机器学习与分布式机器学习_机器学习治疗抑郁症
机器学习与分布式机器学习
重点 (Top highlight)
Machine learning is a hot topic that has permeated numerous public and private industries, as well as a diverse spectrum of academic disciplines, extending far beyond its humble computer science origins. Machine learning techniques are generally considered a subset of the broader field of artificial intelligence (AI), although these two terms are sometimes used interchangeably. Health care is one such industry that has attempted to apply machine learning techniques to a multitude of tasks. Machine learning is by far the most prevalent application of AI within the health care industry, including both physical health [1], and mental health [2, 3]. The goals of machine learning applications within healthcare generally strive to enhance clinical understanding and/or improve patient care. More specifically, there is a growing body of research focused on using machine learning to improve patient screening, diagnosis, clinical decision making, and specific treatment outcomes [1, 2, 4].
中号 achine学习是已经渗透到许多公共和私人行业,以及学科的多频谱,扩大远远超出了其卑微的计算机科学的起源的一个热门话题。 机器学习技术通常被认为是更广泛的人工智能(AI)领域的子集,尽管这两个术语有时可以互换使用。 卫生保健就是其中一种尝试将机器学习技术应用于众多任务的行业。 到目前为止,机器学习是AI在医疗保健行业中最普遍的应用,包括身体健康[1]和心理健康[2,3]。 医疗保健中机器学习应用程序的目标通常致力于增强临床理解和/或改善患者护理。 更具体地说,越来越多的研究致力于使用机器学习来改善患者的筛查,诊断,临床决策和特定治疗结果[1、2、4]。
The application of machine learning to mental health lagged a bit behind its elder sibling physical health, however, we have seen a rapidly growing body of research in recent years applied to various facets of mental health treatment.
机器学习在心理健康中的应用落后于其较早的同龄人的身体健康,但是,近年来,我们看到了应用于心理健康治疗各个方面的研究Swift增长。
Mental health is a large industry in its own right and machine learning research within the mental health domain has been applied to a vast array of topics, including medication treatment [2, 4, 5, 6], clinical diagnosis [4, 7], psychotherapy outcomes [8], and even predicting the onset of severe mental illness [9]. More specifically, the aforementioned applications of machine learning to mental health often focus on a particular diagnostic group, and sometimes even get as granular as a specific treatment modality within that psychiatric disorder. The most prevalent diagnostic group in current machine learning research is unsurprisingly also the most prevalent of mental health disorders, depression. In the United States alone, it is estimated that over 17 million adults had at least one major depressive episode in 2017, a whopping 7% of the population [10].
心理健康本身就是一个大行业,并且在心理健康领域内的机器学习研究已应用于众多主题,包括药物治疗[2,4,5,6],临床诊断[4,7],心理治疗的结果[8],甚至可以预测严重精神疾病的发作[9]。 更具体地说,机器学习在心理健康方面的上述应用通常侧重于特定的诊断组,有时甚至与该精神病性疾病中的特定治疗方式一样。 毫不奇怪,当前机器学习研究中最普遍的诊断人群也是精神疾病,抑郁症。 仅在美国,据估计,2017年有超过1700万成年人患有至少一次严重的抑郁症,占人口的7% [10]。
Although research on machine learning applications in depression is not new, they have only recently started gaining significant traction. A bibliographic analysis of all papers related to the topic of machine learning in depression [3] found that the first paper was published in 1993. The 2nd, however, wasn’t published until 1999, after which slow but steady growth was evident each year. More recently we have seen a relative boom in research on machine learning in depression, with exponential growth particularly evident in the past three years. Given this trajectory, it is safe to assume we are still in the early stages of machine learning research as it applies to depression. It is a promising and exciting field of study with numerous facets of discovery still unfolding before our eyes.
