sql server作业_SQL Server作业性能–报告
sql server作业
描述 (Description)
Once collected, job performance metrics can be used for a variety of reporting needs, from locating jobs that are not performing well to finding optimal release windows, scheduling maintenance, or trending over time. These techniques allow us to maintain insight into parts of SQL Server that are often not monitored enough and prevent job-related emergencies before they become emergencies.
一旦收集了工作绩效指标,就可以将其用于各种报告需求,从查找表现不佳的工作到找到最佳发布窗口,安排维护或随时间推移而趋向。 这些技术使我们能够深入了解通常未受到足够监控SQL Server部分,并在与工作有关的紧急情况发生之前将其预防。
介绍 (Introduction)
Collecting job performance metrics provides us with the opportunity to then report on that data. In that realm, our imaginations are the only limiting factor.
收集工作绩效指标为我们提供了机会,然后报告该数据。 在这个领域,我们的想象力是唯一的限制因素。
We can create further fact tables to store aggregated metrics, perform gaps/islands analysis in order to find optimal job times or busy times, and ready data for consumption by reporting tools, such as SSRS or Power BI.
我们可以创建更多的事实表来存储汇总指标,执行差距/孤岛分析以找到最佳的工作时间或繁忙时间,并准备好数据供报告工具(例如SSRS或Power BI)使用。
Using these tools & metrics, we can look at past data, in order to observe trends and forecast future job runtimes, allowing us to solve a performance problem before it becomes serious. We can use this data to alert on rogue jobs, or those that are performing well out of their typical boundaries. Data can be compared between servers or environments to hunt for differences that may indicate other unrelated processes that are not running optimally, or compare different hardware configurations on job performance.
使用这些工具和指标,我们可以查看过去的数据,以便观察趋势并预测未来的工作时间,从而使我们能够在性能问题变得严重之前解决它。 我们可以使用这些数据来提醒流氓作业或性能超出其典型边界的作业。 可以在服务器或环境之间比较数据,以寻找差异,这些差异可能表明其他不相关的进程运行不佳,或者在工作性能上比较了不同的硬件配置。
The list of applications can continue for quite a while, and will vary depending on how you use SQL Server Agent, and the volume of jobs you create.
应用程序列表可能会持续一段时间,并且会因您使用SQL Server代理的方式以及创建的作业量而异。
SQL Server代理作业 (SQL Server Agent Job)
