创意产品 分析_使用联合分析来发展创意
创意产品 分析
Advertising finds itself in a tenacious spot these days serving two masters: creativity and data.
如今,广告业处于一个顽强的位置,服务于两个大师:创造力和数据。
On the one hand, it values creativity; and it’s not hard to understand why. Creativity helps make brands distinct from each other and meaningful to consumers, it wins awards and improves recognition of the brands, agencies and people involved. And according to Hurman (2016) — who analysed three decades of data — more creative advertising is more effective advertising, driving sales and revenue.
一方面,它重视创造力。 而且不难理解为什么。 创意有助于使品牌彼此不同并对消费者有意义,它可以赢得奖项并提高对品牌,代理商和相关人员的认可。 根据赫尔曼(Hurman,2016)的研究,他分析了三十年的数据,创意广告越有效,广告的销售量和收入就越高。
On the other hand, we are seeing the rise and rise of data. From its beginnings in advertising as post-campaign performance measurement to prove the effectiveness of advertisements, and as an input to strategy development; data has morphed into a powerful force that can influence every aspect of advertising that cannot be ignored. We now have programmatic advertising, personalisation at every point of contact, content-matching algorithms and interactive OOH.
另一方面,我们正在看到数据的兴起。 从广告开始就作为广告活动后的绩效衡量指标,以证明广告的有效性,并作为战略制定的依据; 数据已演变成强大的力量,可以影响广告不可忽视的各个方面。 现在,我们有了程序化广告,每个联系点的个性化,内容匹配算法和交互式OOH。
But the two are not easy bedfellows, as Tim Nudd of Adweek says: “data has always been a bit terrifying to creative people. It’s often seen — sometimes fairly, sometimes not — as a replacement for intuition rather than a way to supplement it”.
但正如Adweek的蒂姆·纳德 ( Tim Nudd )所说,这两者并不容易。 人们经常(有时是公平,有时不是)将其视为直觉的替代,而不是补充直觉的方式。”
Yet data science — as a discipline — is not just about ‘data’. It includes research, analysis and ultimately method and reasoning. These other aspects of data science could be easily applied to creative decision making without taking away from intuition, expression and creative experience. As Payam Cherchian of AKQA says: “more and more clients are coming to the table expecting agencies to have data/ research on every slide to back up their creative ideas, assumptions and strategies”.
然而,作为一门学科的数据科学不仅与“数据”有关。 它包括研究,分析以及最终的方法和推理。 数据科学的这些其他方面可以很容易地应用于创意决策,而无需脱离直觉,表达和创意经验。 正如AKQA的Payam Cherchian所说:“越来越多的客户来到餐桌上,期望代理商在每张幻灯片上都进行数据/研究,以支持他们的创意,假设和策略。”
创意者的问题 (The Creative’s Problem)
The time between receiving a proposal request and submitting it is short, and creatives need to perform a number of mental tasks in that timeframe. They need to understand the brief, ideate, decide on an idea (or two), develop the idea, detail how to make it happen, and budget the idea.
从收到提案请求到提交提案请求的时间很短,并且广告素材需要在该时间范围内执行许多心理任务。 他们需要了解摘要,构思,决定一个(或两个)构思,发展该构思,详细说明如何实现该构思并为该构思进行预算。
Because time is limited the idea(s) presented in the proposal to the client are not always fully fleshed out. The general idea is there, but either the intricate details are missing or assumptions are made; and once the client has signed off on the idea, it needs to move into production. This is where conjoint analysis could be employed to give some data to the creatives decisions.
由于时间有限,建议书中呈现给客户的想法并不总是完全充实。 总体思路就在那里,但是要么错综复杂的细节遗漏,要么做出假设。 一旦客户批准了该想法,就需要投入生产。 在这里可以使用联合分析为创意决策提供一些数据。
联合分析简介 (Intro to Conjoint Analysis)
Conjoint analysis is a choice modelling technique used in market research primarily to find the optimal combination of attributes for a new product. The methodology helps uncover the preferences individuals have by designing different versions of the same product (i.e. 3 ice cream flavours and choice of cone = 9 combinations) and asking potential consumers to rank the different versions of a product from best to worst.