尽管对机器学习在抑郁症中的应用的研究并不新鲜,但直到最近才开始获得重要的关注。 对与抑郁症中的机器学习有关的所有论文的书目分析[3]发现,第一篇论文于1993年发表。然而,第二篇论文直到1999年才发表,此后每年都有缓慢但稳定的增长。 。 最近,我们看到抑郁症中机器学习的研究相对蓬勃发展,在过去三年中,指数式增长尤为明显。 考虑到这一轨迹,可以肯定地认为我们仍处于机器学习研究的早期阶段,因为它适用于抑郁症。 这是一个充满希望的令人兴奋的研究领域,众多的发现面还在我们眼前展现。
目前的应用 (Current Applications)
One of the most prominent and pervasive applications of machine learning in depression has focused on medication treatment outcomes [2, 4, 5, 6, 11, 12]. Indeed, a journal search on machine learning in depression will return a majority of papers specifically focused on psychotropic medication treatment. One prominent study combined clinical data from nine previous depression studies and used machine learning to cluster associated symptoms, and subsequently build a machine learning model to evaluate the efficacy of several major antidepressant medications [2]. The results identified three general symptom clusters and found statistically significant differences between the effectiveness of each antidepressant studied, suggesting that the specific cluster of symptoms a patient is experiencing should inform which antidepressant is prescribed.
机器学习在抑郁症中最突出和最普遍的应用之一集中于药物治疗的结果[2、4、5、6、11、12]。 实际上,有关抑郁症机器学习的期刊搜索将返回大部分专门针对精神药物治疗的论文。 一项重要的研究结合了先前9项抑郁症研究的临床数据,并使用机器学习对相关症状进行聚类,随后建立了机器学习模型来评估几种主要抗抑郁药的疗效[2]。 结果确定了三个一般症状群,并且发现每种研究的抗抑郁药的有效性之间在统计学上有显着差异,这表明患者所经历的特定症状群应告知应使用哪种抗抑郁药。
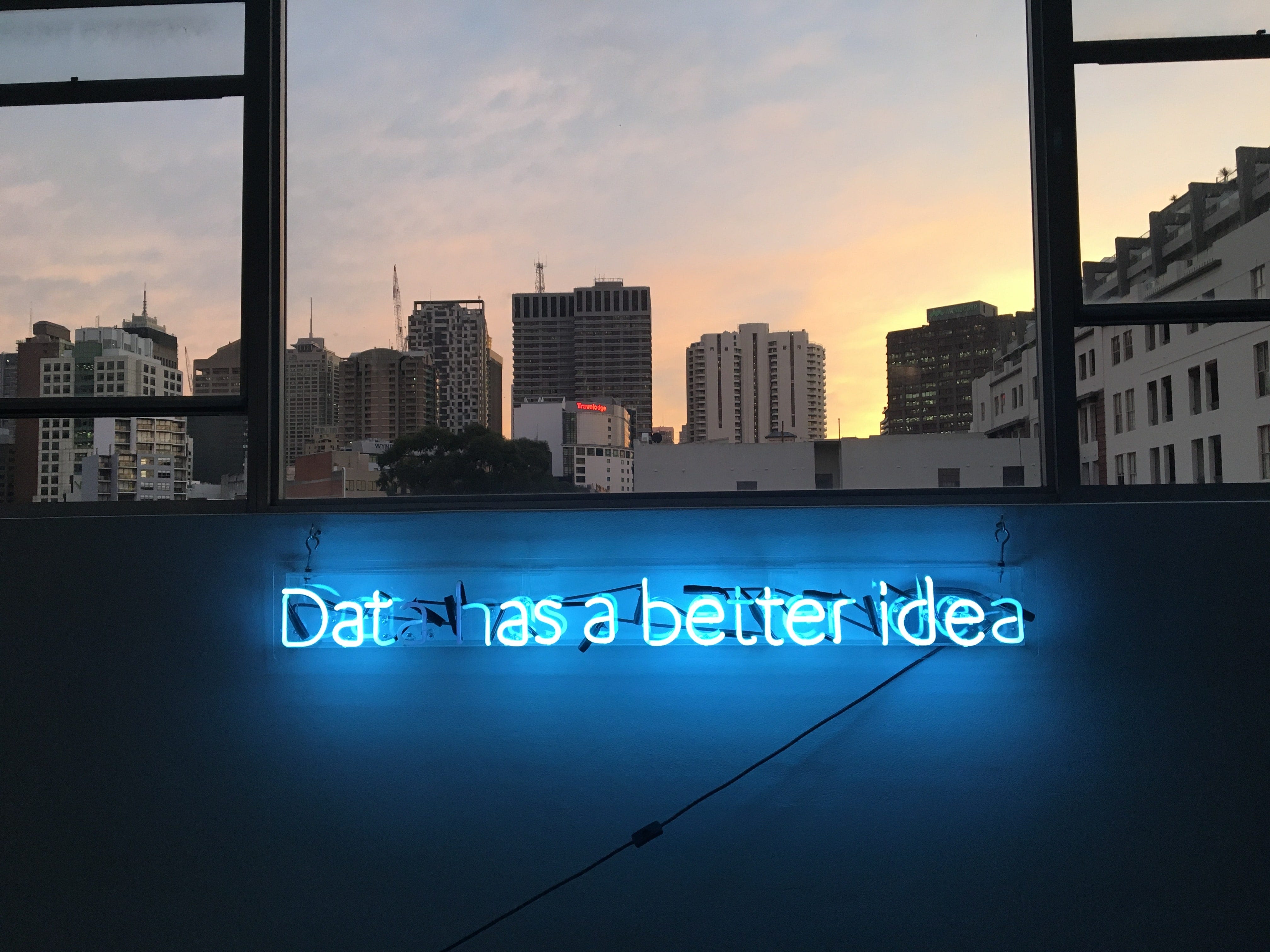
Specific psychological assessment tools, including cognitive, psychomotor, and emotional tests, have also been used to cluster outcomes [5]. These clusters were then used to predict psychiatric medication response and found that certain biomarkers were linked to success based on the antidepressant medication prescribed.
特定的心理评估工具,包括认知,心理运动和情绪测验,也已被用于聚类结果[5]。 然后将这些聚类用于预测精神科药物的React,并发现根据处方的抗抑郁药,某些生物标志物与成功相关。