Previously, we have completed a script that can populate our metrics tables and clean them up as needed. We’ll encapsulate this TSQL into a stored procedure: dbo.usp_get_job_execution_metrics. This stored procedure, as well as all table & index creation scripts, can be downloaded at the end of this article.
以前,我们已经完成了一个脚本,该脚本可以填充指标表并根据需要进行清理。 我们将此TSQL封装到一个存储过程中: dbo.usp_get_job_execution_metrics 。 可以在本文结尾处下载此存储过程以及所有表和索引创建脚本。
To run this regularly, I’ll place the stored procedure execution into its own SQL Server Agent job, with a single step. Deletion of old data can be moved into an independent step if desired, but to keep things simple, I’ve opted to keep it in the main stored procedure. The job looks like this:
为了定期运行此程序,我将通过一个步骤将存储过程执行放入其自己SQL Server代理作业中。 如果需要,可以将删除旧数据移到一个独立的步骤中,但是为了简化起见,我选择将其保留在主存储过程中。 工作看起来像这样:
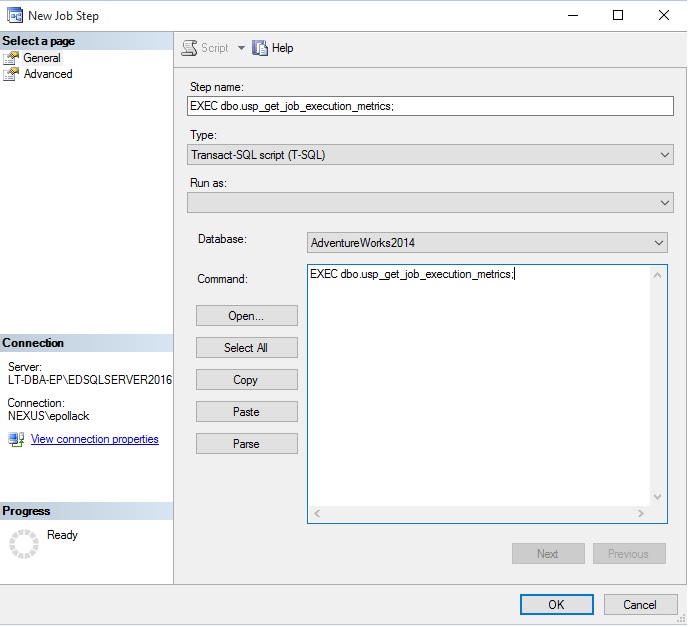
The advanced tab indicates that the job will complete and report success after the one (and only) step completes:
“高级”选项卡指示一个(唯一)步骤完成后,作业将完成并报告成功:
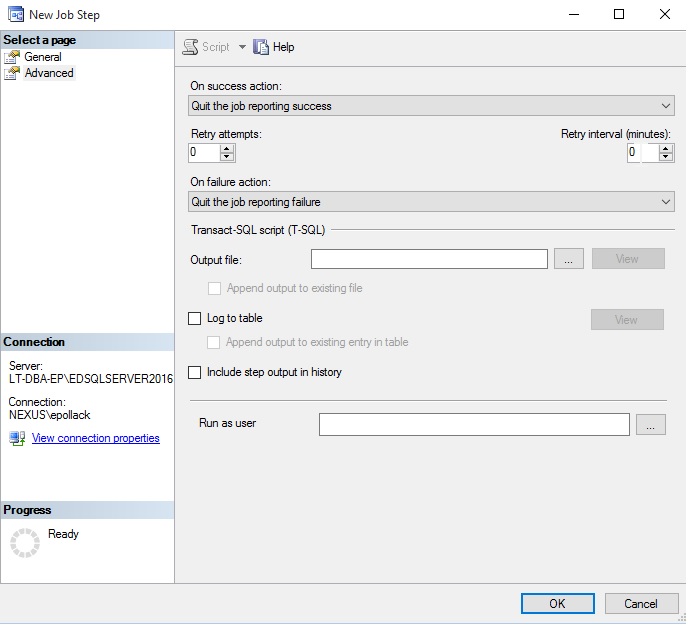
The schedule for this job is set to run it every 15 minutes. Feel free to adjust as needed based on the frequency of job executions and metrics needs on your system:
该作业的时间表设置为每15分钟运行一次。 可以根据系统上作业的执行频率和指标需求随时进行调整:
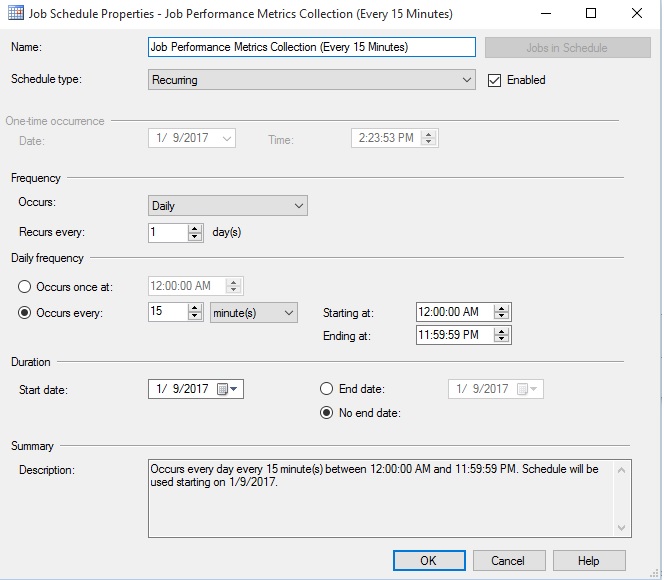
At this point, we have job collection tables, a collection stored procedure, and a job that can run regularly to collect and update our data. The last step is to consider how we will report on this data, and build the appropriate solution to present this data to us in a meaningful fashion!
至此,我们有了作业收集表,收集存储过程以及可以定期运行以收集和更新数据的作业。 最后一步是考虑如何对这些数据进行报告,并构建适当的解决方案以有意义的方式向我们展示这些数据!
报告工作绩效指标 (Reporting on Job Performance Metrics)
We’re now collecting job metrics, which can be very useful for monitoring and validating job history, but we can do much more with this data. To illustrate this, we will walk through a variety of metrics, showing how they can be calculated and returned to a user, report, or dashboard. The final version of this is included in a stored procedure that is attached to this article.
