还假设结果与预测变量之间存在线性关系。
For our Corvette example, a logistic regression can be useful. In a logistic regression, the dependent variable represents a binary choice (yes or no, approve or rejected). In this instance, it is easy to imagine columns of features and the dependent variable represents a binary choice, a sale or no sale. Dependent variables can also be represented as a lift in sales or sales decrease or an increase or decrease in market share.
对于我们的Corvette示例,逻辑回归可能会有用。 在逻辑回归中,因变量表示二元选择(是或否,批准或拒绝)。 在这种情况下,可以轻松想象特征列,并且因变量表示二进制选择,出售还是不出售。 因变量也可以表示为销售额上升或销售额下降或市场份额的上升或下降。
The method of regression can be influenced by the normality of the observations. The shape of the scatter plot can be a simple reveal. Does the data imply that a line best represents a regression, or is there a general curve that suggests a logistic regression? In most instances where the shape is not immediately obvious, you would apply a test such as Q-Q test.
回归方法可能会受到观测值的正态性影响。 散点图的形状可以很简单地显示出来。 数据是否暗示一条线最能代表回归,还是有一条总体曲线表明对数回归? 在大多数情况下,形状不是立即显而易见的,您可以应用诸如QQ测试之类的测试。
No matter how you set up a regression, a good model reveals a strong correlation among the features represented as the influential independent variables, which means finding the right combination of features that would likely entice a sale. A regression model may not be needed in every instance of combining features. But they are valuable in instances of uncertainty, particularly as a service adds an offering.
无论您如何建立回归,一个好的模型都会揭示以有影响力的自变量表示的特征之间的强相关性,这意味着找到可能会吸引销售的正确特征组合。 在每个组合特征的实例中可能都不需要回归模型。 但是它们在不确定的情况下非常有价值,尤其是当服务添加产品时。
Overall regressions can provide a robust means to reinforce intuition on feature combinations or enlighten opportunities for new services. Either case can lead to better business choices for products and services that can incrementally increase sales while minimizing investment.
整体回归可以提供一种强有力的手段来增强功能组合的直觉性或启发新服务的机会。 不论哪种情况,都可以为产品和服务带来更好的业务选择,从而可以在不增加投资的情况下逐步增加销售额。
翻译自: https://medium.com/better-marketing/how-to-plan-regressions-for-new-products-and-service-opportunities-ae69ed62a9de
lasso回归和岭回归
http://www.taodudu.cc/news/show-997364.html
相关文章:
- 贝叶斯 定理_贝叶斯定理实际上是一个直观的分数
- 文本数据可视化_如何使用TextHero快速预处理和可视化文本数据
- 真实感人故事_您的数据可以告诉您真实故事吗?
- k均值算法 二分k均值算法_使用K均值对加勒比珊瑚礁进行分类
- 衡量试卷难度信度_我们可以通过数字来衡量语言难度吗?
- 视图可视化 后台_如何在单视图中可视化复杂的多层主题
- python边玩边学_边听边学数据科学
- 边缘计算 ai_在边缘探索AI!
- 如何建立搜索引擎_如何建立搜寻引擎
- github代码_GitHub启动代码空间
- 腾讯哈勃_用Python的黑客统计资料重新审视哈勃定律
- 如何使用Picterra的地理空间平台分析卫星图像
- hopper_如何利用卫星收集的遥感数据轻松对蚱hopper中的站点进行建模
- 华为开源构建工具_为什么我构建了用于大数据测试和质量控制的开源工具
- 数据科学项目_完整的数据科学组合项目
- uni-app清理缓存数据_数据清理-从哪里开始?
- bigquery_如何在BigQuery中进行文本相似性搜索和文档聚类
- vlookup match_INDEX-MATCH — VLOOKUP功能的升级
- flask redis_在Flask应用程序中将Redis队列用于异步任务
- 前馈神经网络中的前馈_前馈神经网络在基于趋势的交易中的有效性(1)
- hadoop将消亡_数据科学家:适应还是消亡!
- 数据科学领域有哪些技术_领域知识在数据科学中到底有多重要?
- 初创公司怎么做销售数据分析_为什么您的初创企业需要数据科学来解决这一危机...
- r软件时间序列分析论文_高度比较的时间序列分析-一篇论文评论
- selenium抓取_使用Selenium的网络抓取电子商务网站
- 裁判打分_内在的裁判偏见
- 从Jupyter Notebook切换到脚本的5个理由
- ip登录打印机怎么打印_不要打印,登录。
- 机器学习模型 非线性模型_调试机器学习模型的终极指南
- 您的第一个简单的机器学习项目
lasso回归和岭回归_如何计划新产品和服务机会的回归相关推荐
- r语言中残差与回归值的残差图_独家 | 手把手教你用R语言做回归后的残差分析(附代码)-阿里云开发者社区...