Machine learning has also been applied to the topic of antidepressant symptom remission after an initial medication protocol [6, 10], which is a prominent, recurring issue in the treatment of depressive disorders (with medication). Machine learning models were trained on clinical assessment data to classify successful remission (after 12 weeks) across three different antidepressant medications [6]. Results indicated that the 164 clinical features analyzed were able to predict remission for two of the three medication protocols with 60% accuracy.
最初的药物治疗方案后,机器学习也已被用于抗抑郁症状缓解的主题[6,10],这是治疗抑郁症(药物治疗)中反复出现的重要问题。 在临床评估数据上训练了机器学习模型,以对三种不同的抗抑郁药物的成功缓解(12周后)进行分类[6]。 结果表明,分析的164种临床特征能够以60%的准确性预测三种药物治疗方案中的两种的缓解情况。
Although less common in the literature, machine learning has also been applied to other forms of treatment outcomes (apart from medication) in depression treatment. There are two other categories of depression treatment data that stand out, psychotherapy outcomes [8] and imaging data (e.g., Magnetic Resonance Imaging (MRI) scans) [10]. The first meta-analysis on the use of machine learning to predict treatment outcomes in unipolar and bipolar depression evaluated all forms of depression treatment data, including psychotherapy [8]. After initial abstract analysis of 639 potential studies, 75 received full-text review, and 26 met criteria for inclusion meaning it utilized machine learning algorithms to predict depression treatment outcomes. Results generally supported the usefulness of machine learning in predicting treatment outcomes with a combined 82% success rate (p < .05), and indicated algorithms using multiple data types were most effective. When decision trees were trained specifically on MRI data to classify the remission rate after 8 weeks of initial antidepressant treatment, it was found that MRI’s could successfully identify a subset of patients that were likely non-responders to initial antidepressant treatment [10].