联合分析是一种用于市场研究的选择建模技术,主要用于为新产品找到最佳属性组合。 该方法通过设计同一产品的不同版本(即3种冰淇淋口味和9种组合选择),并要求潜在消费者对产品的不同版本(从最佳到最差)进行排名,从而帮助发现个人的喜好。
Choices can be influenced by many psychological, situational and social factors such as habit, inertia, experience, advertising, peer pressure, opinions, etc… but underneath all that it is assumed there is this thing called utility (or value) in the consumers mind that represents how important/ preferred/ desirable each option is (Louviere et al, 2000).
选择可能会受到许多心理,情境和社会因素的影响,例如习惯,惯性,经验,广告,同伴压力,意见等……但是,在所有假定的前提下,消费者的脑海中都存在一种称为效用 (或价值)的东西。代表每种选择的重要性/偏好/理想性(Louviere等,2000)。
While it would be nice to assume we could just analyse the rankings of product versions with each specific attribute (i.e. how high is chocolate ice cream ranked?), conjoint analysis calculates utilities (called part-worths) for each attribute based on the rankings of consumers (Härdle and Simar, 2012: 413) that are used to:
最好假设我们可以分析具有每个特定属性的产品版本的排名(例如,巧克力冰淇淋的排名是多少?),联合分析基于以下属性的排名来计算每个属性的效用 (称为部分价值):习惯于以下方面的消费者(Härdle和Simar,2012:413)
- determine the optimal combination of attributes to maximise utility确定属性的最佳组合以最大化效用
- determine the importance of each attribute according to consumers根据消费者确定每个属性的重要性
- design alternative combinations and analyse their utility设计替代组合并分析其效用
This is how market research is able to determine consumers are interested in a zero-interest, red credit card with a loyalty program that gives you 300 bonus points upon sign-up vs a 5% interest, blue card without a loyalty program.
这就是市场研究如何确定消费者对具有忠诚度计划的零利率红色信用卡感兴趣的原因,该计划在注册后为您提供300的奖励积分,而对于没有忠诚度计划的5%利息的蓝色信用卡。
What if we made one… small… change…
如果我们做了一个……很小的……改变……
Instead of comparing product attributes we were comparing the components of a creative idea that we are thinking of pitching to a client? This would tell us which combination of attributes for our creative idea have the most appeal to the target audience. It would also tell us which attributes are most and least important in their choice (which we should emphasise).
我们没有比较产品属性,而是比较了我们考虑向客户推销的创意的组成部分? 这将告诉我们,我们创意的哪些属性组合对目标受众最有吸引力。 它还会告诉我们哪些属性在选择中最重要和最不重要(我们应该强调)。
一个例子 (An Example)
Let’s assume we’ve received a RFP and the creative team have struck upon an idea to run a secret concert that people have to locate. As the turnaround time for the proposal is incredibly short, that’s about as far the creative team have come with developing the idea. Many questions remain:
假设我们已经收到了RFP,而创意团队想出了一个举办人们必须寻找的秘密音乐会的想法。 由于该提案的周转时间非常短,因此创意团队已经提出了这个想法。 仍然有许多问题:
- how many bands are playing at this concert?这个音乐会上有多少支乐队在演奏?
- is there just one concert or several, in different cities?在不同的城市里只有一场音乐会还是几场?
- do we provide clues or should people follow a signal?我们提供线索还是人们应该遵循信号?
The team could make decisions based on their opinions or budget, or we can use data to see what consumers think using traditional conjoint*.