现在,我们正在收集作业指标,这对于监视和验证作业历史记录非常有用,但是我们可以用这些数据做更多的事情。 为了说明这一点,我们将遍历各种指标,展示如何计算它们并将其返回给用户,报告或仪表板。 本文所附的存储过程中包含此程序的最终版本。
Now that we are ready to go, let’s consider a handful of metrics to report on:
现在我们准备好开始了,让我们考虑一些报告指标:
- Allows us to trend job performance over time in order to find patterns that require attention. 使我们能够随时间变化工作绩效,以便找到需要关注的模式。
- Useful for scheduling new jobs based on existing schedules. 用于根据现有计划安排新作业。
- Helps in planning downtime and understand when quiet times are. 帮助计划停机时间并了解安静时间。
- Catch problems before they become critical. 在问题变得严重之前就抓住它们。
作业平均运行时间 (Job Runtime Averages)
To facilitate the collection of this data, we can create a new table to store these aggregated metrics:
为了便于收集这些数据,我们可以创建一个新表来存储这些汇总指标:
CREATE TABLE dbo.fact_daily_job_runtime_metrics( fact_daily_job_runtime_metrics_ID INT NOT NULL IDENTITY(1,1) CONSTRAINT PK_fact_daily_job_runtime_metrics PRIMARY KEY CLUSTERED,Sql_Agent_Job_Id UNIQUEIDENTIFIER NOT NULL,Job_Run_Date DATE NOT NULL,Job_Run_Count INT NOT NULL,Job_Run_Failure_Count INT NOT NULL,Job_Run_Time_Minimum INT NULL,Job_Run_Time_Maximum INT NULL,Job_Run_Time_Average INT NULL);CREATE NONCLUSTERED INDEX IX_fact_daily_job_runtime_metrics_Sql_Agent_Job_Id ON dbo.fact_daily_job_runtime_metrics (Sql_Agent_Job_Id);
CREATE NONCLUSTERED INDEX IX_fact_daily_job_runtime_metrics_Job_Run_Date ON dbo.fact_daily_job_runtime_metrics (Job_Run_Date);
With this table created, we can add some TSQL to our stored procedure to populate it:
创建此表后,我们可以向存储过程中添加一些TSQL来填充它:
DECLARE @Last_Complete_Averages_Collection_Date DATE;SELECT@Last_Complete_Averages_Collection_Date = MAX(fact_daily_job_runtime_metrics.Job_Run_Date)FROM dbo.fact_daily_job_runtime_metrics;SELECT @Last_Complete_Averages_Collection_Date = ISNULL(@Last_Complete_Averages_Collection_Date, '1/1/1900');DELETE fact_daily_job_runtime_metricsFROM dbo.fact_daily_job_runtime_metricsWHERE fact_daily_job_runtime_metrics.Job_Run_Date >= @Last_Complete_Averages_Collection_Date;INSERT INTO dbo.fact_daily_job_runtime_metrics(Sql_Agent_Job_Id, Job_Run_Date, Job_Run_Count, Job_Run_Failure_Count, Job_Run_Time_Minimum, Job_Run_Time_Maximum, Job_Run_Time_Average)SELECTfact_job_run_time.Sql_Agent_Job_Id,CAST(fact_job_run_time.Job_Start_Datetime AS DATE) AS Job_Run_Date,COUNT(*) AS Job_Run_Count,SUM(CASE WHEN fact_job_run_time.Job_Status = 'Failure' THEN 1 ELSE 0 END) AS Job_Run_Failure_Count,MIN(fact_job_run_time.Job_Duration_Seconds) AS Job_Run_Time_Minimum,MAX(fact_job_run_time.Job_Duration_Seconds) AS Job_Run_Time_Maximum,AVG(fact_job_run_time.Job_Duration_Seconds) AS Job_Run_Time_AverageFROM dbo.fact_job_run_timeWHERE fact_job_run_time.Job_Start_Datetime >= @Last_Complete_Averages_Collection_DateGROUP BY fact_job_run_time.Sql_Agent_Job_Id, CAST(fact_job_run_time.Job_Start_Datetime AS DATE);
Note that we remove an extra day of data prior to population that is from the last populated date. This is done as a safeguard against incomplete data, as we will need to recalculate averages on any data that is still being updated at the time of the job run. For reporting convenience, the job_id can be replaced with the job name, if desired.
请注意,从上次填充日期开始,我们将在填充之前删除额外的一天数据。 这样做是为了防止数据不完整,因为我们将需要重新计算在作业运行时仍在更新的任何数据的平均值。 为了方便报告,如果需要,可以用作业名称替换job_id 。
The result of this script on my local server is a pile of job data that tells me about job executions per day per job:
此脚本在本地服务器上的结果是一堆作业数据,这些数据告诉我每个作业每天的作业执行情况:
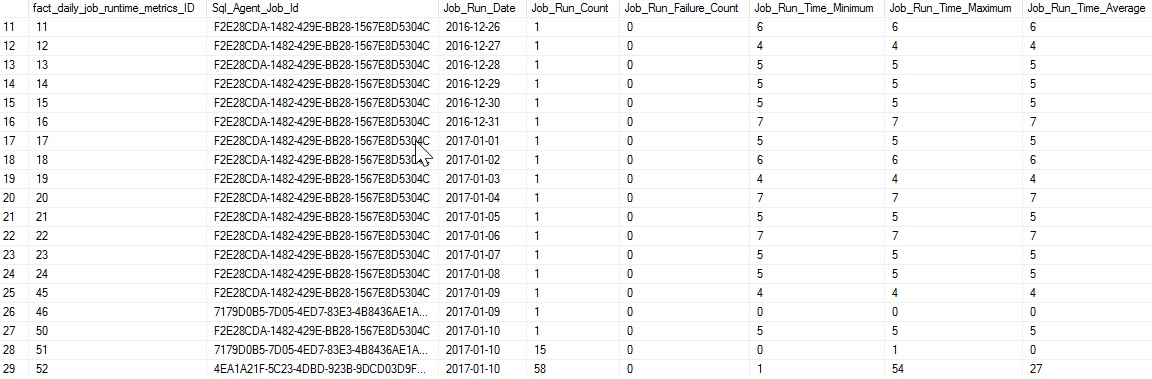
This data is aggregated by date, but could easily be updated to compute averages over a given hour, week, month, or other time period that is convenient. Similarly, we could create multiple fact tables to track metrics over multiple periods. Once data is aggregated, it may no longer be of use to you, in which case cleanup of older data can be performed more aggressively to improve performance and reduce disk usage.