尽管在文献中较少见,但是机器学习也已应用于抑郁治疗中的其他形式的治疗结果(除药物治疗之外)。 还有另外两类抑郁症治疗数据非常突出:心理治疗结果[8]和影像数据(例如,磁共振成像(MRI)扫描)[10]。 关于使用机器学习预测单相和双相抑郁的治疗效果的首次荟萃分析评估了各种形式的抑郁治疗数据,包括心理治疗[8]。 在对639项潜在研究进行初步抽象分析之后,有75篇获得了全文审查,有26篇符合纳入标准,这意味着它利用机器学习算法来预测抑郁症的治疗效果。 结果通常支持机器学习在预测治疗结果方面的有用性,综合成功率为82%(p <.05),并且表明使用多种数据类型的算法最为有效。 当针对MRI数据对决策树进行专门训练以对初始抗抑郁药治疗8周后的缓解率进行分类时,发现MRI可成功识别出可能对初始抗抑郁药无React的部分患者[10]。
Another promising application of machine learning in depression, and also more broadly to all mental health diagnoses, involves utilizing statistics and modeling to redefine current symptom clusters and diagnostic groupings [4]. This would be a vast undertaking and likely encounter significant pushback from decades of the status quo, which has always defined diagnostic groupings based on theoretical categories of psychiatric diagnoses that do not always line up to real-world manifestations of mental illness symptoms. Potential benefits include improved disease identification leading to the development of more effective interventions and medications, and a subsequent decrease in the overwhelming economic and societal cost of mental illness.
机器学习在抑郁症以及更广泛地用于所有精神健康诊断中的另一有希望的应用,涉及利用统计和建模来重新定义当前的症状群和诊断组[4]。 这将是一项艰巨的任务,并且可能会因数十年来的现状而遭受重大挫败,因为数十年来,这种状态始终根据精神病诊断的理论类别来定义诊断分组,而这些理论类别并不总是与现实世界中精神疾病症状的表现相吻合。 潜在的好处包括改善疾病识别,从而导致开发更有效的干预措施和药物,以及随后减少精神疾病的巨大经济和社会成本。
Considering the likely backlash against a complete revamp of diagnostic categorizations, a compromise approach was suggested that combines data-driven machine learning with theory-driven models [7]. More specifically, in this approach the theoretical models inform the feature selection process, leaning out the number of variables fed into machine learning algorithms. There are examples of this type of methodology improving outcomes in other medical/neurological diseases (e.g., Parkinson’s), and thus applying a similar approach to mental illness could improve both diagnosis and treatment outcomes.
考虑到对诊断分类的彻底改造可能产生的抵制,有人提出了一种折衷的方法,将数据驱动的机器学习与理论驱动的模型相结合[7]。 更具体地说,在这种方法中,理论模型通过减少输入到机器学习算法中的变量的数量来指导特征选择过程。 有这种类型的方法可以改善其他医学/神经疾病(例如帕金森氏病)结局的实例,因此对精神疾病采用类似的方法可以改善诊断和治疗结局。
Despite the rapidly growing body of promising research in the application of machine learning to depression, there are some underlying practical and ethical concerns that must be considered.
尽管将机器学习应用于抑郁症的研究Swift发展,但仍需要考虑一些潜在的实践和伦理问题。
Some of the predominant practical concerns involve challenges to aggregating data from disparate sources, as well as difficulties conducting measures commonly used in studies (e.g., MRIs) on actual psychiatric patients in the real world, thus limiting the practicality of such research. Ethical considerations include assuring the patient actually wants to know if they are at risk, and the potential adverse impact and stigma associated with labeling someone with a mental illness, such as Major Depressive Disorder [9].
一些主要的实际问题涉及从不同来源收集数据的挑战,以及在现实世界中对实际精神病患者进行研究(例如MRI)中常用的措施时遇到的困难,从而限制了此类研究的实用性。 道德考量包括确保患者确实想知道自己是否有危险,以及给患有精神疾病(例如严重抑郁症)的人贴标签可能带来的潜在不良影响和污名[9]。
结论 (Conclusion)
This is an exciting time for both providers and consumers of mental health services. Novel diagnostic and treatment schemas for depressive disorders may be on the horizon as we enter the brave new world of machine learning research and learn how best to apply it to our field. The current exponential growth in research in this area is a testament to the potential impact machine learning could have on mental health care. At the same time, we have only scratched the surface of what is possible. We are starting to see shifts in decades-old mentalities on how best to treat psychiatric disorders, to the extent that even the diagnostic groupings themselves are being put to the test.
