柯尔莫可洛夫-斯米洛夫检验(Kolmogorov–Smirnov test,K-S test)
柯尔莫哥洛夫-斯米尔诺夫检验(Колмогоров-Смирнов检验)基于累计分布函数,用以检验两个经验分布是否不同或一个经验分布与另一个理想分布是否不同。
在进行cumulative probability统计(如下图)的时候,你怎么知道组之间是否有显著性差异?有人首先想到单因素方差分析或双尾检验(2 tailed TEST)。其实这些是不准确的,最好采用Kolmogorov-Smirnov test(柯尔莫诺夫-斯米尔诺夫检验)来分析变量是否符合某种分布或比较两组之间有无显著性差异。
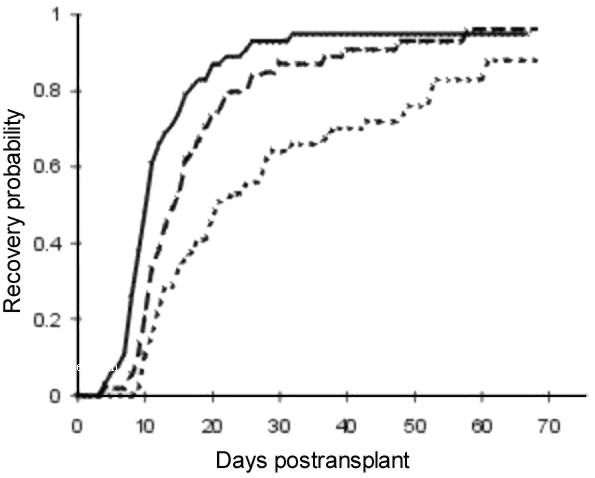
在统计学中,柯尔莫可洛夫-斯米洛夫检验基于累计分布函数,用以检验两个经验分布是否不同或一个经验分布与另一个理想分布是否不同。
在进行累计概率(cumulative probability)统计的时候,你怎么知道组之间是否有显著性差异?有人首先想到单因素方差分析或双尾检验(2 tailedTEST)。其实这些是不准确的,最好采用Kolmogorov-Smirnov test(柯尔莫诺夫-斯米尔诺夫检验)来分析变量是否符合某种分布或比较两组之间有无显著性差异。
分类:
1、Single sample Kolmogorov-Smirnov goodness-of-fit hypothesis test.
采用柯尔莫诺夫-斯米尔诺夫检验来分析变量是否符合某种分布,可以检验的分布有正态分布、均匀分布、Poission分布和指数分布。指令如下:
>> H = KSTEST(X,CDF,ALPHA,TAIL) % X为待检测样本,CDF可选:如果空缺,则默认为检测标准正态分布;
如果填写两列的矩阵,第一列是x的可能的值,第二列是相应的假设累计概率分布函数的值G(x)。ALPHA是显著性水平(默认0.05)。TAIL是表示检验的类型(默认unequal,不平衡)。还有larger,smaller可以选择。
如果,H=1 则否定无效假设; H=0,不否定无效假设(在alpha水平上)
例如,
x = -2:1:4
x =
-2 -1 0 1 2 3 4
[h,p,k,c] = kstest(x,[],0.05,0)
h =
0
p =
0.13632
k =
0.41277
c =
0.48342
The test fails to reject the null hypothesis that the values come from a standard normal distribution.
2、Two-sample Kolmogorov-Smirnov test
检验两个数据向量之间的分布的。
>>[h,p,ks2stat] = kstest2(x1,x2,alpha,tail)
% x1,x2都为向量,ALPHA是显著性水平(默认0.05)。TAIL是表示检验的类型(默认unequal,不平衡)。
例如,x = -1:1:5
y = randn(20,1);
[h,p,k] = kstest2(x,y)
h =
0
p =
0.0774
k =
0.5214
wiki翻译起来太麻烦,还有可能曲解本意,最好看原版解释。
In statistics, the Kolmogorov–Smirnov test (K–S test) is a form of minimum distance estimation used as a nonparametric test of equality of one-dimensional probability distributions used to compare a sample with a reference probability distribution (one-sample K–S test), or to compare two samples (two-sample K–S test). The Kolmogorov–Smirnov statistic quantifies a distance between theempirical distribution function of the sample and the cumulative distribution function of the reference distribution, or between the empirical distribution functions of two samples. The null distribution of this statistic is calculated under the null hypothesis that the samples are drawn from the same distribution (in the two-sample case) or that the sample is drawn from the reference distribution (in the one-sample case). In each case, the distributions considered under the null hypothesis are continuous distributions but are otherwise unrestricted.
The two-sample KS test is one of the most useful and general nonparametric methods for comparing two samples, as it is sensitive to differences in both location and shape of the empirical cumulative distribution functions of the two samples.