此数据按日期汇总,但可以轻松更新以计算给定的小时,周,月或其他方便的时间段内的平均值。 同样,我们可以创建多个事实表来跟踪多个时期的指标。 数据汇总后,可能不再对您有用,在这种情况下,可以更积极地执行较旧数据的清理,以提高性能并减少磁盘使用率。
作业时间表详细信息 (Job Schedule Details)
Returning a short list of all job/schedule relationships from our existing tables is almost trivial, now that the data is formatted in a friendly fashion:
现在,以一种友好的方式格式化数据,从我们现有的表中返回所有作业/计划关系的简短列表几乎是微不足道的:
SELECTdim_sql_agent_job.Sql_Agent_Job_Name,dim_sql_agent_schedule.Schedule_Name,dim_sql_agent_schedule.Schedule_Occurrence,dim_sql_agent_schedule.Schedule_Occurrence_Detail,dim_sql_agent_schedule.Schedule_Frequency,fact_sql_agent_schedule_assignment.Next_Run_DatetimeFROM dbo.fact_sql_agent_schedule_assignmentINNER JOIN dbo.dim_sql_agent_jobON fact_sql_agent_schedule_assignment.Sql_Agent_Job_Id = dim_sql_agent_job.Sql_Agent_Job_IdINNER JOIN dbo.dim_sql_agent_scheduleON fact_sql_agent_schedule_assignment.Schedule_Id = dim_sql_agent_schedule.Schedule_IdWHERE dim_sql_agent_job.Is_Enabled = 1AND dim_sql_agent_job.Is_Deleted = 0AND dim_sql_agent_schedule.Is_Enabled = 1AND dim_sql_agent_schedule.Is_Deleted = 0AND CURRENT_TIMESTAMP BETWEEN dim_sql_agent_schedule.Schedule_Start_Date AND dim_sql_agent_schedule.Schedule_End_DateORDER BY fact_sql_agent_schedule_assignment.Next_Run_Datetime ASC;
The result is a list of all job/schedule pairings, along with the next run time for the job:
结果是所有作业/时间表配对的列表,以及该作业的下一个运行时间:

We could also use a gaps/islands analysis on the job runtime data in order to determine the longest stretches of time when no jobs are running. Create a Dim_Time table, first, to store a joining table of minutes throughout the day:
我们还可以对作业运行时数据使用间隙/孤岛分析,以便确定无作业运行时的最长时间段。 首先创建一个Dim_Time表,以存储全天的分钟联接表:
CREATE TABLE dbo.Dim_Time
( Dim_Time TIME CONSTRAINT PK_Dim_Time PRIMARY KEY CLUSTERED);DECLARE @Current_Time TIME = '00:00';
INSERT INTO dbo.Dim_Time(Dim_Time)
SELECT@Current_Time;SELECT @Current_Time = DATEADD(MINUTE, 1, @Current_Time);WHILE @Current_Time <= '23:59' AND @Current_Time > '00:00'
BEGININSERT INTO dbo.Dim_Time(Dim_Time)SELECT@Current_Time;SELECT @Current_Time = DATEADD(MINUTE, 1, @Current_Time);
END
This data set could be changed to break down times on seconds, hours, or other time parts, if desired, including the addition of many days/dates/years. With this data, we can join duration data for a given day to it and get a picture of job activity over the course of a day:
如果需要,可以更改此数据集以将时间分解为秒,小时或其他时间部分,包括添加许多天/日期/年。 有了这些数据,我们可以将给定日期的工时数据加入其中,并获得一天中的工作活动情况:
DECLARE @Date_to_Check DATE = '1/10/2017';
DECLARE @Number_Of_Concurrent_Jobs INT = 0;SELECTDim_Time
FROM dbo.Dim_Time
WHERE NOT EXISTS (SELECT * FROM dbo.fact_job_run_time WHERE Dim_Time.Dim_Time BETWEEN CAST(fact_job_run_time.Job_Start_Datetime AS TIME) AND CAST(fact_job_run_time.Job_End_Datetime AS TIME)AND CAST(fact_job_run_time.Job_Start_Datetime AS DATE) = @Date_to_Check)
ORDER BY Dim_Time.Dim_Time;
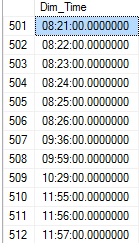
We can take this logic a bit further and analyze our set of times and report back on how many jobs run at any one time and filter accordingly. This would allow for a bit more intelligent scheduling where we could report on periods with nothing running, 1 job running, 2 jobs running, etc…Presumably, the more processes we tolerate running, the more windows of availability there will be for the scheduling of new jobs. To get a data set that shows each time (by second) and the number of concurrent jobs, we can run the following TSQL:
我们可以更进一步地研究这种逻辑,并分析一组时间,并报告一次同时运行了多少作业并进行相应过滤。 这将使调度更加智能化,在此情况下,我们可以报告没有运行,有1个作业正在运行,2个作业正在运行等的时间段……大概,我们允许运行的进程越多,调度的可用性窗口就越多新工作。 要获取显示每次(按秒)和并发作业数的数据集,我们可以运行以下TSQL:
DECLARE @Date_to_Check DATE = '1/10/2017';