对于精神卫生服务的提供者和消费者而言,这是一个令人兴奋的时刻。 随着我们进入机器学习研究这个勇敢的新世界,并学习如何最好地将其应用于我们的领域,可能会出现针对抑郁症的新型诊断和治疗方案。 目前该领域研究的指数增长证明了机器学习对心理保健的潜在影响。 同时,我们只是摸索了一切的可能性。 我们已经开始看到数十年来关于如何最好地治疗精神疾病的观念发生了变化,以至于甚至对诊断组本身也进行了测试。
In terms of depression treatment specifically, we are seeing machine learning successfully applied to improve antidepressant outcomes, reduce remission rates, and better classify groups that respond to specific medications. Multiple disparate sources of data are being used to improve these treatment outcomes, including psychological and cognitive testing, as well as MRI scans and other imaging techniques. Additionally, machine learning techniques are being applied to specific psychotherapy modalities to treat depression in an attempt to improve outcomes and identify patients and symptom presentations that best respond to particular types of therapy.
具体而言,在抑郁症治疗方面,我们看到机器学习已成功应用于改善抗抑郁效果,降低缓解率以及更好地对对特定药物有React的人群进行分类。 多种不同的数据来源正在用于改善这些治疗效果,包括心理和认知测试以及MRI扫描和其他成像技术。 此外,机器学习技术已应用于特定的心理治疗方式,以治疗抑郁症,以改善治疗效果并确定对特定治疗类型最有效的患者和症状表现。
Future research will likely continue along this trajectory, as the validity and reliability of this current foundation are tested and subsequently built upon. Considering the relative lack of machine learning research currently applied to psychotherapy, and in light of the fact that therapy is one of the most common and successful longer-term treatments for depression (on par with medication treatment), I suspect we will start to see a surge in machine learning research in this area. Medication outcomes, however, are easier to conceptualize and test, and overlap more with longer-established medical research, and thus will likely continue to receive outsized attention. Overall, there is a bright future for machine learning applications in the treatment of depression.
随着对当前基础的有效性和可靠性进行测试并在此基础上继续进行,未来的研究可能会沿着这一轨迹继续进行。 考虑到目前相对较缺乏用于心理治疗的机器学习研究,并且鉴于治疗是抑郁症最常见,最成功的长期治疗方法之一(与药物治疗相当),我怀疑我们将开始看到该领域的机器学习研究激增。 但是,药物治疗的结果更容易概念化和测试,并且与历史悠久的医学研究有更多的重叠,因此可能会继续受到极大的关注。 总体而言,机器学习在抑郁症治疗中的应用前景广阔。
✍️ Subscribe to get my newest articles, featured in publications like The Startup & Towards Data Science ➡️
Subscribe️ 订阅以获得我的最新文章,并在The Startup&Towards Data Science➡️ 等出版物中发表
[1] Abhari, S., Kalhori, S., Ebrahimi, M., Hasannejadasl, H., Garavand, A. “Artificial intelligence applications in type 2 diabetes mellitus care: focus on machine learning methods.” Healthcare Informatics Research, vol. 25, no. 4, p. 248, 2019.
[1] Abhari,S.,Kalhori,S.,Ebrahimi,M.,Hasannejadasl,H.,Garavand,A.“在2型糖尿病护理中的人工智能应用:专注于机器学习方法。” 医疗保健信息学研究,第一卷。 25号 4,第 248,2019。
[2] Chekroud, A., Gueorguieva, R., Krumholz, H., Trivedi, M., Krystal, J., McCarthy, G. “Reevaluating the efficacy and predictability of antidepressant treatments: a symptom clustering approach.” JAMA Psychiatry, vol. 74, no. 4, p. 370, 2017.
[2] Chekroud,A.,Gueorguieva,R.,Krumholz,H.,Trivedi,M.,Krystal,J.,McCarthy,G。“重新评估抗抑郁药的疗效和可预测性:一种症状聚类方法。” JAMA精神病学,第1卷。 74号 4,第 370,2017。
[3] Tran, B., McIntyre, R., Latkin, C., Phan, H., Vu, G., Nguyen, H., Gwee, K., Ho, C., Ho, R. “The current research landscape on the artificial intelligence application in the management of depressive disorders: a bibliometric analysis.” International Journal of Environmental Research and Public Health, vol. 16, no. 12, p. 2150, 2019.