The Kolmogorov–Smirnov test can be modified to serve as a goodness of fit test. In the special case of testing for normality of the distribution, samples are standardized and compared with a standard normal distribution. This is equivalent to setting the mean and variance of the reference distribution equal to the sample estimates, and it is known that using the sample to modify the null hypothesis reduces the power of a test. Correcting for this bias leads to theLilliefors test. However, even Lilliefors' modification is less powerful than the Shapiro–Wilk test or Anderson–Darling test for testing normality.[1]
柯尔莫可洛夫-斯米洛夫检验(Kolmogorov–Smirnov test,K-S test)相关推荐
- 坚持真理的艰辛——罗巴切夫斯基创立非欧几何的艰难历程
1893年,在喀山大学树立起世界上第一个数学家的塑像.这位数学家就是俄国的伟大学者.非欧几何的创始人之一罗巴切夫期基(H.N.JIoqaheBCKNN,1792-1856).非欧几何是人类认识史上一个 ...
- 亚当·扎加耶夫斯基诗十二首
亚当·扎加耶夫斯基诗十二首 黄灿然译 神秘主义入门 天气很暖和,光很充沛. 咖啡馆露台上那德国人 膝上搁着一本小书. 我瞥见那书名: <神秘主义入门>. 突然间我明白了,那些 打着尖利的忽 ...
- 【卡米洛特工程】杰克.辛普森(Jake Simpson)的故事——世上最重大的秘密!
关键词.短语: 急速阅读(超)能力 与外星人的合作 罗斯威尔事件 超光速飞船 太阳系巨型舰队 艾森豪威尔会见ET ...
- matlab-罗曼诺夫斯基准则剔除粗大值
罗曼诺夫斯基准则原理 罗曼诺夫斯基准则又称 t检验准则,其特点是首先删除一个可疑的的测得值,然后按 t分布检验被剔除的测量值是否含有粗大误差 罗曼诺夫斯基准则 1)选取合适的显著度a,选择合适 ...
- java 维文生成图片_维文、哈萨克文、柯尔克孜文检测 (java实现把UTF-8转为unicode)...
对于特定语言的检测并不是一个困难的问题:统计一段文字中有多少个字符落在某语言的基本语素集合(例如:中文中的声韵母)中,然后计算比例,就可以给出答案. 已经有ibm的icu在这方面做得很好,而且计算速度 ...
- 塔夫茨计算机科学,塔夫斯大学计算机科学博士专业详情及要求是怎样的?
1.塔夫斯大学计算机科学博士专业介绍: 塔夫斯大学计算机科学系为博士生提供了广泛的财务支持,形式为fellowships和助研和助教奖学金.这些职位覆盖九个月,是学费和助学金的组合,塔夫斯大学计算机科 ...
- 塔夫斯大学计算机专业,Tufts的ECE「塔夫茨大学电气与计算机工程系」
塔夫斯大学电气与计算机工程系开设以下研究生学位课程: 电气工程理学硕士Master of Science in Electrical Engineering:塔夫斯大学电气工程理学硕士项目提供论文(T ...
- 歪写数学史(只要真理——罗巴切夫斯基)
即使目前为止的出场人物里超过半数来自法国, 我依然跳过了如帕斯卡, 傅里叶,彭赛列,泊松这样伟大的名字.当我再次审视巴黎这座时尚之都的时候, 只有戴上墨镜才能不被那一道道比 Hermes.Chanel ...
- python求圆周率马青公式_Python 实现丘德诺夫斯基(Chudnovsky)法計算高精度圓周率...
该楼层疑似违规已被系统折叠 隐藏此楼查看此楼 源码:(需要将 @ 替换成 ASCII空格) # -*- coding: UTF-8 -*- # 丘德诺夫斯基法計算高精度圓周率程序 # Calculat ...
最新文章
- 10分钟理解CSS3 FlexBox
- C++中多态的概念和意义
- Android Unable to resolve target 'android-8'
- 三星 P600 android,顶级硬件S pen笔手写—三星P600_三星 Galaxy Note 10.1 2014 Edition P600_平板电脑市场-中关村在线...
- python 打开excel并在屏幕上呈现_excel-检查文件是否在Python中打开
- 分布式 开源_3个开源分布式跟踪工具
- mysql insert update 同时执行_MySQL进阶三板斧(三)看清“触发器 (Trigger)”的真实面目
- MySQL 8.0 异步复制的三种方式
- String StringBuilder StringBuffer
- 交叉验证与训练集、验证集、测试集
- java.util.LinkedHashMap cannot be cast to
- LayoutLM——文本与布局的预训练用于文档图像理解
- 19.猜数字的游戏:随机生成数字与输入数字进行比较
- 数组的c语言代码带注释,C语言之数组
- c语言中整型常量ox,C语言基础教程:数字常量
- 前摩拜创始人出任百度造车CEO
- “打开ftp服务器上的文件夹时发生错误,请检查是否有权限访问该文件夹
- Pycharm 图像分辨率调整 学习笔记
- 高级程序猿是如何练成的
- Nginx输出header到access日志文件
热门文章
- 宝捷信注塑机PS系列采集方案
- Android Socket通信read阻塞解决办法
- 九月书单3期-《终身成长》《象与骑象人》《1Q84》《法国革命史》
- 外汇投资策略——超短线策略
- JSOI2019 Round1(十二省联考)游记
- 手机c语言多文件编程,C语言多文件编程
- 驼峰式与下划线命名规则
- java bulk_Elasticsearch Java High Level REST Client(Bulk API)
- Stanford的Chinese Segmenter
- 夏津一中2021高考成绩查询,2021德州市地区高考成绩排名查询,德州市高考各高中成绩喜报榜单...