作者:Abhijit Telang 翻译:张睿毅 校对:丁楠雅 文章来源:微信公众号 数据派THU 本文介绍了做残差分析的方法及其重要性,以及利用R语言实现残差分析. 在这篇文章中,我们通过探索残差分 ...
- 新公司只有短信通知入职_介绍我们新的短信通知服务
新公司只有短信通知入职 At eUKhost, we always aim to provide the best service and support to our customers. We w ...
- lasso回归_线性回归amp;lasso回归amp;岭回归介绍与对比
1. 回顾最小二乘法 详细的解释在以下这个链接 https://www.matongxue.com/madocs/818 简而言之,最小二乘法展现了平方误差值最小的时候,就是最好的拟合回归线. 2. ...
- python lasso做交叉验证法_标准方程法_岭回归_LASSO算法_弹性网
程序所用文件:https://files.cnblogs.com/files/henuliulei/%E5%9B%9E%E5%BD%92%E5%88%86%E7%B1%BB%E6%95%B0%E6%8 ...
- lasso回归与岭回归
lasso回归与岭回归 简介 lasso回归(Least Absolute Shrinkage and Selection Operator Regression)与岭回归(Ridge Regress ...
- 线性模型系数解读中的那些坑,以套索回归(LASSO)和岭回归(Ridege)为例
线性模型系数解读中的那些坑,以套索回归(LASSO)和岭回归(Ridege)为例 在线性回归类模型的训练过程中,模型通过数据拟合了目标变量y与特征变量X的关心,通过特征变量的线性组合来表征目标变量y; ...
- 线性回归——lasso回归和岭回归(ridge regression)
目录 线性回归--最小二乘 Lasso回归和岭回归 为什么 lasso 更容易使部分权重变为 0 而 ridge 不行? References 线性回归很简单,用线性函数拟合数据,用 mean squ ...
- 线性回归、lasso回归、岭回归以及弹性网络的系统解释
线性回归.lasso回归.岭回归以及弹性网络的系统解释 声明 背景介绍 概述 线性回归模型函数及损失函数 线性回归算法 多项式回归(线性回归的推广) 广义线性回归(线性回归的推广) 岭回归(Ridge ...
- 应用预测建模第六章-线性回归-预测化合物溶解度练习-R语言(多元线性回归,稳健回归,偏最小二乘回归,岭回归,lasso回归,弹性网)
模型:多元线性回归,稳健回归,偏最小二乘回归,岭回归,lasso回归,弹性网 语言:R语言 参考书:应用预测建模 Applied Predictive Modeling (2013) by Max K ...
最新文章
- Yii抛出的各种异常
- [Python]ConfigParser解析配置文件
- JMS中的消息通信模型
- c语言期末考试复习题
- IT桔子分布式项目1
- Codeforces Round #223 (Div. 2): E. Sereja and Brackets(线段树)
- android开发里遇到的坑——eclipse项目导入android studio以后Run按钮灰色
- PHP网上书店销售系统
- 泰拉瑞亚云服务器的搭建
- 【Android Studio】如果你不管怎么折腾都提示Minimum supported Gradle version is 7.0.2. Current version is 6.8,请看这里
- 串口服务器远程距离,基于串口服务器的变频器远程控制系统.pdf
- 【九度】题目1419:文献排序
- 如何获取vs code中插件Waka Time的API key
- poj3046 Ant Counting
- Direct3D中的纹理映射
- python 快速获取图片大小
- MQ常见问题及解决方案
- DirectShow 09 - 音视频捕捉
- static应用知识:代码块
- 旧电脑又卡又慢,一招解决!
热门文章
- InstallShield For .Net制作.Net项目安装包之完整代码
- tensorflow一元二次函数拟合
- 版本低于1.7的jQuery过滤用户输入数据所使用的正则表达式存在缺陷
- zooland 新开源的RPC项目,希望大家在开发的微服务的时候多一种选择,让微服务开发简单,并且容易上手。...
- 2018年应用交付控制器市场将发生重大变化
- “机器换人”之潮涌向珠三角,蓝领工人将何去何从
- P1401 城市(30分,正解网络流)
- go grpc 深入笔记
- Javascript构造函数的继承
- magento2邮件调试方法