[3] Tran,B.,McIntyre,R.,Latkin,C.,Phan,H.,Vu,G.,Nguyen,H.,Gwee,K.,Ho,C.,Ho,R。抑郁症管理中人工智能应用的研究前景:文献计量分析。” 国际 环境研究与公共卫生杂志,第一卷。 16号 第十二页 2150,2019。
[4] Bzdok, D., Meyer-Lindenberg, A. “Machine learning for precision psychiatry: opportunities and challenges.” Biological Psychiatry: Cognitive Neuroscience and Neuroimaging, vol. 3, no. 3, p. 223, 2017.
[4] Bzdok,D.,Meyer-Lindenberg,A。“为精准精神病学学习机器:机遇与挑战。” 生物精神病学:认知神经科学和神经影像学,第1卷。 3号 3,第 223,2017。
[5] Etkin, A., Patenaude, B., Song, Y., Usherwood, T., Rekshan, W., Schatzberg, A., Rush, A., Williams, L. “A cognitive-emotional biomarker for predicting remission with antidepressant medications: A report from the iSPOT-D trial.” Neuropsychopharmacology, vol. 40, p. 1332, 2015.
[5] Etkin,A.,Patenaude,B.,Song,Y.,Usherwood,T.,Rekshan,W.,Schatzberg,A.,Rush,A.,Williams,L.“用于预测的认知情感生物标记抗抑郁药的缓解:iSPOT-D试验的报告。” 神经心理药理学卷 第40页 1332,2015年。
[6] Chekroud, A., Zotti, R., Shehzad, Z., Gueorguieva, R., Johnson, M., Trivedi, M., Cannon, T., Krystal, J., Corlett, P. “Cross-trial prediction of treatment outcome in depression: A machine learning approach.” The Lancet Psychiatry, vol. 3, no. 3, p. 243, 2016.
[6] Chekroud,A.,Zotti,R.,Shehzad,Z.,Gueorguieva,R.,Johnson,M.,Trivedi,M.,Cannon,T.,Krystal,J.,Corlett,P.“ Cross-抑郁症治疗结果的临床试验预测:一种机器学习方法。” 柳叶刀精神病学,卷。 3号 3,第 243,2016年。
[7] Huys, Q., Maia, T., Frank, M. “Computational psychiatry as a bridge from neuroscience to clinical applications.” Nature Neuroscience, vol. 19, no. 3, p. 404, 2016.
[7] Huys,Q.,Maia,T.,Frank,M.“计算精神病学是从神经科学到临床应用的桥梁。” 自然神经科学,第一卷。 19号 3,第 404,2016年。
[8] Lee, Y., Ragguett, R., Mansur, R., Boutilier, J., Rosenblat, J., Trevizol, A., Brietzke, E., Lin, K., Pan, Z., Subramaniapillai, M., Chan, T., Fus, D., Park, C., Musial, N., Zuckerman, H., Chen, V., Ho, R., Rong, C., McIntyre, R. “Applications of machine learning algorithms to predict therapeutic outcomes in depression: A meta-analysis and systematic review.” Journal of Affective Disorders, vol. 241, no. 208, p. 519, 2018.
[8] Lee,Y.,Ragguett,R.,Mansur,R.,Boutilier,J.,Rosenblat,J.,Trevizol,A.,Brietzke,E.,Lin,K.,Pan,Z.,Subramaniapillai, M.,Chan,T.,Fus,D.,Park,C.,Musial,N.,Zuckerman,H.,Chen,V.,Ho,R.,Rong,C.,McIntyre,R.机器学习算法预测抑郁症的治疗效果:荟萃分析和系统评价。” 情感障碍杂志,第一卷。 241号 第208页 519,2018。
[9] Lawrie, S., Fletcher-Watson, S., Whalley, H., McIntosh, A. “Predicting major mental illness: ethical and practical considerations.” BJPsych Open, vol. 5, no. 2, p. 30, 2019.