WITH CTE_JOB_DATA AS (SELECTCAST(fact_job_run_time.Job_Start_Datetime AS TIME) AS Job_Start_time,CAST(fact_job_run_time.Job_End_Datetime AS TIME) AS Job_End_timeFROM dbo.fact_job_run_timeWHERE CAST(fact_job_run_time.Job_Start_Datetime AS DATE) = @Date_to_Check),
CTE_DIM_TIME AS (SELECTDim_Time,COUNT(*) AS Number_Of_Jobs_RunningFROM dbo.Dim_TimeINNER JOIN CTE_JOB_DATAON Dim_Time.Dim_Time BETWEEN CTE_JOB_DATA.Job_Start_time AND CTE_JOB_DATA.Job_End_timeGROUP BY Dim_Time)
SELECT*
INTO #Job_Run_Data
FROM CTE_DIM_TIME;SELECT * FROM #Job_Run_Data;
This script will take all times in the dim_time table and compare them to our job performance data, returning a count for each minute of the jobs running at that time, only for those times in which jobs were running:
该脚本将把所有时间都放在dim_time表中,并将它们与我们的工作绩效数据进行比较,仅针对当时正在运行的那些时间,返回当时运行的每一分钟的计数:

From here, we could constrain the results to allow for one job running (or 2, or 3) and perform an islands analysis on it in order to determine the optimal time to run a job. For this example, we’ll allow for a single running job. To facilitate a more efficient query, the results from above will be used, pulling from the temp table, rather than creating one monster query with both aggregation and islands analysis contained within:
从这里开始,我们可以将结果约束为允许一个作业运行(或2或3),并对其进行孤岛分析,以确定运行作业的最佳时间。 对于此示例,我们将允许一个正在运行的作业。 为了促进更有效的查询,将使用上面的结果,从temp表中提取结果,而不是创建一个包含聚合和孤岛分析的怪物查询:
WITH CTE_JOB_RUN_DATA AS (SELECTDim_Time.Dim_Time,ISNULL(Job_Run_Data.Number_Of_Jobs_Running, 0) AS Number_Of_Jobs_RunningFROM Dim_TimeLEFT JOIN #Job_Run_Data Job_Run_DataON Dim_Time.Dim_Time = Job_Run_Data.Dim_Time
),
ISLAND_START AS
(SELECTJob_Run_Data.Dim_Time,ROW_NUMBER() OVER(ORDER BY Job_Run_Data.Dim_Time ASC) AS Row_NumFROM CTE_JOB_RUN_DATA Job_Run_DataWHERE Job_Run_Data.Number_Of_Jobs_Running <= 1AND EXISTS (SELECT *FROM CTE_JOB_RUN_DATA Previous_RunWHERE Previous_Run.Dim_Time = DATEADD(MINUTE, -1, Job_Run_Data.Dim_Time)AND Previous_Run.Number_Of_Jobs_Running > 1)
),
ISLAND_END AS
(SELECTJob_Run_Data.Dim_Time,ROW_NUMBER() OVER(ORDER BY Job_Run_Data.Dim_Time ASC) AS Row_NumFROM CTE_JOB_RUN_DATA Job_Run_DataWHERE Job_Run_Data.Number_Of_Jobs_Running <= 1AND EXISTS (SELECT *FROM CTE_JOB_RUN_DATA Next_RunWHERE Next_Run.Dim_Time = DATEADD(MINUTE, 1, Job_Run_Data.Dim_Time)AND Next_Run.Number_Of_Jobs_Running > 1)
)
SELECTISLAND_START.Dim_Time AS Job_Time_Island_Start,ISLAND_END.Dim_Time AS Job_Time_Island_End,(SELECT COUNT(*) FROM Dim_Time Island_Time_Minutes WHERE Island_Time_ Minutes.Dim_Time BETWEEN ISLAND_START.Dim_Time AND ISLAND_END.Dim_Time) AS Island_Time_Minutes
FROM ISLAND_START
INNER JOIN ISLAND_END
ON ISLAND_START.Row_Num = ISLAND_END.Row_Num
ORDER BY ISLAND_START.Dim_Time;
The result of this query is a set of acceptable times to potentially schedule a new job, the start and end times for each window, and the length of the window (In minutes). If we didn’t have a reliable dim_time table, or a uniform increment of minutes as we do here, an additional ROW_NUMBER could be added to CTE_JOB_RUN_DATA to normalize messy data and allow for easy analysis across it. The results look like this:
该查询的结果是一组可以安排新作业的可接受时间,每个窗口的开始和结束时间以及窗口的长度(以分钟为单位)。 如果我们没有可靠的dim_time表,或者没有统一的分钟增量,则可以在CTE_JOB_RUN_DATA中添加额外的ROW_NUMBER以规范杂乱数据并对其进行轻松分析。 结果如下:
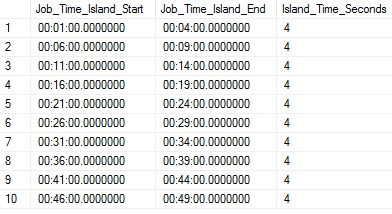
This isn’t the most useful view as we are getting lots of duplication. What we ideally want to see are the longest time windows first. To get this ordering, we can implement another CTE or put the above results into a second temp table, from where we can freely query the small result set. For this example, I’ve put the above data into a temp table called #Job_Runtime_Windows, and then run the query below:
这不是最有用的视图,因为我们正在重复很多。 我们理想地希望看到的是最长的时间窗。 为了获得这种排序,我们可以实现另一个CTE或将以上结果放入第二个临时表中,从中我们可以自由查询小的结果集。 对于此示例,我将上面的数据放入了一个名为#Job_Runtime_Windows的临时表中,然后运行以下查询:
SELECT*
FROM #Job_Runtime_Windows
ORDER BY Island_Time_Minutes DESC;
The results show a specific time frame that appears ideal for the addition of a new job:
结果显示了一个特定的时间范围,该时间范围似乎是添加新工作的理想选择:

长期和短期的工作 (Long and Short Running Jobs)
Another area of concern are jobs that run for an abnormal amount of time. To accurately alert or report on these, we need to have a fairly good idea of what is normal or not normal, both in terms of absolute and relative metrics comparisons.
另一个令人关注的领域是运行时间异常的作业。 要准确地警告或报告这些情况,就绝对指标和相对指标的比较而言,我们需要对什么是正常还是不正常有一个很好的了解。
For example, we could create a rule that states, “Any job that runs for 50% longer than its average time should be flagged as long-running”. If a job typically takes 500ms seconds to execute, and one day suddenly takes 1s, we likely won’t want an alert firing, as the difference is still very small. In other words, we would want to consider a threshold for runtime increases to ensure we don’t get false-alarms, such as only considering jobs that take 5 minutes or more to execute.
例如,我们可以创建一条规则,规定:“任何运行时间比平均时间长50%的工作都应标记为长时间运行”。 如果一项工作通常需要500毫秒的时间来执行,而一天突然要花1秒钟,那么我们可能不希望触发警报,因为差异仍然很小。 换句话说,我们希望考虑运行时间增加的阈值,以确保不会收到错误警报,例如仅考虑需要花费5分钟或更长时间才能执行的作业。
Earlier, we wrote a script that would populate fact_daily_job_runtime_metrics, which provides us with a table of daily run stats, which can be used as a baseline to compare against. Since that table includes average values and counts, we can compute averages over any time span (weeks, months, etc…). We then can look at all job runs for today, and report on any that are taking too long to run:
之前,我们编写了一个脚本,该脚本将填充fact_daily_job_runtime_metrics ,该脚本为我们提供了每日运行统计信息表,可用作比较的基准。 由于该表包括平均值和计数,因此我们可以计算任何时间范围(周,月等)的平均值。 然后,我们可以查看今天的所有作业运行情况,并报告所有花费太长时间才能运行的作业:
WITH CTE_AVERAGE_JOB_RUNTIME AS (SELECTdim_sql_agent_job.Sql_Agent_Job_Name,dim_sql_agent_job.Sql_Agent_Job_Id,SUM(fact_daily_job_runtime_metrics.Job_Run_Count) AS Job_Run_Count,SUM(fact_daily_job_runtime_metrics.Job_Run_Time_Average * fact_daily_job_runtime_metrics.Job_Run_Count) / SUM(fact_daily_job_runtime_metrics.Job_Run_Count) AS Job_Run_Time_AverageFROM dbo.fact_daily_job_runtime_metricsINNER JOIN dbo.dim_sql_agent_jobON fact_daily_job_runtime_metrics.Sql_Agent_Job_Id = dim_sql_agent_job.Sql_Agent_Job_IdGROUP BY dim_sql_agent_job.Sql_Agent_Job_Id, dim_sql_agent_job.Sql_Agent_Job_Name)
SELECTCTE_AVERAGE_JOB_RUNTIME.Sql_Agent_Job_Name,fact_job_run_time.Job_Start_Datetime,fact_job_run_time.Job_End_Datetime,fact_job_run_time.Job_Duration_Seconds,fact_job_run_time.Job_Status,CTE_AVERAGE_JOB_RUNTIME.Job_Run_Count,
CTE_AVERAGE_JOB_RUNTIME.Job_Run_Time_Average
FROM dbo.fact_job_run_time
INNER JOIN CTE_AVERAGE_JOB_RUNTIME
ON CTE_AVERAGE_JOB_RUNTIME.Sql_Agent_Job_Id = fact_job_run_time.Sql_Agent_Job_Id
WHERE fact_job_run_time.Job_Start_Datetime >= CAST(CURRENT_TIMESTAMP AS DATE)
AND CTE_AVERAGE_JOB_RUNTIME.Job_Run_Time_Average > 15
AND fact_job_run_time.Job_Duration_Seconds > CTE_AVERAGE_JOB_RUNTIME.Job_Run_Time_Average * 2;
This script computes an average all-time for our duration data. If desired, we could constrain it to the past week, month, quarter, or whatever other time frame seems appropriate for “recent” data. We then compare today’s job runtimes to the average and if any individual job took more than double the average, a row is returned. We intentionally ignore any jobs that average 15 seconds or less, since they would likely cause unnecessary noise. This filter can also be adjusted to be more or less aggressive, to omit specific types of jobs, or otherwise clean up results such that no false alerts are generated. The results on my local server look like this:
该脚本为我们的工期数据计算平均时间。 如果需要,我们可以将其限制为过去的一周,一个月,一个季度或其他适合“最新”数据的时间范围。 然后,我们将今天的作业运行时间与平均值进行比较,如果任何单个作业花费的平均值超过平均值的两倍,则会返回一行。 我们故意忽略平均15秒或更短的任何作业,因为它们可能会引起不必要的噪音。 还可以将该过滤器调整为更具攻击性,以省略特定类型的作业,或者以其他方式清除结果,从而不会生成错误警报。 我的本地服务器上的结果如下所示:
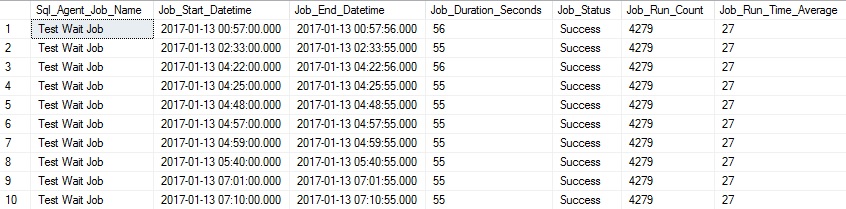
The results show each instance of a job that took longer than double the average run time (27 seconds in this case) and some pertinent details for it. This reporting can be sent out whenever needed, and could even be alerted on, if such a need arose. The metrics that determine a long running job are completely customizable. We can similarly filter for short running jobs—those that execute extremely quickly, and therefore may not be performing the usual amount of work. Adjusting this is as simple as changing the criteria of the WHERE clause above to:
结果显示,每个作业实例花费的时间超过平均运行时间的两倍(在这种情况下为27秒),并且还包含一些相关细节。 可以在需要时发送此报告,如果需要,甚至可以发出警报。 可以确定长时间运行的指标是完全可定制的。 我们可以类似地筛选短期运行的作业,这些作业执行得非常快,因此可能无法执行通常的工作量。 调整此方法就像将上面的WHERE子句的标准更改为:
- Run for a different time frame, multiple days, a fraction of a day, etc… 运行不同的时间范围,数天,一小部分时间等...
- Set a minimum or maximum threshold to check. 设置一个最小或最大阈值进行检查。
- Eliminate edge cases, such as jobs that are supposed to be very quick. 消除边缘情况,例如本应非常快的工作。
- Create more liberal boundaries for jobs that are known to be erratic. Statistics such as standard deviation can be useful in better gauging how inconsistent results are, in order to avoid hard-coding job details. 为已知不稳定的工作创建更多自由边界。 诸如标准偏差之类的统计信息可用于更好地衡量结果的不一致程度,从而避免对工作细节进行硬编码。
性能 (Performance)
With queries that are full of aggregation, common table expressions, table scans, and tiered queries, it is only natural to inquire about performance.
对于充满聚合,通用表表达式,表扫描和分层查询的查询,自然而然地询问性能。
In general, the processes that write this data are relatively speedy and will do what they need to quickly and without introducing any latency or resource drain on the system they are run. The reporting queries generally rely on table scans and have the potential to get slow. While not problematic, this is the primary reason that we separate our reporting data into new, customized tables and generate further reporting tables as we determine a need for more metrics. For example, we create dbo.fact_daily_job_runtime_metrics and store daily averages in this table, rather than run our aggregations directly against our more granular data, or against the MSDB system views.
通常,写入此数据的进程相对较快,将快速执行所需的操作,而不会在运行的系统上引入任何延迟或资源消耗。 报告查询通常依赖于表扫描,并且可能变慢。 尽管没有问题,但这是我们将报告数据分为新的自定义表并在确定需要更多指标时生成进一步报告表的主要原因。 例如,我们创建dbo.fact_daily_job_runtime_metrics并将每日平均值存储在该表中,而不是直接针对更细粒度的数据或针对MSDB系统视图运行聚合。
This provides us with more control, and the ability to design and structure the metrics tables to meet our custom needs. Include only the columns we need, with supporting indexes, and reports that are helpful. Any extraneous data in MSDB can be left out, and we only need to maintain as much data as we wish. Oftentimes, the granular data we store in fact_job_run_time and fact_step_job_run_time can be aggregated into more compact tables, such as the daily runtime metrics table referenced above. Once this data is crunched for the day, we need only keep it for a short while and then delete it. For some use-cases, a week or two may be all that is necessary to keep. If all we care about are metrics and will never review the detail data, then a single day of retention may be sufficient.