团队可以根据他们的意见或预算做出决定,或者我们可以使用数据来查看消费者使用传统的联合体*的想法。
The first thing we need to do is design the choice model. In this example we have three attributes each with two levels (options):
我们需要做的第一件事是设计选择模型。 在此示例中,我们具有三个带有两个级别(选项)的属性:

Because the number of attributes and levels is small, the total number of combinations is small, 8 in fact, leading to a factorial design (which represents every possible combination):
因为属性和级别的数量很少,所以组合的总数很小,实际上是8,这导致了阶乘设计 (代表每种可能的组合):
- one band, in Melbourne, send out clues一支乐队在墨尔本发出线索
- one band, in Melbourne, follow the signal墨尔本的一个乐队,跟随信号
- one band, in 5 capital cities, send out clues5个省会城市的一支乐队发出线索
- one band, in 5 capital cities, follow the signal5个省会城市中的一个乐队,跟随信号
- several bands, in Melbourne, send out clues墨尔本的几支乐队发出线索
- several bands, in Melbourne, follow the signal墨尔本有几个乐队跟随信号
- several bands, in 5 capital cities, send out clues5个省会城市的几个乐队发出线索
- several bands, in 5 capital cities, follow the signal5个省会城市的几个乐队跟随信号
Side note: when the number of attributes and levels start to increase, the number of possible combinations gets very high. Too high for anyone to rank the combinations in a sensible order. In these cases we take only a subset of combinations — such that will still give us enough information to run the analysis on all the attributes. This is called a fractional factorial design. As a rule of thumb, when there are more than 10 combinations, you should use a fractional factorial design.
旁注:当属性和级别的数量开始增加时,可能的组合数量会非常多。 太高了,无法以合理的顺序对组合进行排名。 在这些情况下,我们仅采用组合的子集-这样仍将为我们提供足够的信息以对所有属性进行分析。 这称为分数阶乘设计 。 根据经验,当组合超过10个时,应使用分数阶乘设计。
Now we can design a survey and administer it to a group of consumers in the target audience. According to Peduzzi et al. (1996), the minimum sample size for a choice experiment can be worked out with the following formula:
现在,我们可以设计调查并将其管理给目标受众中的一组消费者。 根据Peduzzi等。 (1996),选择实验的最小样本量可以用以下公式计算:

where q is the number of questions asked in the survey, a is the number of combinations per question, and c is the maximum number of levels of any attribute in the choice model. Given we have 1 question, asking respondents to rank 8 combinations and the most levels of any attribute is 2, this reveals we need a sample of at least 250.
其中q是调查中提出的问题数量, a是每个问题的组合数量, c是选择模型中任何属性的最大级别数。 假设我们有1个问题,则要求受访者对8个组合进行排名,并且任何属性的最高水平为2,这表明我们需要至少250个样本。
We can ask each respondent to rank these combinations from best (1) to worst (8), plug the raw data into a spreadsheet or R to run the analysis. I’ve setup a Google Sheet to run this type of conjoint analysis that can handle up to 10 combinations of 6 attributes and a sample size of 500, but you could do more in R or specialist software like Sawtooth and Q.
我们可以要求每个受访者对这些组合从最佳(1)到最差(8)进行排名,将原始数据插入电子表格或R中以运行分析。 我已经设置了一个Google表格来运行这种类型的联合分析,最多可以处理6个属性的10个组合,样本大小为500,但是您可以在R或Sawtooth和Q之类的专业软件中进行更多操作。
Assume we get the following results:
假设我们得到以下结果:

The importance metric tells us how ‘important’ or ‘preferred’ each attribute was in the consumers ranking of the options. It tells us that the number of bands was the most important factor in their decisions.
重要程度指标告诉我们,每个属性在选项的消费者排名中是“重要”还是“优先”。 它告诉我们,乐队的数量是他们决定的最重要因素。
The part-worths breakdown the attribute preference further to show which levels ‘increase’ perceived utility (consumer preference) and which detract. Here, the results suggest the best combination is:
部分价值进一步分解属性偏好,以显示哪些水平“增加”了感知效用(消费者偏好),哪些降低了。 在这里,结果表明最佳组合是:
- one band, in Melbourne, follow the signal墨尔本的一个乐队,跟随信号
This now tells the creative team not to worry about sourcing several bands for the concert nor worry about finding locations in other cities; giving them more time and energy focus on developing a better experience that people actually want.