[9] S. Lawrie,S。Fletcher-Watson,H。Whalley,A。McIntosh,“预测重大精神疾病:伦理和实践考虑。” BJPsych公开赛,第一卷。 5号 2,第 2019年30月30日。
[10] National Institute of Mental Health, “Major depression: Prevalence of major depressive episode among adults” nimh.nih.gov, section 2, 2007. [Online]. Available: https://www.nimh.nih.gov/health/statistics/major-depression.shtml. [Accessed: Apr. 27, 2020].
[10]国家心理健康研究所,“严重抑郁症:成年人严重抑郁发作的患病率”, nimh.nih.gov ,第2部分,2007年。[在线]。 可用: https : //www.nimh.nih.gov/health/statistics/major-depression.shtml 。 [访问:2020年4月27日]。
[11] Korgaonkar, M., Rekshan, W., Gordon, E., Rush, A., Williams, L., Blasey, C., Grieve, S. “Magnetic resonance imaging measures of brain structure to predict antidepressant treatment outcome in major depressive disorder.” EBioMedicine, vol. 2, no. 1, p. 37, 2015.
[11] M. Korgaonkar,W。Rekshan,Gordon,E。Rush,A.,Williams,L.,Blasey,C.,Grieve,S。“脑结构的磁共振成像测量可预测抗抑郁治疗的结局在严重的抑郁症中。” EBioMedicine,第1卷。 2,没有 1页 2015年3月37日。
[12] Mehltretter, J., Rollins, C., Benrimoh, D., Fratila, R., Perlman, K., Israel, S., Miresco, M., Wakid, M., Turecki, G. “Analysis of features selected by a deep learning model for differential treatment selection in depression.” Frontiers in Artificial Intelligence, vol. 2, p. 31, 2020.
[12] Mehltretter,J.,Rollins,C.,Benrimoh,D.,Fratila,R.,Perlman,K.,Israel,S.,Miresco,M.,Wakid,M.,Turecki,G.“分析深度学习模型选择的功能用于抑郁症的差异治疗选择。” 人工智能前沿 。 2,第 2020年3月31日。
翻译自: https://medium.com/swlh/machine-learning-in-the-treatment-of-depression-87dcd63f528d
机器学习与分布式机器学习
http://www.taodudu.cc/news/show-4522470.html
相关文章:
- 大数据 端到端_成为数据科学家的端到端指南
- 神码ai人工智能写作机器人_人工智能和机器学习可以改善营销的6种方式
- 计算机与计算机网络_让计算机承担责任
- 数据 术语_这5个必须知道的数据科学家进入零售领域的术语
- 基于树的模型的更好功能
- 向前logistic回归与向后筛选出一样的变量_了解逻辑回归系数
- 怎样避免无意识偏见_精神病学意识到大数据和人工智能的价值和偏见
- 根据一堆数字判定下一个数字_坐在一堆数字黄金
- 为什么某些Win32技术在Windows NT服务中行为不当?
- 头一回见!提升10倍效率,阿里给业务校验平台插上了AI的翅膀
- C++——NOIP提高组——飞扬的小鸟
- luogu P1941 飞扬的小鸟
- NOIP 2014 飞扬的小鸟
- 【NOIP2014提高组】飞扬的小鸟
- luogu1941 飞扬的小鸟
- codevs 3729 飞扬的小鸟
- 比较两个Integer的值是否相等
- 投资理财-言微不劝人
- 计算机组成原理——微指令格式
- 微前端之 qiankun 入门、上手、实战(构建大型 web 应用)
- 2022年 微前端技术调研- 图文并茂
- 微商加人的24种方法微商怎么加人怎么做
- 鸿蒙使用linux内核微内核,浅谈鸿蒙操作系统的微内核
- 微电子电路——反相器网表详解
- 双11付费专栏9折优惠
- 阿里云9折优惠码,省一个月的钱!!! 优惠码:jtlvp4
- natapp邀请码,新用户购买域名可以享受9折优惠
- c语言输入三个商品的价格,若有一个大于100元或者总价大于200元,全部商品打9折,并完成付款和找零
- 阿里云推荐码 9折优惠 vx89to
- ETC通行费9折活动
机器学习与分布式机器学习_机器学习治疗抑郁症相关推荐
- 机器学习线性回归学习心得_机器学习中的线性回归
机器学习线性回归学习心得 机器学习中的线性回归 (Linear Regression in Machine Learning) There are two types of supervised ma ...