这为我们提供了更多的控制权,并提供了设计和构建指标表以满足我们的自定义需求的能力。 仅包括我们需要的列,支持索引和有用的报告。 MSDB中的任何无关数据都可以被忽略,我们只需要维护所需的数据就可以了。 通常,我们存储在fact_job_run_time和fact_step_job_run_time中的粒度数据可以聚合到更紧凑的表中,例如上面引用的每日运行时指标表。 当天处理完这些数据后,我们只需要保留一小会儿,然后将其删除即可。 对于某些用例,可能需要保留一两个星期。 如果我们只关心指标,并且永远不会查看详细数据,那么保留一天就足够了。
By controlling the data size and maintaining only the most useful metrics and relevant data, we can ensure that our reports run quickly. Even the Job duration/islands analysis, comprised of 3 cascading CTEs, can be fast, so long as the underlying data is kept simple and streamlined. Consider moving data to temporary tables when crunching more complex metrics, instead repeatedly accessing a large fact table.
通过控制数据大小并仅保留最有用的指标和相关数据,我们可以确保报告能够快速运行。 即使由3个级联CTE组成的Job Duration / island分析也可以快速进行,只要基本数据保持简单且精简即可。 在处理更复杂的指标时,考虑将数据移至临时表,而不是重复访问大型事实表。
In no examples here was performance a significant concern, but knowing how to deal with large reporting tables effectively can help in keeping things moving along efficiently. We do not want to suffer the irony of a reporting job that monitors job performance and becomes the resource hog on our server
sql server作业_SQL Server作业性能–报告相关推荐
- sql server 跟踪_SQL Server作业性能–跟踪
sql server 跟踪 描述 (Description) Keeping track of our SQL Server Agent jobs is a very effective way to ...
- sql server 面试_SQL Server审核面试问题
sql server 面试 In this article, we will discuss a number of common and important SQL Server Audit que ...
- sql server 快照_SQL Server复制:配置快照和事务复制
sql server 快照 In this article of the series on SQL Server replication, we will explore ways to confi ...
- sql server 跟踪_SQL Server跟踪标志指南; 从-1到840
sql server 跟踪 SQL Server trace flags are configuration handles that can be used to enable or disable ...
- sql server 群集_SQL Server群集索引概述
sql server 群集 This article targets the beginners and gives an introduction of the clustered index in ...
- sql tempdb清理_SQL Server 2019内存优化的TempDB元数据
sql tempdb清理 In this article, I will walk you through the new feature in SQL Server 2019, memory-opt ...
- 2008 r2 server sql 中文版补丁_SQL Server 2008 SP4 补丁
SQL Server 2008 SP4 补丁对于客户而言,Microsoft SQL Server 2008 Service Pack 4 中的几个关键改进如下所示: 改进了从 SQL Server ...
- sql数据透视_SQL Server中的数据科学:取消数据透视
sql数据透视 In this article, in the series, we'll discuss understanding and preparing data by using SQL ...
- sql server 别名_SQL Server别名概述
sql server 别名 This article gives an overview of SQL Server Alias and its usage for connecting with S ...
最新文章
- linux的QQ浏览器里微信,移动端微信内置浏览器(或QQ浏览器)无法识别onclick事件的问题解决...
- docker安装clickhouse_Docker中安装ClickHouse,单机、集群、分布式
- 华为ipd产品开发流程_华为集成产品开发(IPD)流程的解读
- 0点mysql_【转载】MySQL查询当天0点,昨天时间
- 跳槽必看:产品经理面试试题汇总
- 【ubuntu】ubuntu下用make编译程序报错找不到openssl/conf.h
- zabbix mysql设置中文乱码_解决zabbix监控因php问题导致图形界面中文乱码方法
- antd table设置表格一个单元格的字体颜色_alireacttable:高性能 React 表格组件
- IE浏览器下如何低延迟播放RTSP或RTMP流
- 95-10-170-启动-KafkaRequestHandlerPool
- 基于JAVA+Swing+MYSQL的超市管理系统
- java 元组_简单实现 Java 的 Tuple 元组数据类型
- 通过 Kubeadm联网部署K8S
- L1-1 PTA使我精神焕发 (5 分)
- Python 爬取表情包并保存在数据库中(以往的爬虫改进)
- 视频压缩编码和音频压缩编码的基本原理
- spider_study 1
- 岭南的一艘 “海盗船” 出海时扬起了单机游戏的帆
- 给大家分享两套WordPress收费主题
- java动态心形程序_java swing实现动态心形图案的代码下载