现在,这告诉创意团队不必担心会为音乐会筹集多个乐队,也不必担心会在其他城市找到地点。 给他们更多的时间和精力专注于开发人们真正想要的更好的体验。
Conjoint analysis doesn’t replace the role of the creative in ideation, nor does it leave data as an end product to evaluate creative performance. It helps support creative decision making with rigour and enables data to play a larger role than just an API connection to a database for personalising advertisements.
联合分析不会取代创意在创意中的作用,也不会将数据作为评估创意效果的最终产品。 它不仅可以帮助支持严谨的创意决策,还可以使数据发挥更大的作用,而不仅仅是与数据库的API连接以个性化广告。
实现它 (Making it happen)
Implementing conjoint analysis to creative decision making does not require much change to current processes. In fact it requires a bit more communication between creatives and analysts and some patience:
在创意决策中实施联合分析不需要对当前流程进行太多更改。 实际上,这需要广告素材和分析师之间进行更多的交流,并且要有一定的耐心:
第1步:创建广告素材感兴趣的属性和级别的列表 (Step 1: create a list of attributes and levels the creatives are interested in)
- Keep to 2–6 attributes and 2–4 levels per attribute保留2–6个属性,每个属性2-4个级别
- Avoid using attributes that are hard to specify or quantify — like high/ low quality避免使用难以指定或量化的属性,例如高质量/低质量
- More attributes provide a more accurate picture, but becomes too much information for a respondent to think about更多的属性可以提供更准确的描述,但对于受访者而言,它成为太多信息而无法考虑
- Check how much did your mental image of the idea change with the inclusion of each component, if not much, then it might not be relevant检查您对创意的心理印象在包含每个成分的情况下发生了多少变化,如果变化不大,那么可能不相关
步骤2:设计人们会排名的替代组合 (Step 2: design alternative combinations that people will rank)
- With a small number of attributes and levels, you could have people rank every possible combination使用少量的属性和级别,您可以使人们对每种可能的组合进行排名
- In cases that the number of combinations is large, you will need to select a smaller number of combinations using a special methodology (your analyst will need to do this)如果组合的数量很大,则需要使用特殊的方法来选择较小数量的组合(您的分析师将需要执行此操作)
- Avoid prohibited pairs (i.e. speed and fuel economy) because that is not realistic避免使用禁止对(即速度和燃油经济性),因为这是不现实的
You can work out the minimum number of combinations needed (Cordella et al, 2013) where t is the total number of levels across all attributes and a is the number of attributes
您可以计算出所需的最小组合数(Cordella等,2013),其中t是所有属性的级别总数, a是属性的数量
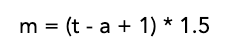
- Because choice experiments are dependent on the design of the study, you cannot simply remove faulty combinations from the survey. You cannot add or remove attributes or levels either由于选择实验取决于研究的设计,因此您不能简单地从调查中删除错误的组合。 您不能添加或删除属性或级别
步骤3:确定样本量并发送调查 (Step 3: determine the sample size and send out survey)
- Who are you surveying? They might be existing customers from the clients CRM, or leads from a web form, or fans from the clients Facebook page. You might be fortunate enough to run it via Google Surveys for a representative sample of the general population.您正在调查谁? 他们可能是客户CRM的现有客户,也可能是Web表单的潜在客户,也可能是客户Facebook页面的粉丝。 您可能很幸运,可以通过Google调查来针对一般人群进行代表性采样。
- The data collection should be quick given that the survey is rather short (1 question). I’d expect a turnaround time of 1–2 weeks and close it after that point.鉴于调查时间较短(1个问题),因此数据收集应Swift。 我希望周转时间为1-2周,然后将其关闭。
步骤4:进行分析并将结果与创意团队的初步想法进行比较 (Step 4: run the analysis and compare results to creative team’s initial thoughts)
The two key metrics are importance and part-worths. Importance tells you to what extent each attribute influenced people’s preferences and the part-worths break that down into the individual levels of each attribute.
两个关键指标是重要性和部分价值 。 重要性告诉您每个属性在多大程度上影响了人们的偏好,而部分价值则将其分解为每个属性的各个层次。
The analysis should also produce a little simulator that allows you to calculate the utility value of any combination of attributes and levels. This is useful when creative ideas are constrained by budget or client (i.e. can no longer use the follow the signal hint, so what’s our next best option?)