- 机器学习中qa测试_机器学习项目测试怎么做?(看实例)
机器学习交付项目通常包含两部分产物,一部分是机器学习模型,另一部分是机器学习应用系统.机器学习模型是嫁接在应用之上产生价值的.比如:一款预测雷雨天气的APP,它的雷雨预测功能就是由机器学习模型完成的. ...
- 机器学习解决什么问题_机器学习帮助解决水危机
机器学习解决什么问题 According to Water.org and Lifewater International, out of 57 million people in Tanzania, ...
- 机器学习回归模型精度_机器学习模型实施精度召回和概率截止。
机器学习回归模型精度 Congratulations on completing your Machine Learning (ML) pipeline! In the second part of ...
- 机器学习朴素贝叶斯_机器学习基础朴素贝叶斯分类
机器学习朴素贝叶斯 In the previous stories, I had given an explanation of the program for implementation of v ...
- 机器学习模型 知乎_机器学习:模型评估之评估方法
机器学习已经成为了人工智能的核心研究领域之一,它的研究动机就是为了让计算机系统具有人的学习能力以便实现人工智能.目前,关于机器学习定义的说法比较多,而被广泛采用的定义是"利用经验来改善计算 ...
- 机器学习中qa测试_机器学习自动化单元测试平台
机器学习自动化单元测试平台.零代码.全方位.自动化测试方法/函数的正确性和可用性. 原理 后端不需要写任何单元测试代码(逻辑代码.注解代码等全都不要), 这个工具会自动生成测试参数,并执行方法,拿到返 ...
- 机器学习模型定点化_机器学习模型的超参数优化
引言 模型优化是机器学习算法实现中最困难的挑战之一.机器学习和深度学习理论的所有分支都致力于模型的优化. 机器学习中的超参数优化旨在寻找使得机器学习算法在验证数据集上表现性能最佳的超参数.超参数与一般 ...
- 机器学习模型 知乎_机器学习-模型选择与评价
交叉验证 首先选择模型最简单的方法就是,利用每一种机器学习算法(逻辑回归.SVM.线性回归等)计算训练集的损失值,然后选择其中损失值最小的模型,但是这样是不合理的,因为当训练集不够.特征过多时容易过拟 ...
- 机器学习里面的基函数_机器学习系列(一)——基础概念及分类
机器学习基础(一) emm... 那个第一篇文章,简单的自我介绍一下,机器学习小白,希望和大家一起进步,有什么问题可以留言一起探讨. 本文撰写于2020/1/1 是本人学习机器学习时,做的笔记摘要,为 ...
最新文章
- Batch Size对神经网络训练的影响
- printf输出字符串的一些格式
- 关于文件的默认读取和保存路径(转)
- 2 字符串String
- oralce10g中约束与列属性NULLABLE的关系
- Java分布式系统高并发解决方案
- android 不同型号的终端的UI适配--一种解决方法
- equals, hashCode, toString方法重写,深入探究equals
- springboot实现条形码_Springboot转发重定向实现方式解析
- js获取可视区域高度
- java 操作日志记录_高效日志系统搭建秘技!架构师必读
- 趣谈网络协议栈,以太网基础MAC和PHY
- c 语言ifelse语句的用法,C 语言 if...else 语句
- 计算机上什么键有存储,计算器存储运算键是什么?
- html电子贺卡国庆,中秋贺卡设计欣赏 电子贺卡制作 中秋电子贺卡模板
- php 应用截图,PHP应用:php使用CutyCapt实现网页截图保存的方法
- 散列表--双散列、再散列与可扩散列
- 【云服务器 ECS 实战】专有网络 VPC、弹性网卡的概述与配置
- java runnable main_【BUG】”main” prio=5 tid=1 RUNNABLE
- 电大计算机毕业论文任务书范文,广播电视大学毕业设计任务书表格.doc