分析还应产生一个小的模拟器,使您可以计算属性和级别的任何组合的效用值。 当创意受到预算或客户的限制时,此功能很有用(即无法再使用遵循信号提示,那么我们的下一个最佳选择是什么?)
最后的想法 (Final Thoughts)
I hope that I’ve given you a new opportunity to introduce data and analytical thinking to your creative work, and shown you that the two can be harmoniously integrated in a much better way than they currently are. If you enjoyed this post, please share it with your friends.
希望我给您了一个新的机会,可以将数据和分析思维引入您的创意工作,并向您展示可以以比目前更好的方式将两者融为一体。 如果您喜欢这篇文章,请与您的朋友分享。
*Of the three conjoint methods: traditional (TC), menu-based (MBC), and choice-based (CBC) I think traditional is probably easiest to apply to creative problem solving because (1) it can be setup in a spreadsheet (MBC and CBC require specialist software), and (2) the number of attributes they would be interested in would be low (3–5)
*在三种联合方法中:传统(TC),基于菜单(MBC)和基于选择(CBC),我认为传统可能最容易应用于创意问题解决,因为(1)可以在电子表格中进行设置( MBC和CBC需要专业软件),并且(2)他们感兴趣的属性数量很少(3-5)
翻译自: https://medium.com/sigma-1/using-conjoint-analysis-to-develop-creative-ideas-8d6344f58ca2
创意产品 分析
http://www.taodudu.cc/news/show-997525.html
相关文章:
- 多层感知机 深度神经网络_使用深度神经网络和合同感知损失的能源产量预测...
- 使用Matplotlib Numpy Pandas构想泰坦尼克号高潮
- pca数学推导_PCA背后的统计和数学概念
- 鼠标移动到ul图片会摆动_我们可以从摆动时序分析中学到的三件事
- 神经网络 卷积神经网络_如何愚弄神经网络?
- 如何在Pandas中使用Excel文件
- tableau使用_使用Tableau升级Kaplan-Meier曲线
- numpy 线性代数_数据科学家的线性代数—用NumPy解释
- 数据eda_银行数据EDA:逐步
- Bigmart数据集销售预测
- dt决策树_决策树:构建DT的分步方法
- 已知两点坐标拾取怎么操作_已知的操作员学习-第3部分
- 特征工程之特征选择_特征工程与特征选择
- 熊猫tv新功能介绍_熊猫简单介绍
- matlab界area_Matlab的数据科学界
- hdf5文件和csv的区别_使用HDF5文件并创建CSV文件
- 机器学习常用模型:决策树_fairmodels:让我们与有偏见的机器学习模型作斗争
- 100米队伍,从队伍后到前_我们的队伍
- mongodb数据可视化_使用MongoDB实时可视化开放数据
- Python:在Pandas数据框中查找缺失值
- Tableau Desktop认证:为什么要关心以及如何通过
- js值的拷贝和值的引用_到达P值的底部:直观的解释
- struts实现分页_在TensorFlow中实现点Struts
- 钉钉设置jira机器人_这是当您机器学习JIRA票证时发生的事情
- 小程序点击地图气泡获取气泡_气泡上的气泡
- PopTheBubble —测量媒体偏差的产品创意
- 面向Tableau开发人员的Python简要介绍(第3部分)
- pymc3使用_使用PyMC3了解飞机事故趋势
- 吴恩达神经网络1-2-2_图神经网络进行药物发现-第2部分
- 数据图表可视化_数据可视化十大最有用的图表
创意产品 分析_使用联合分析来发展创意相关推荐
- 100件创意产品设计_增强创意信心的20件事
100件创意产品设计 重点 (Top highlight) 都是有创意的. 您只需要一推即可. (All are creative. You just need a push.) People exp ...
- python灰色关联度分析_灰色关联分析法 python
广告关闭 腾讯云11.11云上盛惠 ,精选热门产品助力上云,云服务器首年88元起,买的越多返的越多,最高返5000元! 本文2290字,预计阅读需10分钟: 关联分析(association anal ...
- 转录组分析_高级转录组分析和R数据可视化
封面来源:https://www.zhihu.com/question/304747766 常规转录组是我们最常接触到的一种高通量测序数据类型,其实验方法成熟,花费较低,是大部分CNS必备的技术,以后 ...
- python酒店评论分析_酒店舆情分析
Python进行携程酒店评论舆情分析 第一步:分析设计 我们是对酒店进行舆情分析,所有我们的核心是酒店的评论数据:对住客的评论数据进行特征提取,通过住客对酒店的评分,对数据进行分类,并使用朴素贝叶斯算 ...
- python歌词分析_用Python分析周杰伦6.5W字的歌词,原来他是这样的人
原标题:用Python分析周杰伦6.5W字的歌词,原来他是这样的人 雷锋网按:本文作者冬鑫,原文载于作者,雷锋网已获授权.文中所有链接详见文末"阅读原文". 前一段时间分析民谣歌词 ...
- java 象限分析_四象限分析法
四象限分析法,也叫矩阵分析法,是指将事物(如产品.服务等)的两个重要属性(指标)作为分析的依据,进行分类关联分析,找出解决问题的一种分析方法. 以属性A为横轴,属性B为纵轴,组成一个坐标系,在两坐标轴 ...
- 各类软件马斯洛需求层次分析_需求的分析层次
各类软件马斯洛需求层次分析 When I joined Square, I was embedded on a product that had been in-market for a year b ...
- 轨迹分析_单细胞轨迹分析知多少拟时间分析比较
轨迹推断(TI,trajectory inference)可以根据测序细胞之间表达模式的相似性对单细胞沿着轨迹进行排序,以此来模拟细胞动态变化的过程,是单细胞研究应用中一种常用且重要的方法,轨迹推断也 ...
- 什么叫pmt测试分析_直读分析光谱仪核心配件
目前,直读光谱仪根据核心部件可分成三种,PMT技术,CCD技术,CMOS技术,还有出现的拥有两种核心部件的直读光谱仪.如果一种器件真的已经能够替代另一种,并且性能优越,目前市场中就会消失一种,但是三种 ...
最新文章
- 爬取了1W个字节跳动岗位信息,我发现了什么?
- php怎么随机生成二个中文,PHP生成随机字符串的两种办法
- Android复习07【创建数据库、insert()插入数据、查看数据库、根据列索引获取参数值、根据列名-返回索引、增删改查数据、数据分页、修改表结构、Room框架】
- delphi mysql dll直接_十万火急!!!那位高手用过libmysql.dll直接连接MySql数据库?如何将二进制文件保存到blob字段中? (60分)...
- ES6 (总结篇二)
- linux命令补遗 - 1
- POJ 3415 Common Substrings (后缀数组,长度不小于k的公共子串的个数)
- office密码解锁工具
- PN结/三极管/MOSFET理解
- 运放参数的详细解释和分析-part2-如何测量输入偏置电流Ib和输入失调电流Ios
- 【超详细】Windows平台安装 apache 2.4
- 用python画钢铁侠_秒变钢铁侠,这个暑假,用 Python 让自己成为超级英雄!
- ajax post请求导致的跨域和浏览器兼容性问题
- 软件工程之功能性需求和非功能性需求
- win10 jdk环境变量配置
- iOS:crash崩溃日志分析
- sql和python还有c语言_TIOBE 4 月排行榜:SQL 进入前十,Python 继续攀升
- C#网络编程复习资料
- Type interface com.dao.StudentDao is not known to the MapperRegistry.
- 自然语言处理常见算法与模型
热门文章
- matlab求解常微分方程组/传染病模型并绘制SIR曲线
- 随笔--互联网进化论
- Python ValueError: IO operation on closed file
- 解决Warning: Cannot modify header information - headers already sent b...
- 关于向MySQL插入一条新纪录的问题
- Spring Cloud 中 分布式事务解决方案 -- 阿里GTS的使用
- Windows Server 2008 NFS
- Mecanim动画系统
- remoteing2
- 1.4版本上线(第八次会议)